LLM-Agent-UMF: LLM-based Agent Unified Modeling Framework for Seamless Integration of Multi Active/Passive Core-Agents
Amine B. Hassouna, Hana Chaari, Ines Belhaj
2024-09-23
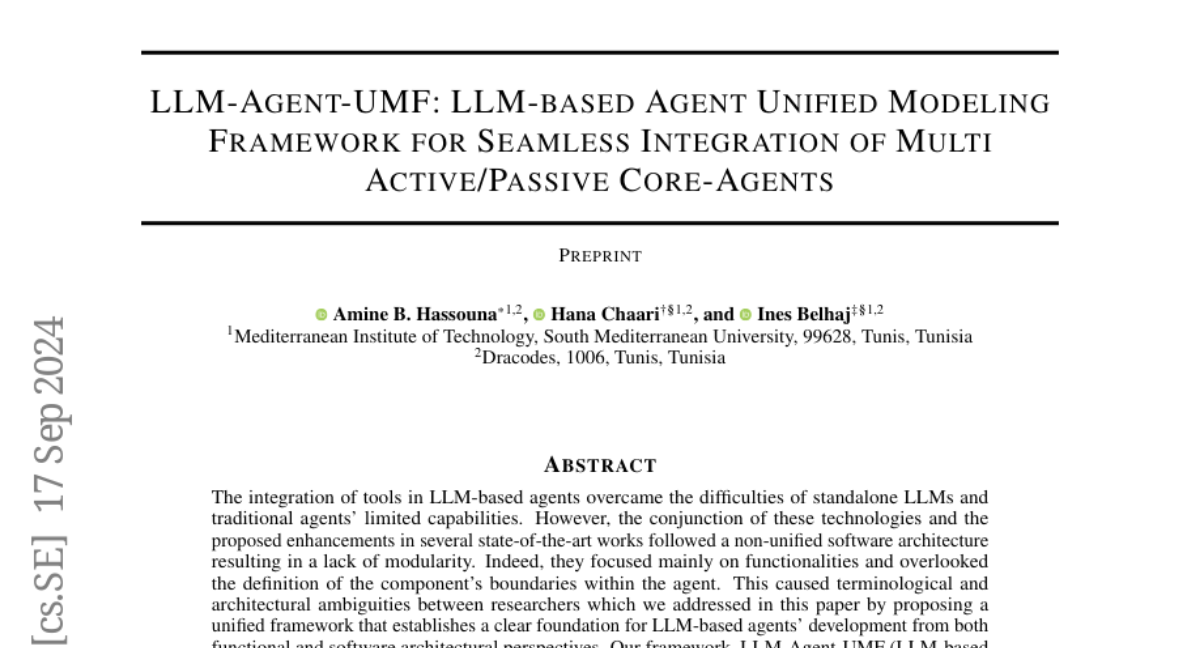
Summary
This paper introduces LLM-Agent-UMF, a new framework designed to improve the way large language model (LLM) agents are built and integrated. It aims to create a clear structure for combining different types of agents to enhance their capabilities in various tasks.
What's the problem?
Current methods for integrating LLMs and other tools into agents often lack a unified structure, leading to confusion among researchers and developers. This results in difficulties in understanding how different components work together, which can hinder the development of effective AI systems. Additionally, important aspects like security are often overlooked.
What's the solution?
To address these issues, the researchers proposed the LLM-Agent-UMF framework, which clearly defines the roles of different components within an agent. It introduces the concept of a core-agent that coordinates various tasks through five key modules: planning, memory, profile, action, and security. The framework also classifies core-agents into active and passive types based on their functions. By doing this, it allows for better organization and integration of multiple agents working together on complex tasks.
Why it matters?
This research is important because it lays the groundwork for creating more efficient and effective AI agents. By providing a clear structure for developing LLM-based agents, it helps researchers and developers communicate better and build systems that can tackle more complicated challenges. Furthermore, by including a focus on security, it ensures that these agents can operate safely in real-world applications.
Abstract
The integration of tools in LLM-based agents overcame the difficulties of standalone LLMs and traditional agents' limited capabilities. However, the conjunction of these technologies and the proposed enhancements in several state-of-the-art works followed a non-unified software architecture resulting in a lack of modularity. Indeed, they focused mainly on functionalities and overlooked the definition of the component's boundaries within the agent. This caused terminological and architectural ambiguities between researchers which we addressed in this paper by proposing a unified framework that establishes a clear foundation for LLM-based agents' development from both functional and software architectural perspectives. Our framework, LLM-Agent-UMF (LLM-based Agent Unified Modeling Framework), clearly distinguishes between the different components of an agent, setting LLMs, and tools apart from a newly introduced element: the core-agent, playing the role of the central coordinator of the agent which comprises five modules: planning, memory, profile, action, and security, the latter often neglected in previous works. Differences in the internal structure of core-agents led us to classify them into a taxonomy of passive and active types. Based on this, we proposed different multi-core agent architectures combining unique characteristics of various individual agents. For evaluation purposes, we applied this framework to a selection of state-of-the-art agents, thereby demonstrating its alignment with their functionalities and clarifying the overlooked architectural aspects. Moreover, we thoroughly assessed four of our proposed architectures by integrating distinctive agents into hybrid active/passive core-agents' systems. This analysis provided clear insights into potential improvements and highlighted the challenges involved in the combination of specific agents.