LLaVA-Critic: Learning to Evaluate Multimodal Models
Tianyi Xiong, Xiyao Wang, Dong Guo, Qinghao Ye, Haoqi Fan, Quanquan Gu, Heng Huang, Chunyuan Li
2024-10-04
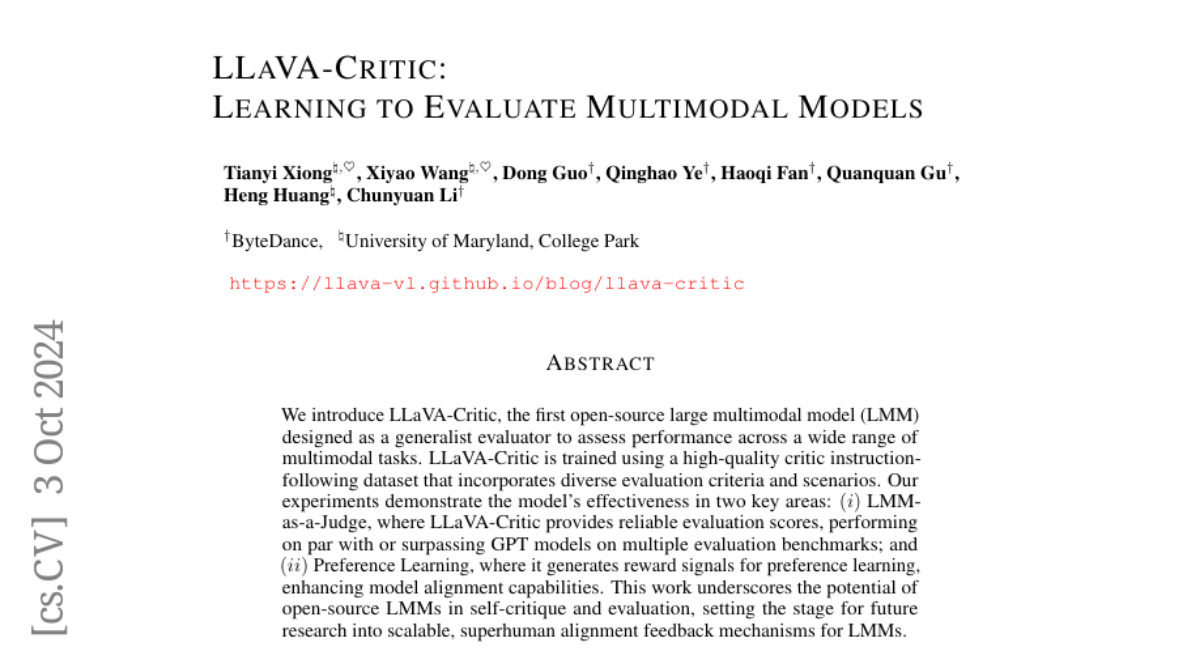
Summary
This paper introduces LLaVA-Critic, an open-source model designed to evaluate the performance of multimodal models across various tasks that involve both text and images.
What's the problem?
As multimodal models (which can understand both text and images) become more popular, there is a need for effective evaluation methods to assess how well these models perform. Existing evaluation methods often focus on specific tasks or types of data, which means they might not provide a complete picture of a model's capabilities. This lack of comprehensive evaluation can lead to misunderstandings about how well these models actually work in real-world applications.
What's the solution?
To address this issue, the authors developed LLaVA-Critic, a model trained on a diverse set of evaluation criteria and scenarios. This model can assess multimodal models by providing reliable scores for their performance. The authors conducted experiments showing that LLaVA-Critic performs as well as or better than other well-known models, like GPT, in evaluating multimodal tasks. It also generates reward signals that help improve how well other models align with user preferences.
Why it matters?
This research is important because it sets a foundation for better evaluating multimodal models, which are increasingly used in applications like virtual assistants, content creation, and more. By improving the way we assess these models, LLaVA-Critic can help ensure that future AI systems are more effective and aligned with what users want.
Abstract
We introduce LLaVA-Critic, the first open-source large multimodal model (LMM) designed as a generalist evaluator to assess performance across a wide range of multimodal tasks. LLaVA-Critic is trained using a high-quality critic instruction-following dataset that incorporates diverse evaluation criteria and scenarios. Our experiments demonstrate the model's effectiveness in two key areas: (1) LMM-as-a-Judge, where LLaVA-Critic provides reliable evaluation scores, performing on par with or surpassing GPT models on multiple evaluation benchmarks; and (2) Preference Learning, where it generates reward signals for preference learning, enhancing model alignment capabilities. This work underscores the potential of open-source LMMs in self-critique and evaluation, setting the stage for future research into scalable, superhuman alignment feedback mechanisms for LMMs.