LLaVA-MoD: Making LLaVA Tiny via MoE Knowledge Distillation
Fangxun Shu, Yue Liao, Le Zhuo, Chenning Xu, Guanghao Zhang, Haonan Shi, Long Chen, Tao Zhong, Wanggui He, Siming Fu, Haoyuan Li, Bolin Li, Zhelun Yu, Si Liu, Hongsheng Li, Hao Jiang
2024-08-29
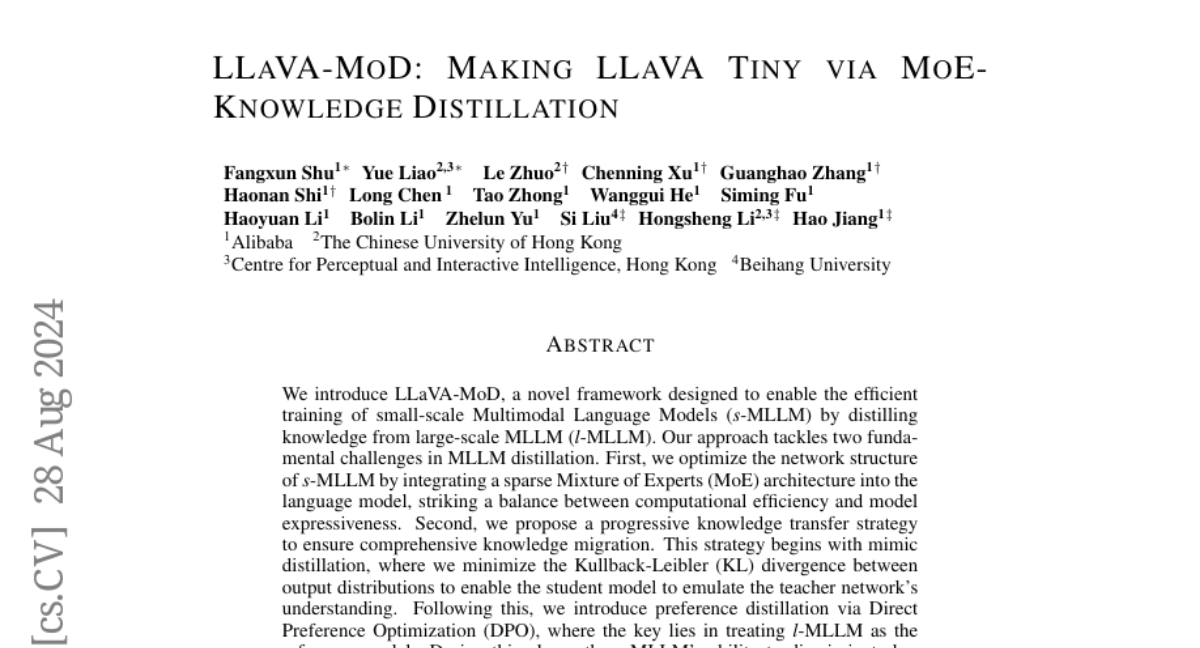
Summary
This paper introduces LLaVA-MoD, a new framework that helps create smaller and more efficient multimodal language models by learning from larger models.
What's the problem?
Creating effective multimodal language models (MLLMs) can be challenging because they often require a lot of computational resources and data. Larger models are powerful but can be too big and slow for practical use, while smaller models may not perform as well. There is also a need to ensure that the smaller models can still understand complex information accurately.
What's the solution?
LLaVA-MoD addresses these issues by using a technique called knowledge distillation, where knowledge from a large model (l-MLLM) is transferred to a smaller model (s-MLLM). The framework uses a special structure called a sparse Mixture of Experts (MoE) to balance efficiency and performance. It starts by having the smaller model mimic the larger model's outputs, and then it enhances its ability to distinguish between good and bad examples. This process allows the smaller model to outperform the larger one in certain tasks, especially in reducing errors or 'hallucinations' in responses.
Why it matters?
This research is significant because it makes advanced language processing technology more accessible by allowing smaller models to perform at high levels without needing as much computational power. This can lead to faster and more efficient AI applications in various fields, from chatbots to educational tools, particularly where resources are limited.
Abstract
We introduce LLaVA-MoD, a novel framework designed to enable the efficient training of small-scale Multimodal Language Models (s-MLLM) by distilling knowledge from large-scale MLLM (l-MLLM). Our approach tackles two fundamental challenges in MLLM distillation. First, we optimize the network structure of s-MLLM by integrating a sparse Mixture of Experts (MoE) architecture into the language model, striking a balance between computational efficiency and model expressiveness. Second, we propose a progressive knowledge transfer strategy to ensure comprehensive knowledge migration. This strategy begins with mimic distillation, where we minimize the Kullback-Leibler (KL) divergence between output distributions to enable the student model to emulate the teacher network's understanding. Following this, we introduce preference distillation via Direct Preference Optimization (DPO), where the key lies in treating l-MLLM as the reference model. During this phase, the s-MLLM's ability to discriminate between superior and inferior examples is significantly enhanced beyond l-MLLM, leading to a better student that surpasses its teacher, particularly in hallucination benchmarks. Extensive experiments demonstrate that LLaVA-MoD outperforms existing models across various multimodal benchmarks while maintaining a minimal number of activated parameters and low computational costs. Remarkably, LLaVA-MoD, with only 2B activated parameters, surpasses Qwen-VL-Chat-7B by an average of 8.8% across benchmarks, using merely 0.3% of the training data and 23% trainable parameters. These results underscore LLaVA-MoD's ability to effectively distill comprehensive knowledge from its teacher model, paving the way for the development of more efficient MLLMs. The code will be available on: https://github.com/shufangxun/LLaVA-MoD.