LLM Pruning and Distillation in Practice: The Minitron Approach
Sharath Turuvekere Sreenivas, Saurav Muralidharan, Raviraj Joshi, Marcin Chochowski, Mostofa Patwary, Mohammad Shoeybi, Bryan Catanzaro, Jan Kautz, Pavlo Molchanov
2024-08-22
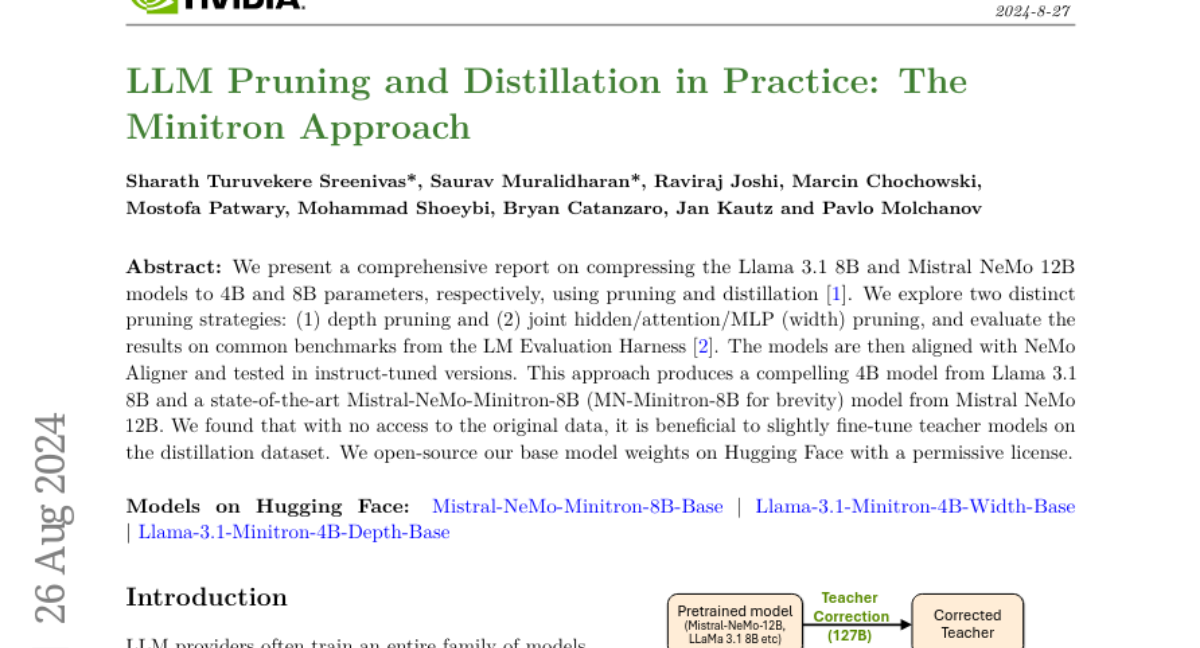
Summary
This paper discusses the Minitron approach, which focuses on making large language models smaller and more efficient through techniques called pruning and distillation.
What's the problem?
Large language models, like Llama 3.1 and Mistral NeMo, can be very powerful but also very large, which makes them slow and resource-intensive to use. This can be a problem when trying to deploy these models in real-world applications where speed and efficiency are important.
What's the solution?
The authors present a method to reduce the size of these models from 8 billion and 12 billion parameters down to 4 billion and 8 billion parameters, respectively. They explore two main strategies for this: depth pruning (removing less important layers) and width pruning (removing parts of the model that aren’t as necessary). They also fine-tune the models to improve their performance after pruning. This results in smaller models that still perform well on various tasks.
Why it matters?
This research is significant because it helps make powerful language models more accessible by reducing their size and resource requirements. Smaller models can be used in more applications, making advanced AI technologies available to a wider audience without needing expensive hardware.
Abstract
We present a comprehensive report on compressing the Llama 3.1 8B and Mistral NeMo 12B models to 4B and 8B parameters, respectively, using pruning and distillation. We explore two distinct pruning strategies: (1) depth pruning and (2) joint hidden/attention/MLP (width) pruning, and evaluate the results on common benchmarks from the LM Evaluation Harness. The models are then aligned with NeMo Aligner and tested in instruct-tuned versions. This approach produces a compelling 4B model from Llama 3.1 8B and a state-of-the-art Mistral-NeMo-Minitron-8B (MN-Minitron-8B for brevity) model from Mistral NeMo 12B. We found that with no access to the original data, it is beneficial to slightly fine-tune teacher models on the distillation dataset. We open-source our base model weights on Hugging Face with a permissive license.