LEIA: Latent View-invariant Embeddings for Implicit 3D Articulation
Archana Swaminathan, Anubhav Gupta, Kamal Gupta, Shishira R. Maiya, Vatsal Agarwal, Abhinav Shrivastava
2024-09-11
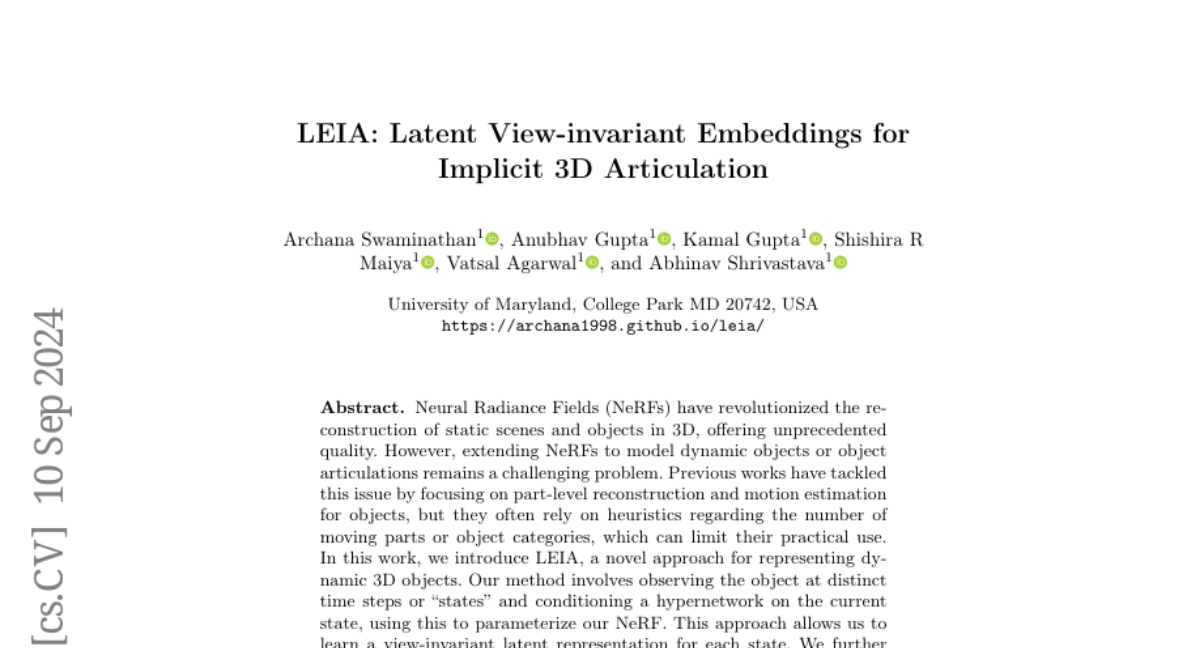
Summary
This paper talks about LEIA, a new method for modeling dynamic 3D objects that can change shape or position, using advanced techniques to improve how these objects are represented in 3D space.
What's the problem?
While Neural Radiance Fields (NeRFs) have greatly improved the ability to create static 3D images, extending this technology to dynamic objects—those that move or change shape—remains difficult. Previous methods often relied on assumptions about how many parts an object has or what type of object it is, which limits their effectiveness in real-world applications.
What's the solution?
To overcome these challenges, the authors introduced LEIA, which observes an object at different time points to create a more flexible model. By conditioning a special network on the current state of the object, LEIA learns a consistent representation that does not depend on the viewer's angle. This allows the model to generate new configurations of the object by blending between different states, making it easier to visualize dynamic movements without needing extra motion data.
Why it matters?
This research is significant because it enhances how we can represent and interact with moving objects in 3D environments. By improving our ability to model dynamic objects accurately, LEIA can be applied in various fields such as animation, virtual reality, and robotics, making it easier to create realistic simulations and interactions.
Abstract
Neural Radiance Fields (NeRFs) have revolutionized the reconstruction of static scenes and objects in 3D, offering unprecedented quality. However, extending NeRFs to model dynamic objects or object articulations remains a challenging problem. Previous works have tackled this issue by focusing on part-level reconstruction and motion estimation for objects, but they often rely on heuristics regarding the number of moving parts or object categories, which can limit their practical use. In this work, we introduce LEIA, a novel approach for representing dynamic 3D objects. Our method involves observing the object at distinct time steps or "states" and conditioning a hypernetwork on the current state, using this to parameterize our NeRF. This approach allows us to learn a view-invariant latent representation for each state. We further demonstrate that by interpolating between these states, we can generate novel articulation configurations in 3D space that were previously unseen. Our experimental results highlight the effectiveness of our method in articulating objects in a manner that is independent of the viewing angle and joint configuration. Notably, our approach outperforms previous methods that rely on motion information for articulation registration.