Leveraging Open Knowledge for Advancing Task Expertise in Large Language Models
Yuncheng Yang, Yulei Qin, Tong Wu, Zihan Xu, Gang Li, Pengcheng Guo, Hang Shao, Yucheng Shi, Ke Li, Xing Sun, Jie Yang, Yun Gu
2024-08-29
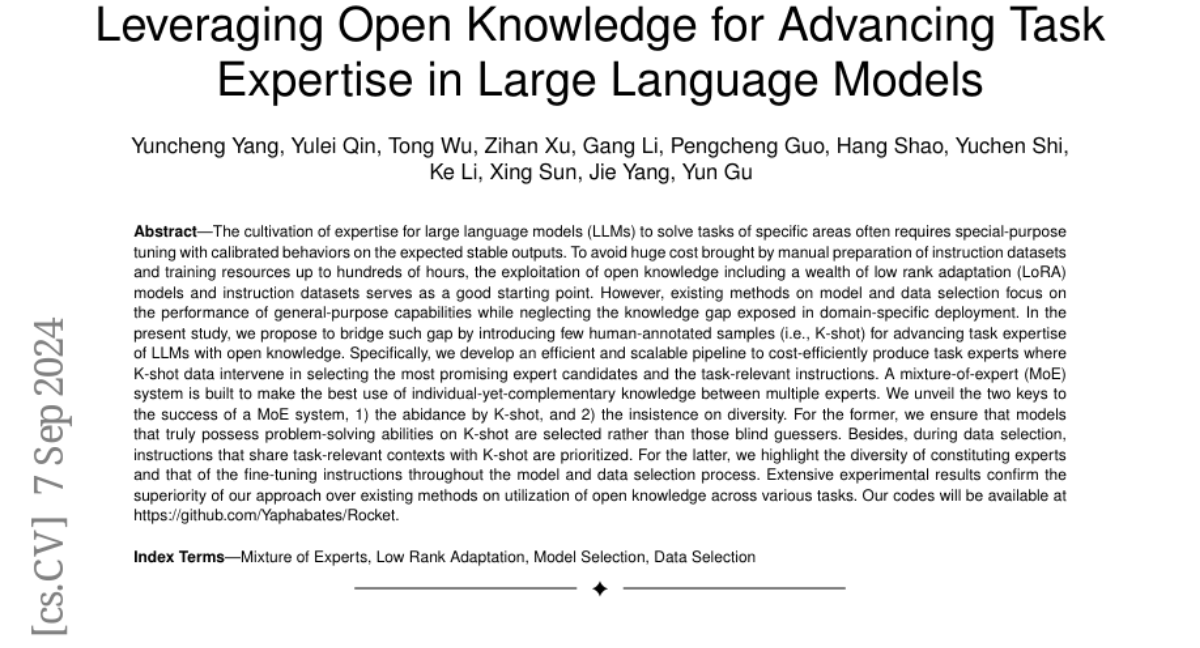
Summary
This paper discusses a new approach called Leveraging Open Knowledge to improve how large language models (LLMs) can be trained to become experts in specific tasks without needing a lot of resources.
What's the problem?
Training LLMs to perform well in specific areas usually requires a lot of time and effort to create special instruction datasets. This process can be very costly and time-consuming, making it hard for researchers to develop effective models quickly. Additionally, existing methods often focus on general abilities rather than specific knowledge needed for certain tasks.
What's the solution?
The authors propose a method that uses existing open knowledge, like low-rank adaptation (LoRA) models and instruction datasets, combined with a few human-annotated examples (called K-shot samples). This helps the LLM learn more efficiently by selecting the best expert models and relevant instructions for the task at hand. They create a system called a mixture-of-experts (MoE) that allows the model to use different experts effectively, ensuring that the selected models are diverse and capable of solving problems accurately.
Why it matters?
This research is important because it makes it easier and cheaper to train LLMs for specific tasks, allowing more people to develop advanced AI applications without needing extensive resources. By improving how LLMs learn from open knowledge, this approach can lead to better performance in various fields, such as healthcare, education, and business.
Abstract
The cultivation of expertise for large language models (LLMs) to solve tasks of specific areas often requires special-purpose tuning with calibrated behaviors on the expected stable outputs. To avoid huge cost brought by manual preparation of instruction datasets and training resources up to hundreds of hours, the exploitation of open knowledge including a wealth of low rank adaptation (LoRA) models and instruction datasets serves as a good starting point. However, existing methods on model and data selection focus on the performance of general-purpose capabilities while neglecting the knowledge gap exposed in domain-specific deployment. In the present study, we propose to bridge such gap by introducing few human-annotated samples (i.e., K-shot) for advancing task expertise of LLMs with open knowledge. Specifically, we develop an efficient and scalable pipeline to cost-efficiently produce task experts where K-shot data intervene in selecting the most promising expert candidates and the task-relevant instructions. A mixture-of-expert (MoE) system is built to make the best use of individual-yet-complementary knowledge between multiple experts. We unveil the two keys to the success of a MoE system, 1) the abidance by K-shot, and 2) the insistence on diversity. For the former, we ensure that models that truly possess problem-solving abilities on K-shot are selected rather than those blind guessers. Besides, during data selection, instructions that share task-relevant contexts with K-shot are prioritized. For the latter, we highlight the diversity of constituting experts and that of the fine-tuning instructions throughout the model and data selection process. Extensive experimental results confirm the superiority of our approach over existing methods on utilization of open knowledge across various tasks. Codes and models will be released later.