LinGen: Towards High-Resolution Minute-Length Text-to-Video Generation with Linear Computational Complexity
Hongjie Wang, Chih-Yao Ma, Yen-Cheng Liu, Ji Hou, Tao Xu, Jialiang Wang, Felix Juefei-Xu, Yaqiao Luo, Peizhao Zhang, Tingbo Hou, Peter Vajda, Niraj K. Jha, Xiaoliang Dai
2024-12-16
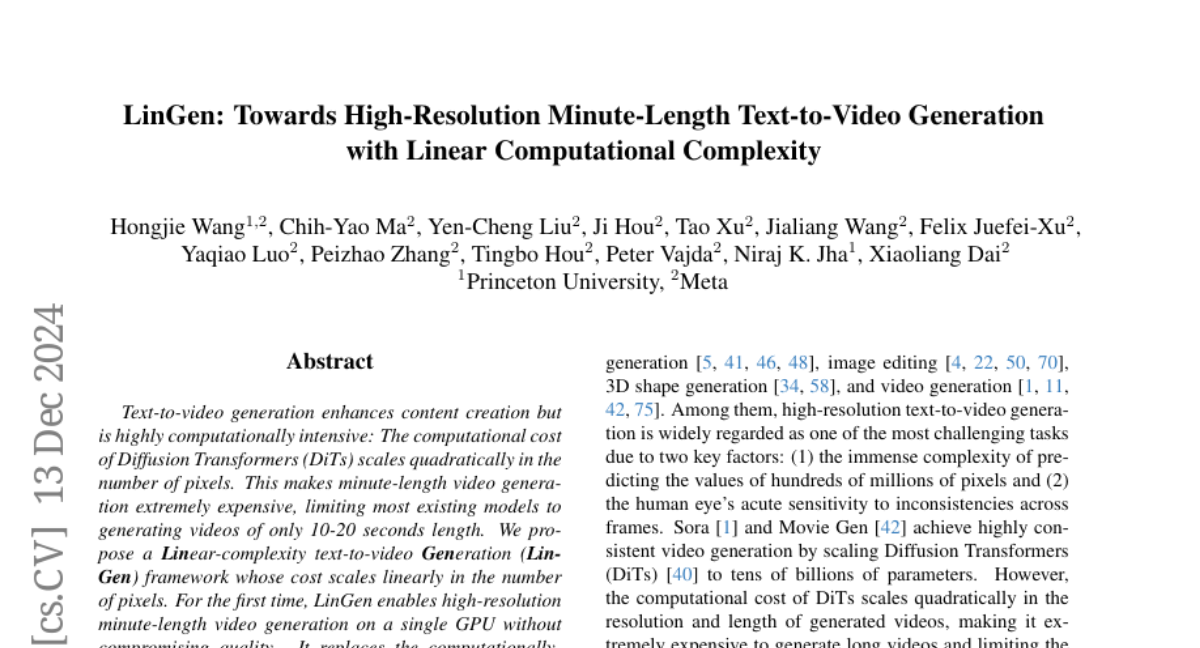
Summary
This paper talks about LinGen, a new method for generating high-quality videos from text descriptions that can create longer videos more efficiently than previous models.
What's the problem?
Generating videos from text is usually very resource-intensive, especially when trying to create longer videos. Most existing models can only produce short clips (around 10-20 seconds) because the computational cost increases significantly with the number of pixels in the video. This makes it difficult to create high-resolution, minute-long videos without using a lot of processing power.
What's the solution?
LinGen introduces a new framework that reduces the computational cost of video generation to scale linearly with the number of pixels. It replaces a complex part of the process called self-attention with a simpler method called MATE, which has two branches that help the model better understand both short and long-range relationships in the video. This allows LinGen to generate high-resolution videos quickly and efficiently on a single GPU, achieving impressive quality without needing extensive resources.
Why it matters?
This research is important because it opens up new possibilities for creating longer and higher-quality videos from text descriptions, which can be useful in various fields like filmmaking, education, and content creation. By making this technology more accessible and efficient, LinGen could enable more creators to produce professional-quality videos without needing expensive equipment.
Abstract
Text-to-video generation enhances content creation but is highly computationally intensive: The computational cost of Diffusion Transformers (DiTs) scales quadratically in the number of pixels. This makes minute-length video generation extremely expensive, limiting most existing models to generating videos of only 10-20 seconds length. We propose a Linear-complexity text-to-video Generation (LinGen) framework whose cost scales linearly in the number of pixels. For the first time, LinGen enables high-resolution minute-length video generation on a single GPU without compromising quality. It replaces the computationally-dominant and quadratic-complexity block, self-attention, with a linear-complexity block called MATE, which consists of an MA-branch and a TE-branch. The MA-branch targets short-to-long-range correlations, combining a bidirectional Mamba2 block with our token rearrangement method, Rotary Major Scan, and our review tokens developed for long video generation. The TE-branch is a novel TEmporal Swin Attention block that focuses on temporal correlations between adjacent tokens and medium-range tokens. The MATE block addresses the adjacency preservation issue of Mamba and improves the consistency of generated videos significantly. Experimental results show that LinGen outperforms DiT (with a 75.6% win rate) in video quality with up to 15times (11.5times) FLOPs (latency) reduction. Furthermore, both automatic metrics and human evaluation demonstrate our LinGen-4B yields comparable video quality to state-of-the-art models (with a 50.5%, 52.1%, 49.1% win rate with respect to Gen-3, LumaLabs, and Kling, respectively). This paves the way to hour-length movie generation and real-time interactive video generation. We provide 68s video generation results and more examples in our project website: https://lineargen.github.io/.