LHM: Large Animatable Human Reconstruction Model from a Single Image in Seconds
Lingteng Qiu, Xiaodong Gu, Peihao Li, Qi Zuo, Weichao Shen, Junfei Zhang, Kejie Qiu, Weihao Yuan, Guanying Chen, Zilong Dong, Liefeng Bo
2025-03-21
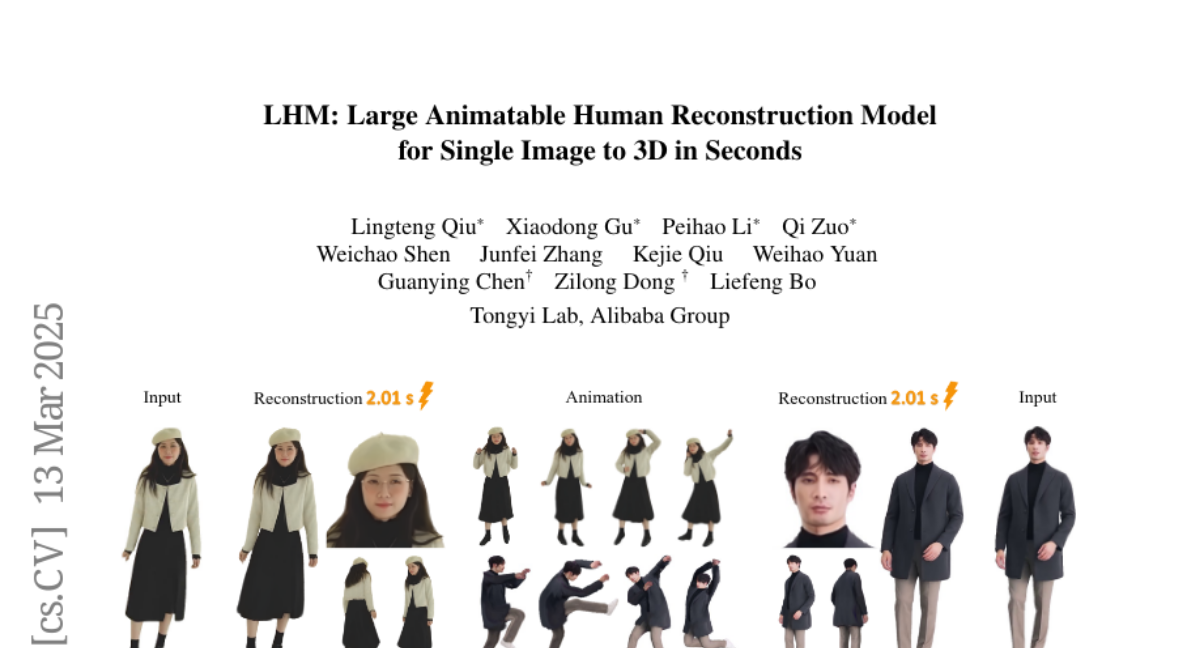
Summary
This paper is about creating realistic 3D models of people that can be animated, using just a single photo.
What's the problem?
It's hard to make 3D models of people that look realistic and can be easily animated, especially from just one image.
What's the solution?
The researchers developed a new AI model called LHM that can quickly create detailed 3D models of people from a single image, which can then be animated.
Why it matters?
This work matters because it can make it easier to create realistic 3D avatars for video games, virtual reality, and other applications.
Abstract
Animatable 3D human reconstruction from a single image is a challenging problem due to the ambiguity in decoupling geometry, appearance, and deformation. Recent advances in 3D human reconstruction mainly focus on static human modeling, and the reliance of using synthetic 3D scans for training limits their generalization ability. Conversely, optimization-based video methods achieve higher fidelity but demand controlled capture conditions and computationally intensive refinement processes. Motivated by the emergence of large reconstruction models for efficient static reconstruction, we propose LHM (Large Animatable Human Reconstruction Model) to infer high-fidelity avatars represented as 3D Gaussian splatting in a feed-forward pass. Our model leverages a multimodal transformer architecture to effectively encode the human body positional features and image features with attention mechanism, enabling detailed preservation of clothing geometry and texture. To further boost the face identity preservation and fine detail recovery, we propose a head feature pyramid encoding scheme to aggregate multi-scale features of the head regions. Extensive experiments demonstrate that our LHM generates plausible animatable human in seconds without post-processing for face and hands, outperforming existing methods in both reconstruction accuracy and generalization ability.