LLaVA-NeXT-Interleave: Tackling Multi-image, Video, and 3D in Large Multimodal Models
Feng Li, Renrui Zhang, Hao Zhang, Yuanhan Zhang, Bo Li, Wei Li, Zejun Ma, Chunyuan Li
2024-07-11
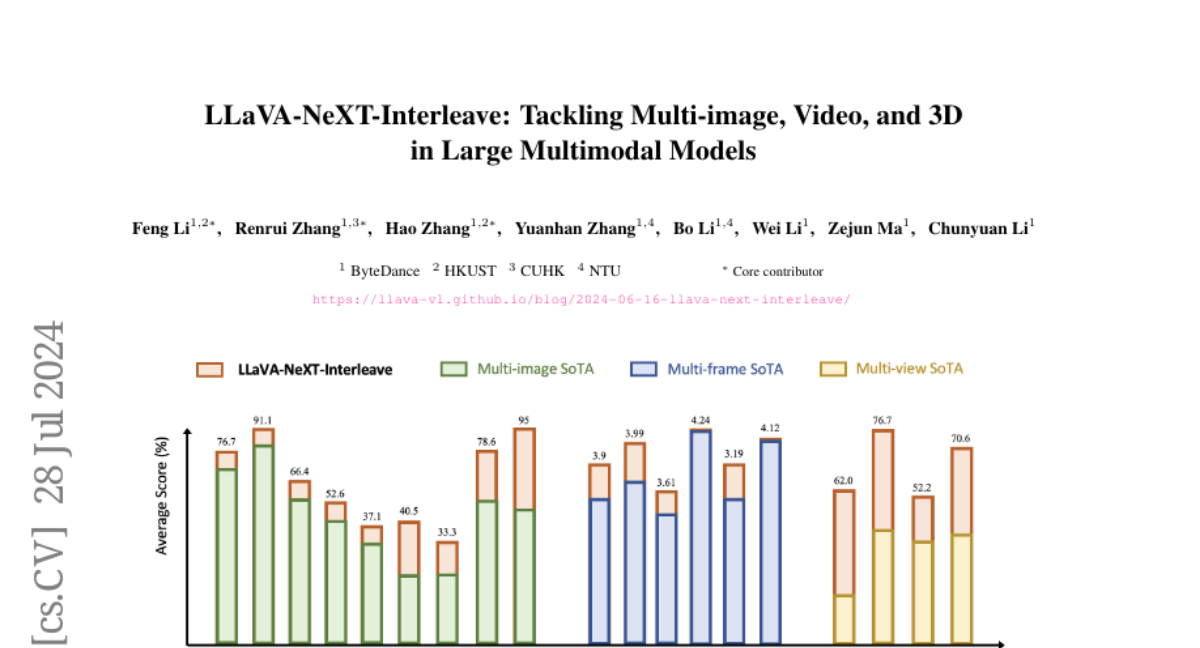
Summary
This paper talks about LLaVA-NeXT-Interleave, a new model designed to improve how large multimodal models (LMMs) handle various types of visual data, including multiple images, videos, and 3D views. It aims to enhance the capabilities of these models by allowing them to work with different scenarios simultaneously.
What's the problem?
The main problem is that most existing LMMs focus only on single-image tasks, which limits their ability to understand and process more complex visual inputs like multiple images or videos. Additionally, previous research has treated different types of visual scenarios separately, making it hard for models to generalize their learning across different contexts and tasks.
What's the solution?
To solve this issue, the authors introduced LLaVA-NeXT-Interleave, which is capable of processing multi-image, multi-frame (video), multi-view (3D), and multi-patch (single-image) data all at once. They created a new dataset called M4-Instruct that contains over 1.1 million samples across four main areas and 14 tasks. This dataset helps train the model to effectively handle various visual inputs. They also developed the LLaVA-Interleave Bench to evaluate how well the model performs across these different tasks.
Why it matters?
This research is important because it pushes the boundaries of what LMMs can do by enabling them to process and understand complex visual information more effectively. By improving how these models handle diverse types of data, LLaVA-NeXT-Interleave can lead to advancements in applications like video analysis, augmented reality, and other fields where understanding multiple forms of visual input is crucial.
Abstract
Visual instruction tuning has made considerable strides in enhancing the capabilities of Large Multimodal Models (LMMs). However, existing open LMMs largely focus on single-image tasks, their applications to multi-image scenarios remains less explored. Additionally, prior LMM research separately tackles different scenarios, leaving it impossible to generalize cross scenarios with new emerging capabilities. To this end, we introduce LLaVA-NeXT-Interleave, which simultaneously tackles Multi-image, Multi-frame (video), Multi-view (3D), and Multi-patch (single-image) scenarios in LMMs. To enable these capabilities, we regard the interleaved data format as a general template and compile the M4-Instruct dataset with 1,177.6k samples, spanning 4 primary domains with 14 tasks and 41 datasets. We also curate the LLaVA-Interleave Bench to comprehensively evaluate the multi-image performance of LMMs. Through extensive experiments, LLaVA-NeXT-Interleave achieves leading results in multi-image, video, and 3D benchmarks, while maintaining the performance of single-image tasks. Besides, our model also exhibits several emerging capabilities, e.g., transferring tasks across different settings and modalities. Code is available at https://github.com/LLaVA-VL/LLaVA-NeXT