LLM-based Optimization of Compound AI Systems: A Survey
Matthieu Lin, Jenny Sheng, Andrew Zhao, Shenzhi Wang, Yang Yue, Yiran Wu, Huan Liu, Jun Liu, Gao Huang, Yong-Jin Liu
2024-10-23
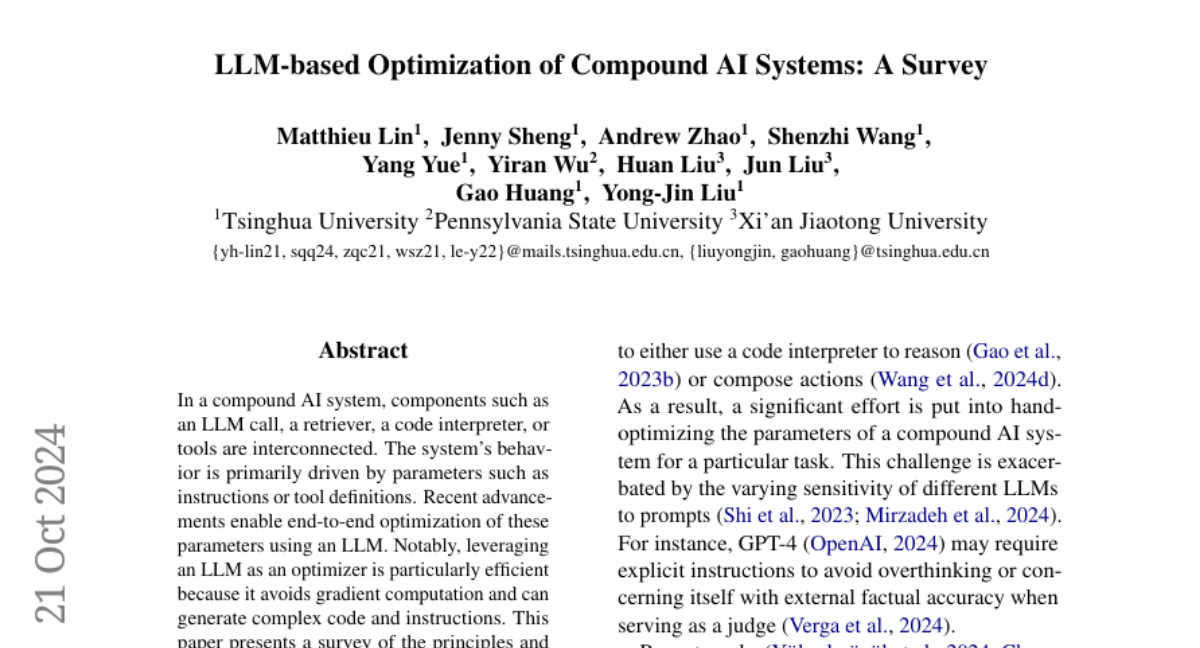
Summary
This paper surveys how large language models (LLMs) can be used to optimize complex AI systems that involve multiple interconnected components, like code interpreters and retrieval systems.
What's the problem?
In compound AI systems, which are made up of different parts working together, optimizing how these parts interact can be challenging. Traditionally, this optimization requires a lot of manual work to set parameters and instructions for each component, which can be time-consuming and inefficient.
What's the solution?
The authors propose using LLMs to automate the optimization process. By leveraging LLMs as optimizers, they can generate instructions and code without needing to calculate gradients manually. This allows for end-to-end optimization of the entire system, making it more efficient. The paper discusses various approaches and trends in this area, providing insights into how LLMs can improve the design and performance of compound AI systems.
Why it matters?
This research is important because it shows how integrating LLMs into the development of AI systems can lead to faster and more effective solutions. By automating the optimization process, researchers and developers can create better AI systems that are easier to manage and adapt to real-world needs.
Abstract
In a compound AI system, components such as an LLM call, a retriever, a code interpreter, or tools are interconnected. The system's behavior is primarily driven by parameters such as instructions or tool definitions. Recent advancements enable end-to-end optimization of these parameters using an LLM. Notably, leveraging an LLM as an optimizer is particularly efficient because it avoids gradient computation and can generate complex code and instructions. This paper presents a survey of the principles and emerging trends in LLM-based optimization of compound AI systems. It covers archetypes of compound AI systems, approaches to LLM-based end-to-end optimization, and insights into future directions and broader impacts. Importantly, this survey uses concepts from program analysis to provide a unified view of how an LLM optimizer is prompted to optimize a compound AI system. The exhaustive list of paper is provided at https://github.com/linyuhongg/LLM-based-Optimization-of-Compound-AI-Systems.