LLaVA-OneVision: Easy Visual Task Transfer
Bo Li, Yuanhan Zhang, Dong Guo, Renrui Zhang, Feng Li, Hao Zhang, Kaichen Zhang, Yanwei Li, Ziwei Liu, Chunyuan Li
2024-08-07
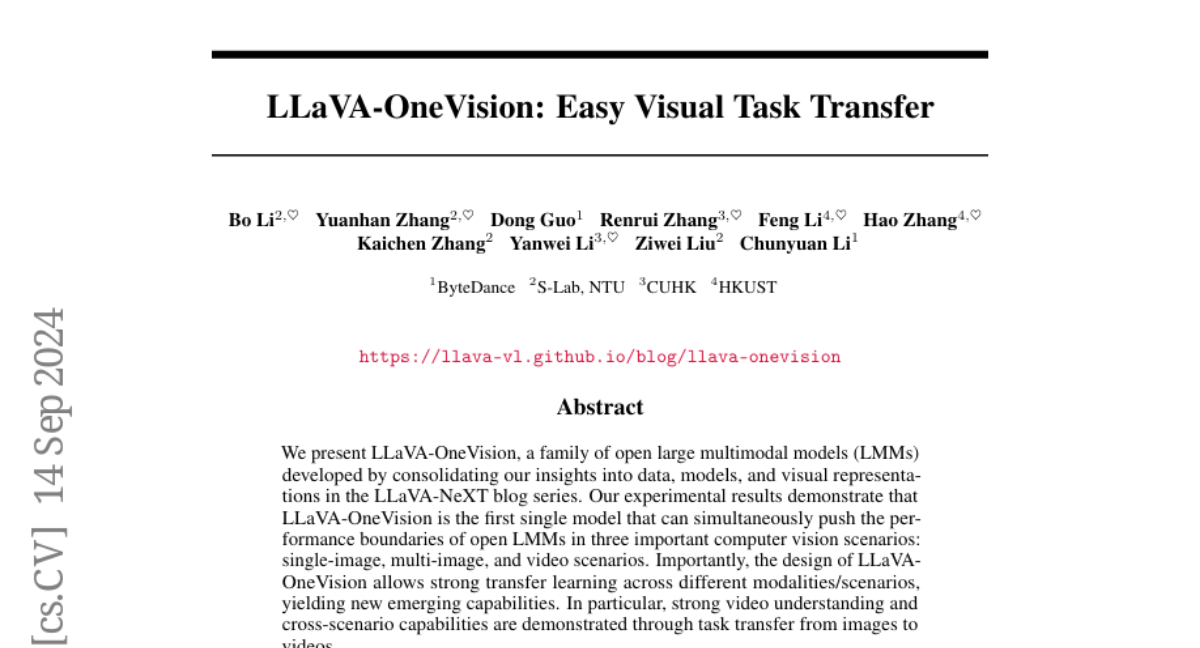
Summary
This paper introduces LLaVA-OneVision, a new model that can effectively handle various visual tasks involving single images, multiple images, and videos, making it easier to transfer learning between these different types of tasks.
What's the problem?
As technology advances, there is a growing need for models that can understand and process visual information from different sources, like single images, groups of images, and videos. However, many existing models struggle to perform well across these different scenarios, limiting their usefulness in real-world applications.
What's the solution?
LLaVA-OneVision is designed to overcome these challenges by being the first model that excels in all three scenarios simultaneously. It uses a method called transfer learning, which allows it to apply knowledge gained from one type of task (like analyzing images) to another (like understanding videos). This model has shown strong performance in video understanding by transferring skills learned from images to videos.
Why it matters?
LLaVA-OneVision is significant because it provides a more versatile tool for developers and researchers working with visual data. By improving how models can switch between different types of visual tasks, this research paves the way for creating smarter AI systems that can better assist users in various applications, such as video analysis, image recognition, and more.
Abstract
We present LLaVA-OneVision, a family of open large multimodal models (LMMs) developed by consolidating our insights into data, models, and visual representations in the LLaVA-NeXT blog series. Our experimental results demonstrate that LLaVA-OneVision is the first single model that can simultaneously push the performance boundaries of open LMMs in three important computer vision scenarios: single-image, multi-image, and video scenarios. Importantly, the design of LLaVA-OneVision allows strong transfer learning across different modalities/scenarios, yielding new emerging capabilities. In particular, strong video understanding and cross-scenario capabilities are demonstrated through task transfer from images to videos.