LLM Circuit Analyses Are Consistent Across Training and Scale
Curt Tigges, Michael Hanna, Qinan Yu, Stella Biderman
2024-07-16
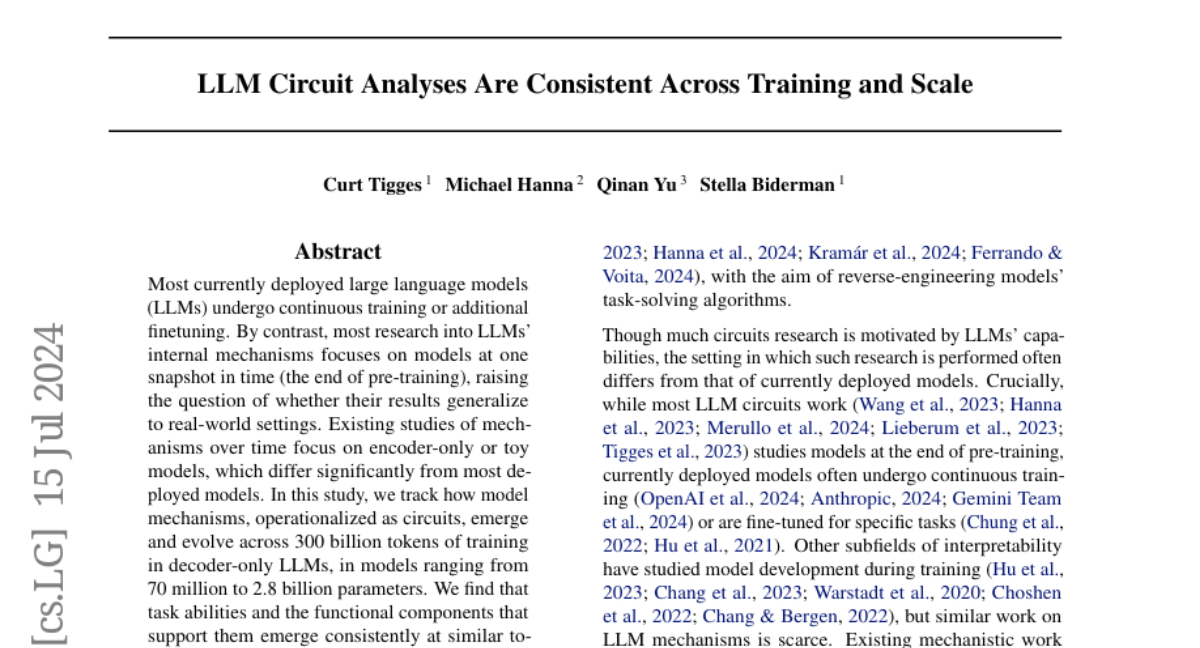
Summary
This paper discusses how the internal mechanisms of large language models (LLMs) evolve during training and whether these mechanisms remain consistent across different model sizes and training stages.
What's the problem?
Most research on LLMs focuses on their performance at a single point in time, usually at the end of their initial training. This raises concerns about whether these findings are applicable in real-world scenarios where models undergo continuous training and fine-tuning. Additionally, previous studies often examine simpler models that do not represent the complexity of currently deployed LLMs.
What's the solution?
The authors conducted a study tracking how the internal mechanisms, referred to as circuits, develop over 300 billion tokens of training in various decoder-only LLMs ranging from 70 million to 2.8 billion parameters. They found that important task abilities and the components that support them emerge consistently at similar points during training, regardless of model size. Even though the specific components might change roles over time, the overall algorithms they implement remain stable. This suggests that insights gained from analyzing smaller models can still apply to larger models after more training.
Why it matters?
This research is significant because it provides a deeper understanding of how LLMs function over time and across different sizes. By demonstrating that circuit analyses can generalize across training stages and model scales, it helps researchers make more informed predictions about model behavior in real-world applications. This could lead to improvements in how we design and train LLMs for various tasks.
Abstract
Most currently deployed large language models (LLMs) undergo continuous training or additional finetuning. By contrast, most research into LLMs' internal mechanisms focuses on models at one snapshot in time (the end of pre-training), raising the question of whether their results generalize to real-world settings. Existing studies of mechanisms over time focus on encoder-only or toy models, which differ significantly from most deployed models. In this study, we track how model mechanisms, operationalized as circuits, emerge and evolve across 300 billion tokens of training in decoder-only LLMs, in models ranging from 70 million to 2.8 billion parameters. We find that task abilities and the functional components that support them emerge consistently at similar token counts across scale. Moreover, although such components may be implemented by different attention heads over time, the overarching algorithm that they implement remains. Surprisingly, both these algorithms and the types of components involved therein can replicate across model scale. These results suggest that circuit analyses conducted on small models at the end of pre-training can provide insights that still apply after additional pre-training and over model scale.