LLM-Mediated Guidance of MARL Systems
Philipp D. Siedler, Ian Gemp
2025-03-20
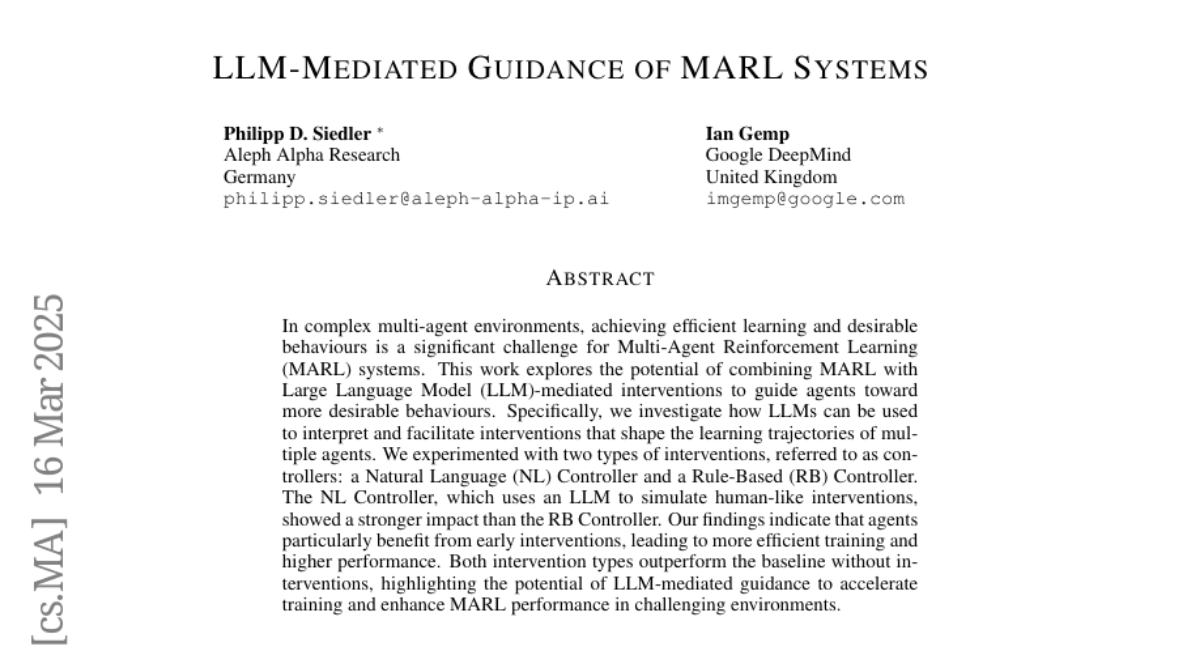
Summary
This paper explores how to use AI language models to help train other AI agents to work together better in complex environments.
What's the problem?
It's hard to train groups of AI agents to learn the right behaviors and work efficiently in complicated situations.
What's the solution?
The researchers used language models to guide the learning process of the AI agents, showing them what good behavior looks like and helping them learn faster.
Why it matters?
This work matters because it could lead to AI systems that are better at coordinating and collaborating in challenging real-world scenarios.
Abstract
In complex multi-agent environments, achieving efficient learning and desirable behaviours is a significant challenge for Multi-Agent Reinforcement Learning (MARL) systems. This work explores the potential of combining MARL with Large Language Model (LLM)-mediated interventions to guide agents toward more desirable behaviours. Specifically, we investigate how LLMs can be used to interpret and facilitate interventions that shape the learning trajectories of multiple agents. We experimented with two types of interventions, referred to as controllers: a Natural Language (NL) Controller and a Rule-Based (RB) Controller. The NL Controller, which uses an LLM to simulate human-like interventions, showed a stronger impact than the RB Controller. Our findings indicate that agents particularly benefit from early interventions, leading to more efficient training and higher performance. Both intervention types outperform the baseline without interventions, highlighting the potential of LLM-mediated guidance to accelerate training and enhance MARL performance in challenging environments.