LLM-DetectAIve: a Tool for Fine-Grained Machine-Generated Text Detection
Mervat Abassy, Kareem Elozeiri, Alexander Aziz, Minh Ngoc Ta, Raj Vardhan Tomar, Bimarsha Adhikari, Saad El Dine Ahmed, Yuxia Wang, Osama Mohammed Afzal, Zhuohan Xie, Jonibek Mansurov, Ekaterina Artemova, Vladislav Mikhailov, Rui Xing, Jiahui Geng, Hasan Iqbal, Zain Muhammad Mujahid, Tarek Mahmoud, Akim Tsvigun, Alham Fikri Aji, Artem Shelmanov, Nizar Habash
2024-08-09
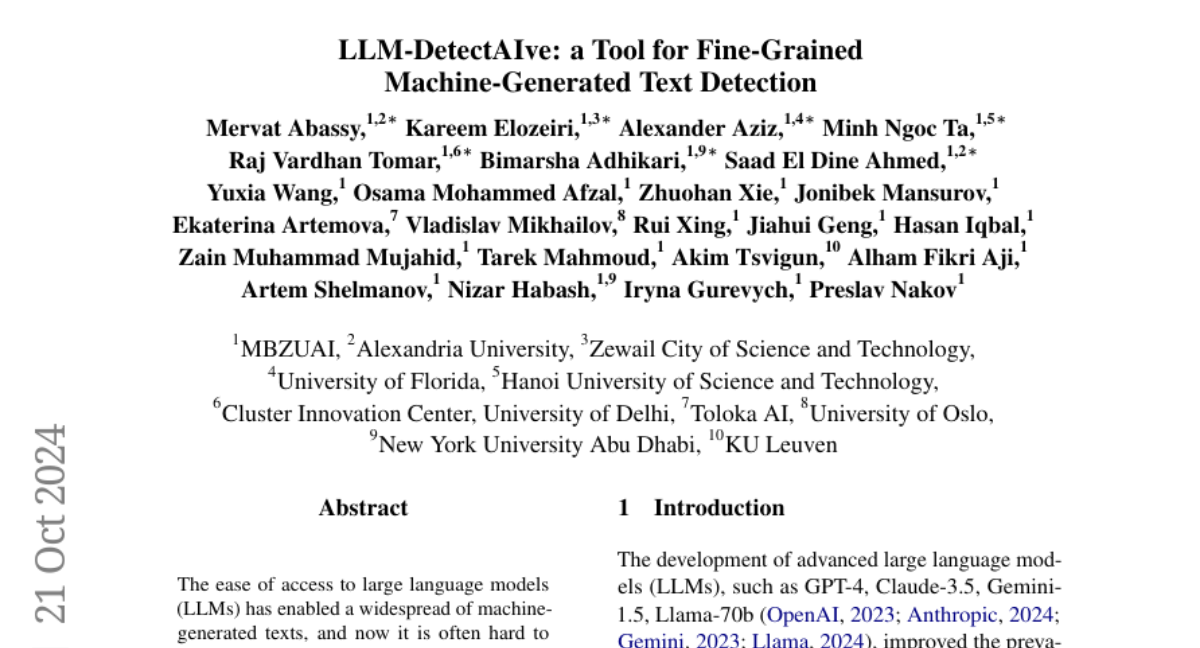
Summary
This paper presents LLM-DetectAIve, a tool designed to detect whether a piece of text was written by a human or generated by a machine, with a focus on providing detailed classifications.
What's the problem?
With the rise of large language models (LLMs) that can produce text similar to what humans write, it has become difficult to tell if a text is human-written or machine-generated. This is especially concerning in educational settings, where using machine-generated content might violate academic integrity. Existing detection tools typically only classify text as either human or machine-generated, which isn't enough to understand the nuances of how the text was created.
What's the solution?
LLM-DetectAIve improves on existing detection methods by categorizing texts into four distinct groups: human-written, machine-generated, machine-written but polished by a human, and human-written but polished by a machine. This fine-grained classification allows for better understanding and monitoring of how LLMs are used in different contexts. The tool was tested and shown to effectively identify the authorship of various texts, making it useful for educators and researchers.
Why it matters?
This research is important because it helps maintain academic integrity by providing a reliable way to identify the source of written content. By distinguishing between different types of text generation, LLM-DetectAIve can help educators ensure that students are not improperly using AI tools in their work, ultimately promoting fair practices in education and research.
Abstract
The widespread accessibility of large language models (LLMs) to the general public has significantly amplified the dissemination of machine-generated texts (MGTs). Advancements in prompt manipulation have exacerbated the difficulty in discerning the origin of a text (human-authored vs machinegenerated). This raises concerns regarding the potential misuse of MGTs, particularly within educational and academic domains. In this paper, we present LLM-DetectAIve -- a system designed for fine-grained MGT detection. It is able to classify texts into four categories: human-written, machine-generated, machine-written machine-humanized, and human-written machine-polished. Contrary to previous MGT detectors that perform binary classification, introducing two additional categories in LLM-DetectiAIve offers insights into the varying degrees of LLM intervention during the text creation. This might be useful in some domains like education, where any LLM intervention is usually prohibited. Experiments show that LLM-DetectAIve can effectively identify the authorship of textual content, proving its usefulness in enhancing integrity in education, academia, and other domains. LLM-DetectAIve is publicly accessible at https://huggingface.co/spaces/raj-tomar001/MGT-New. The video describing our system is available at https://youtu.be/E8eT_bE7k8c.