LML: Language Model Learning a Dataset for Data-Augmented Prediction
Praneeth Vadlapati
2024-09-30
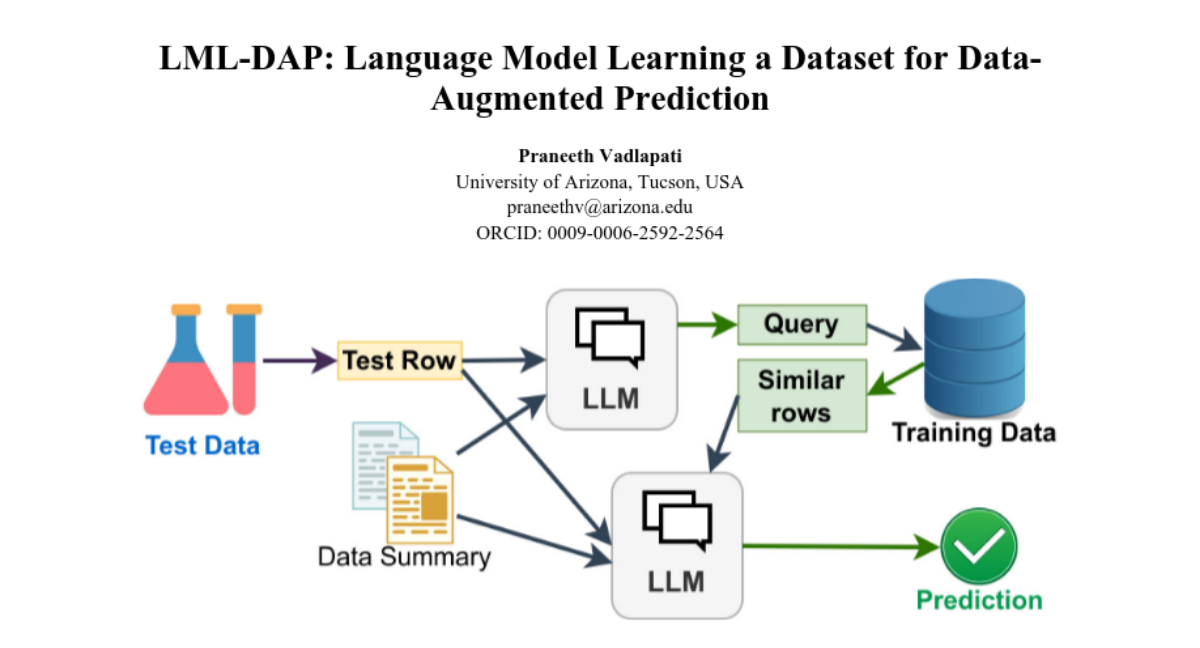
Summary
This paper presents a new method called Language Model Learning (LML) that uses Large Language Models (LLMs) for classifying data, making the process easier and more effective than traditional machine learning methods.
What's the problem?
Traditional machine learning models often require a lot of time and effort to clean data and create features, which can be complicated and inefficient. They may not always provide clear explanations for their predictions, making it hard to understand how they work.
What's the solution?
The authors propose LML, which simplifies the classification process by using a method called Data-Augmented Prediction (DAP). This method summarizes the training data to identify important features, automatically generates queries to find relevant information, and allows the LLM to make informed classifications. Additionally, it includes a feature that lets users see the reasoning behind each prediction, improving transparency.
Why it matters?
This research is significant because it shows how LLMs can be used effectively for classification tasks, potentially outperforming traditional models. By making the process easier and providing clear explanations for predictions, LML could help improve trust in AI systems and expand their applications in various fields.
Abstract
This paper introduces a new approach to using Large Language Models (LLMs) for classification tasks, which are typically handled using Machine Learning (ML) models. Unlike ML models that rely heavily on data cleaning and feature engineering, this method streamlines the process using LLMs. This paper proposes a new concept called "Language Model Learning (LML)" powered by a new method called "Data-Augmented Prediction (DAP)". The classification is performed by LLMs using a method similar to humans manually exploring and understanding the data and deciding classifications using data as a reference. Training data is summarized and evaluated to determine the features that lead to the classification of each label the most. In the process of DAP, the system uses the data summary to automatically create a query, which is used to retrieve relevant rows from the dataset. A classification is generated by the LLM using data summary and relevant rows, ensuring satisfactory accuracy even with complex data. Usage of data summary and similar data in DAP ensures context-aware decision-making. The proposed method uses the words "Act as an Explainable Machine Learning Model" in the prompt to enhance the interpretability of the predictions by allowing users to review the logic behind each prediction. In some test cases, the system scored an accuracy above 90%, proving the effectiveness of the system and its potential to outperform conventional ML models in various scenarios. The code is available at https://github.com/Pro-GenAI/LML-DAP