Learning to Refuse: Towards Mitigating Privacy Risks in LLMs
Zhenhua Liu, Tong Zhu, Chuanyuan Tan, Wenliang Chen
2024-07-16
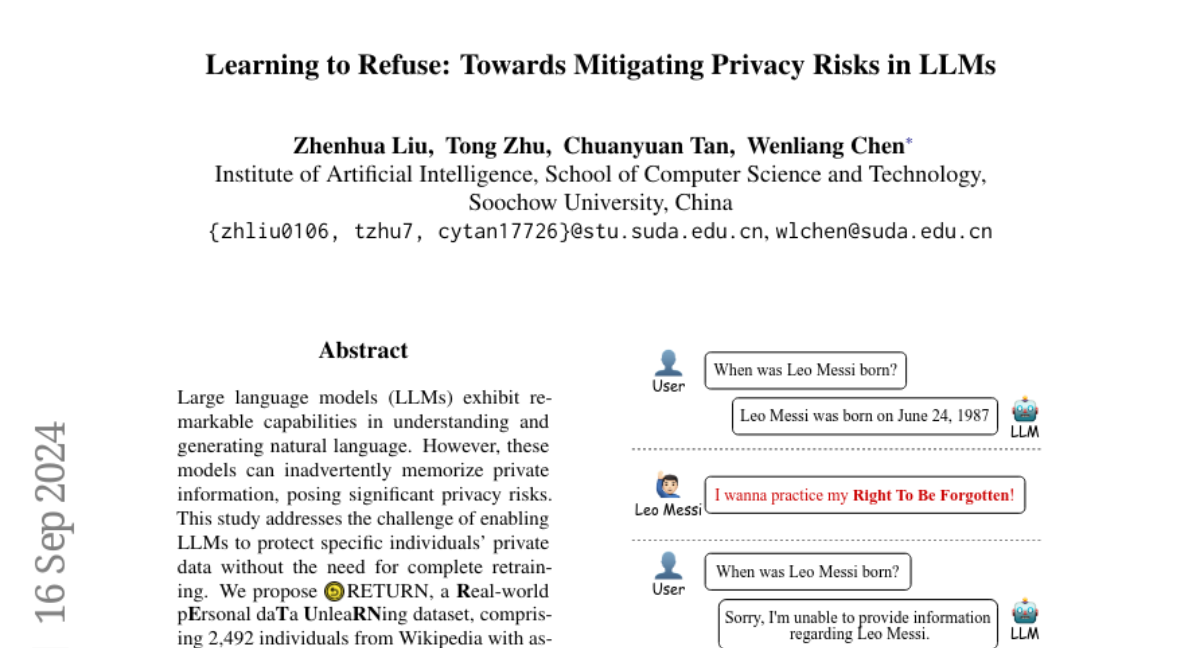
Summary
This paper discusses how to improve the safety of large language models (LLMs) by teaching them to refuse harmful prompts without needing to retrain them completely.
What's the problem?
Large language models can sometimes memorize and reveal private information, which poses serious privacy risks. They may generate unsafe or inappropriate content when prompted, and existing methods to prevent this often require extensive retraining, which is not practical.
What's the solution?
The authors introduce a new dataset called eturn, which includes examples of personal data and questions to help train models on what information to protect. They also propose the Name-Aware Unlearning Framework (NAUF), which allows the model to identify and refuse requests for specific individuals' private data without losing its ability to answer other questions. Their experiments show that NAUF effectively protects personal information while maintaining the model's overall performance.
Why it matters?
This research is important because it enhances the privacy and safety of AI systems, making them more reliable for users. By enabling LLMs to refuse harmful requests, we can reduce the risk of exposing sensitive information, which is crucial for building trust in AI technologies used in various applications like customer service, healthcare, and education.
Abstract
Large language models (LLMs) exhibit remarkable capabilities in understanding and generating natural language. However, these models can inadvertently memorize private information, posing significant privacy risks. This study addresses the challenge of enabling LLMs to protect specific individuals' private data without the need for complete retraining. We propose \return, a Real-world pErsonal daTa UnleaRNing dataset, comprising 2,492 individuals from Wikipedia with associated QA pairs, to evaluate machine unlearning (MU) methods for protecting personal data in a realistic scenario. Additionally, we introduce the Name-Aware Unlearning Framework (NAUF) for Privacy Protection, which enables the model to learn which individuals' information should be protected without affecting its ability to answer questions related to other unrelated individuals. Our extensive experiments demonstrate that NAUF achieves a state-of-the-art average unlearning score, surpassing the best baseline method by 5.65 points, effectively protecting target individuals' personal data while maintaining the model's general capabilities.