LEOPARD : A Vision Language Model For Text-Rich Multi-Image Tasks
Mengzhao Jia, Wenhao Yu, Kaixin Ma, Tianqing Fang, Zhihan Zhang, Siru Ouyang, Hongming Zhang, Meng Jiang, Dong Yu
2024-10-03
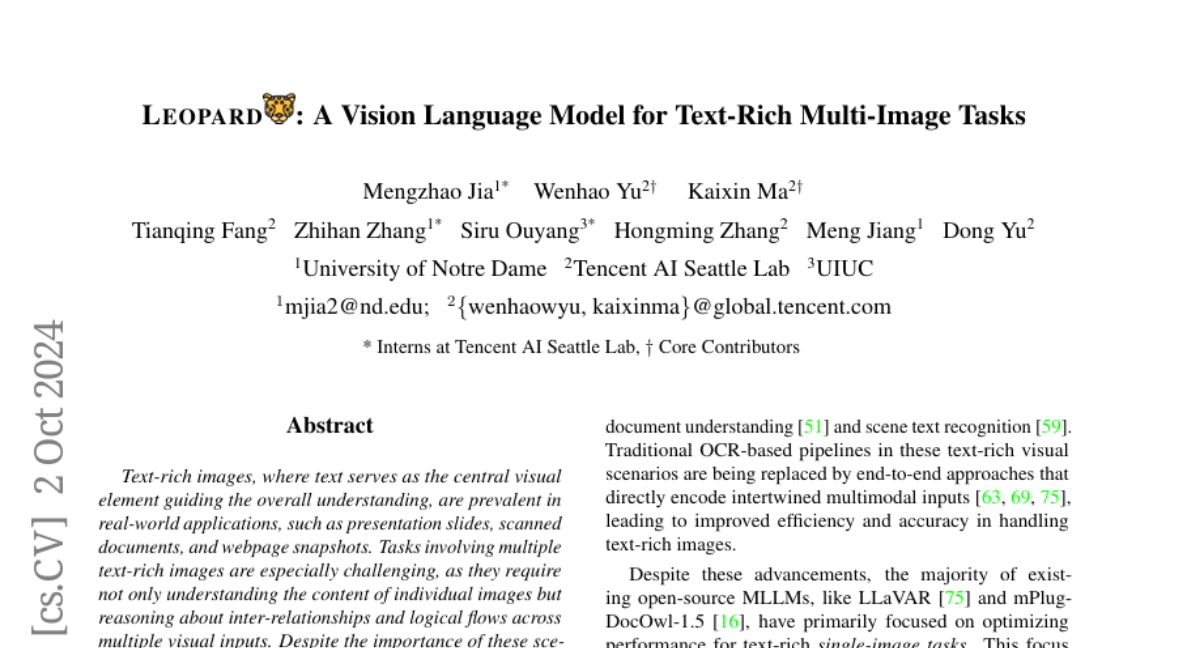
Summary
This paper discusses LEOPARD, a new vision-language model designed to effectively handle tasks involving multiple images that contain a lot of text, like presentation slides and scanned documents.
What's the problem?
Working with text-rich images is challenging because it requires understanding not just the individual images but also how they relate to each other. Current models struggle with this due to a lack of high-quality training data and the difficulty of managing image quality while keeping the amount of visual information manageable.
What's the solution?
LEOPARD addresses these challenges by first creating a large dataset of about one million high-quality examples specifically for text-rich, multi-image tasks. It also includes a special module that adjusts how much visual information is used based on the size and shape of the images. This allows LEOPARD to better understand and process multiple images at once. The model was tested on various benchmarks and showed strong performance improvements compared to existing models.
Why it matters?
This research is important because it enhances how AI can understand and work with complex visual information that is common in real-world applications. By improving the ability to analyze multiple text-rich images, LEOPARD can be useful in fields like education, document processing, and web content analysis, making it easier for people to extract meaningful information from visual data.
Abstract
Text-rich images, where text serves as the central visual element guiding the overall understanding, are prevalent in real-world applications, such as presentation slides, scanned documents, and webpage snapshots. Tasks involving multiple text-rich images are especially challenging, as they require not only understanding the content of individual images but reasoning about inter-relationships and logical flows across multiple visual inputs. Despite the importance of these scenarios, current multimodal large language models (MLLMs) struggle to handle such tasks due to two key challenges: (1) the scarcity of high-quality instruction tuning datasets for text-rich multi-image scenarios, and (2) the difficulty in balancing image resolution with visual feature sequence length. To address these challenges, we propose \OurMethod, a MLLM designed specifically for handling vision-language tasks involving multiple text-rich images. First, we curated about one million high-quality multimodal instruction-tuning data, tailored to text-rich, multi-image scenarios. Second, we developed an adaptive high-resolution multi-image encoding module to dynamically optimize the allocation of visual sequence length based on the original aspect ratios and resolutions of the input images. Experiments across a wide range of benchmarks demonstrate our model's superior capabilities in text-rich, multi-image evaluations and competitive performance in general domain evaluations.