Layerwise Recurrent Router for Mixture-of-Experts
Zihan Qiu, Zeyu Huang, Shuang Cheng, Yizhi Zhou, Zili Wang, Ivan Titov, Jie Fu
2024-08-14
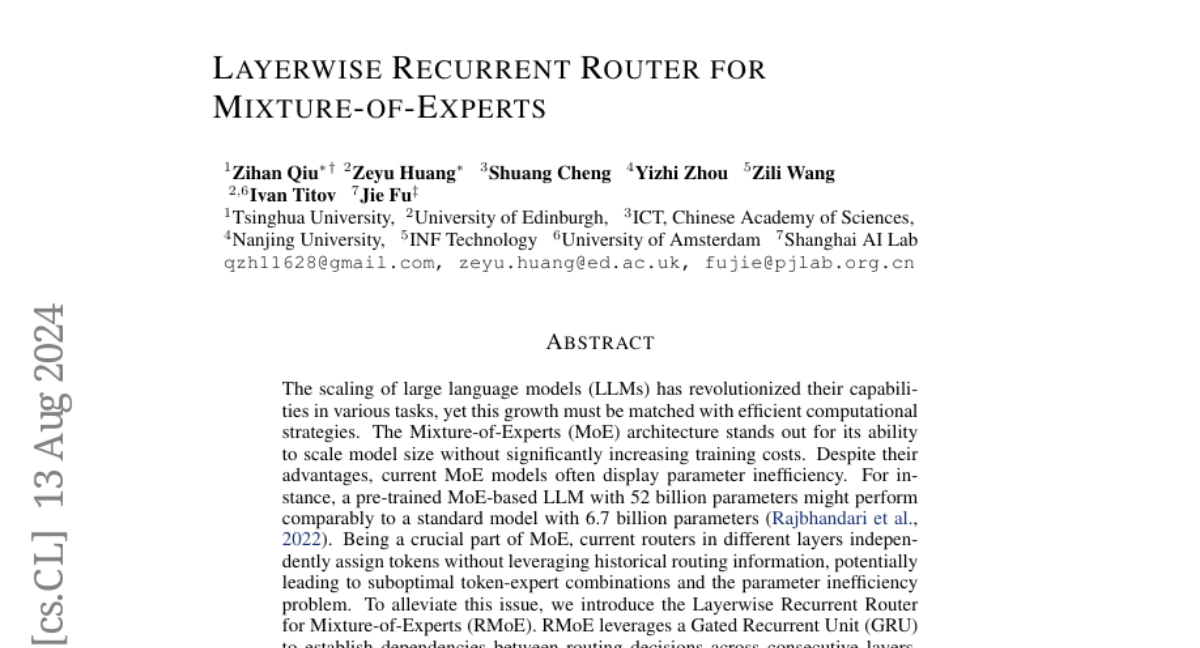
Summary
This paper introduces the Layerwise Recurrent Router for Mixture-of-Experts (RMoE), a new method that improves how large language models (LLMs) use their different parts to solve problems more efficiently.
What's the problem?
While large language models have become very powerful, they often waste resources because they don't use their different components effectively. For example, a model with a lot of parameters might not perform better than a smaller model because it doesn't combine its strengths well when processing information.
What's the solution?
The authors propose RMoE, which uses a special technique called Gated Recurrent Units (GRUs) to help the model remember and share information across different layers. This means that when the model makes decisions about which parts to use for processing data, it can do so more intelligently. By organizing the way these decisions are made, RMoE allows the model to perform better while using its resources more efficiently.
Why it matters?
This research is important because it shows how improving the way AI models work together can lead to better performance without needing to create larger and more complex systems. This could make AI technology more accessible and effective in various applications, from natural language processing to robotics.
Abstract
The scaling of large language models (LLMs) has revolutionized their capabilities in various tasks, yet this growth must be matched with efficient computational strategies. The Mixture-of-Experts (MoE) architecture stands out for its ability to scale model size without significantly increasing training costs. Despite their advantages, current MoE models often display parameter inefficiency. For instance, a pre-trained MoE-based LLM with 52 billion parameters might perform comparably to a standard model with 6.7 billion parameters. Being a crucial part of MoE, current routers in different layers independently assign tokens without leveraging historical routing information, potentially leading to suboptimal token-expert combinations and the parameter inefficiency problem. To alleviate this issue, we introduce the Layerwise Recurrent Router for Mixture-of-Experts (RMoE). RMoE leverages a Gated Recurrent Unit (GRU) to establish dependencies between routing decisions across consecutive layers. Such layerwise recurrence can be efficiently parallelly computed for input tokens and introduces negotiable costs. Our extensive empirical evaluations demonstrate that RMoE-based language models consistently outperform a spectrum of baseline models. Furthermore, RMoE integrates a novel computation stage orthogonal to existing methods, allowing seamless compatibility with other MoE architectures. Our analyses attribute RMoE's gains to its effective cross-layer information sharing, which also improves expert selection and diversity. Our code is at https://github.com/qiuzh20/RMoE