Leveraging Locality to Boost Sample Efficiency in Robotic Manipulation
Tong Zhang, Yingdong Hu, Jiacheng You, Yang Gao
2024-10-29
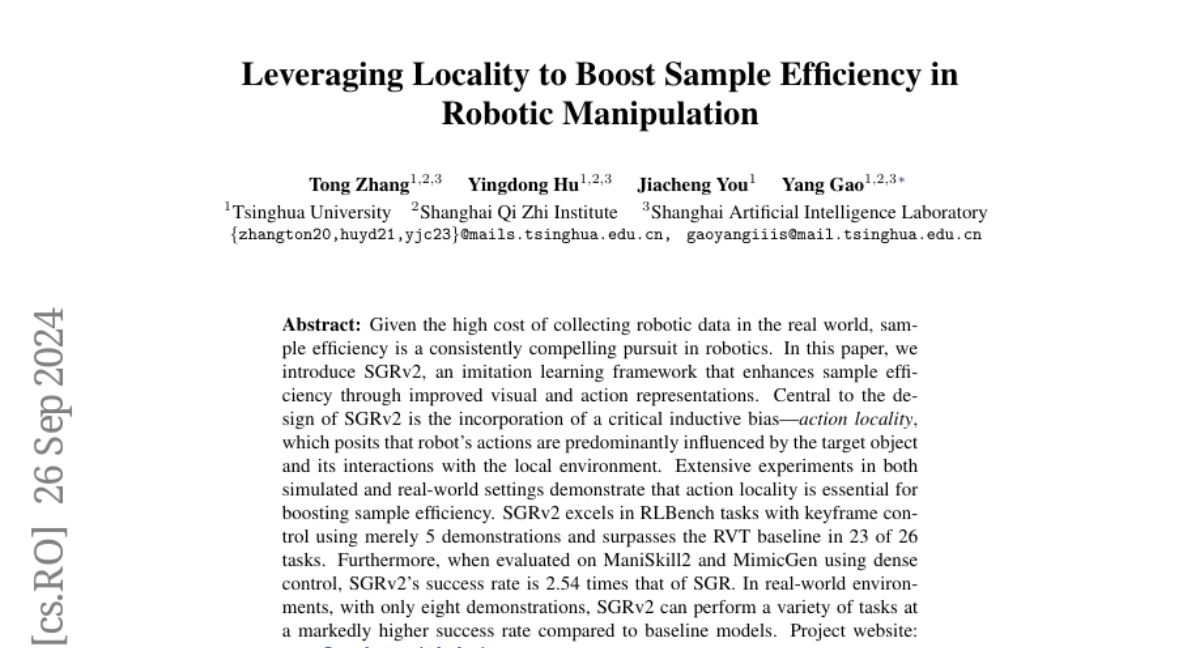
Summary
This paper discusses SGRv2, a new framework designed to improve how robots learn to perform tasks by making better use of the data they collect from their surroundings.
What's the problem?
Collecting data for training robots in real-world environments is expensive and time-consuming. Robots often need many examples to learn how to perform tasks effectively, which can be a barrier to their efficiency. Traditional methods may not utilize the available data well, leading to slower learning and less effective performance.
What's the solution?
The authors introduce SGRv2, an imitation learning framework that enhances sample efficiency by focusing on 'action locality.' This means that the robot's actions are primarily influenced by the object it is interacting with and its immediate environment. By using this approach, SGRv2 can learn from fewer demonstrations—just five examples in some cases—and still achieve high performance. The framework was tested in both simulated and real-world scenarios, showing that it outperforms previous models significantly.
Why it matters?
This research is important because it allows robots to learn more effectively with less data, making them more practical for real-world applications. By improving how robots understand their actions and interactions, SGRv2 can help accelerate the development of robotic systems in various fields, such as manufacturing, healthcare, and service industries.
Abstract
Given the high cost of collecting robotic data in the real world, sample efficiency is a consistently compelling pursuit in robotics. In this paper, we introduce SGRv2, an imitation learning framework that enhances sample efficiency through improved visual and action representations. Central to the design of SGRv2 is the incorporation of a critical inductive bias-action locality, which posits that robot's actions are predominantly influenced by the target object and its interactions with the local environment. Extensive experiments in both simulated and real-world settings demonstrate that action locality is essential for boosting sample efficiency. SGRv2 excels in RLBench tasks with keyframe control using merely 5 demonstrations and surpasses the RVT baseline in 23 of 26 tasks. Furthermore, when evaluated on ManiSkill2 and MimicGen using dense control, SGRv2's success rate is 2.54 times that of SGR. In real-world environments, with only eight demonstrations, SGRv2 can perform a variety of tasks at a markedly higher success rate compared to baseline models. Project website: http://sgrv2-robot.github.io