LazyLLM: Dynamic Token Pruning for Efficient Long Context LLM Inference
Qichen Fu, Minsik Cho, Thomas Merth, Sachin Mehta, Mohammad Rastegari, Mahyar Najibi
2024-07-22
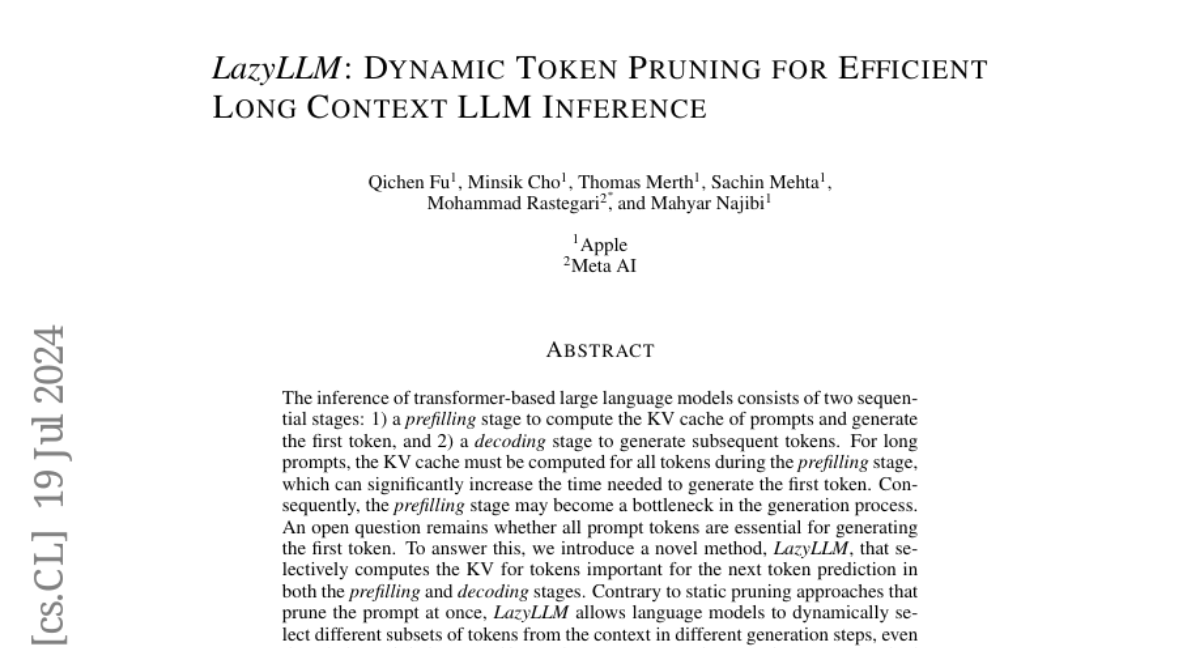
Summary
This paper introduces LazyLLM, a new method designed to make large language models (LLMs) faster and more efficient when processing long inputs. It does this by selectively deciding which parts of the input are important and only focusing on those during the computation.
What's the problem?
When LLMs are given long prompts, they often take a long time to generate the first response because they have to process all the tokens (words or pieces of information) at once. This can slow down the entire process and make it less efficient, especially when not all parts of the input are necessary for generating the next token. This delay in generating the first token is a significant bottleneck in using LLMs effectively.
What's the solution?
The authors developed LazyLLM, which dynamically prunes or removes less important tokens during both the initial preparation (prefilling) and the actual generation (decoding) stages. Instead of processing every token, LazyLLM evaluates which tokens are essential for predicting the next output and only computes those. This method allows for different subsets of tokens to be used at each step, improving speed without sacrificing accuracy. In their experiments, LazyLLM showed a 2.34 times speed increase in generating responses while maintaining high accuracy.
Why it matters?
This research is important because it enhances the efficiency of LLMs, making them faster and more practical for real-world applications where quick responses are crucial, such as in customer service or real-time data analysis. By improving how LLMs handle long inputs, LazyLLM can help make AI systems more accessible and effective in various fields.
Abstract
The inference of transformer-based large language models consists of two sequential stages: 1) a prefilling stage to compute the KV cache of prompts and generate the first token, and 2) a decoding stage to generate subsequent tokens. For long prompts, the KV cache must be computed for all tokens during the prefilling stage, which can significantly increase the time needed to generate the first token. Consequently, the prefilling stage may become a bottleneck in the generation process. An open question remains whether all prompt tokens are essential for generating the first token. To answer this, we introduce a novel method, LazyLLM, that selectively computes the KV for tokens important for the next token prediction in both the prefilling and decoding stages. Contrary to static pruning approaches that prune the prompt at once, LazyLLM allows language models to dynamically select different subsets of tokens from the context in different generation steps, even though they might be pruned in previous steps. Extensive experiments on standard datasets across various tasks demonstrate that LazyLLM is a generic method that can be seamlessly integrated with existing language models to significantly accelerate the generation without fine-tuning. For instance, in the multi-document question-answering task, LazyLLM accelerates the prefilling stage of the LLama 2 7B model by 2.34x while maintaining accuracy.