LoLDU: Low-Rank Adaptation via Lower-Diag-Upper Decomposition for Parameter-Efficient Fine-Tuning
Yiming Shi, Jiwei Wei, Yujia Wu, Ran Ran, Chengwei Sun, Shiyuan He, Yang Yang
2024-10-18
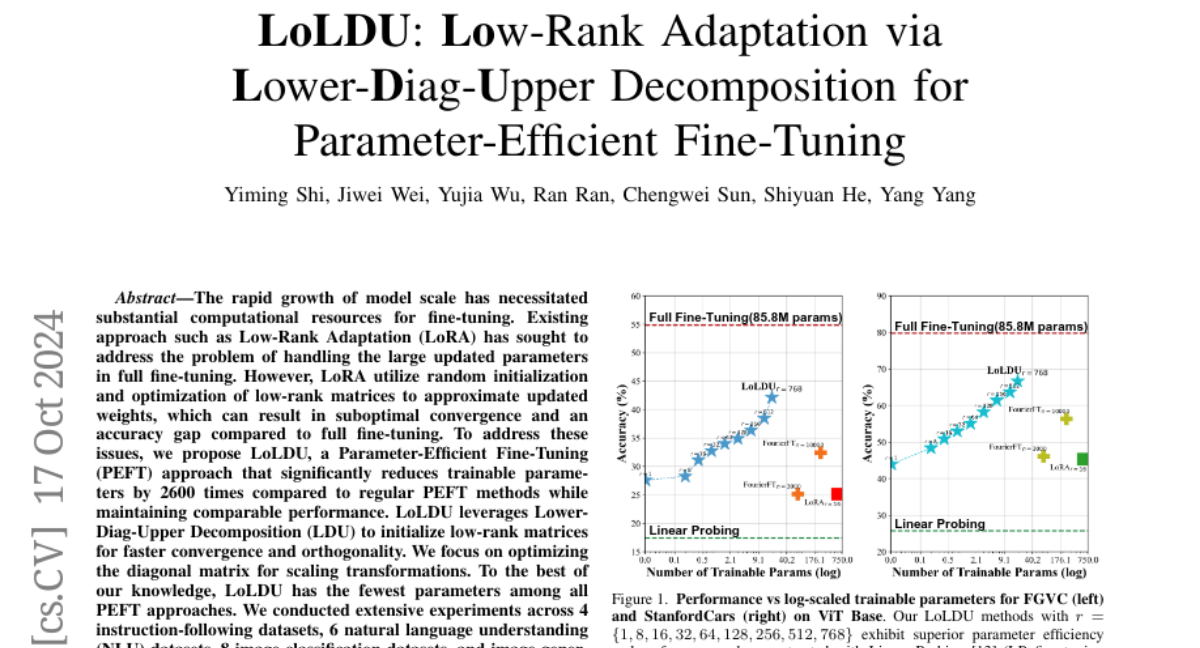
Summary
This paper introduces LoLDU, a new method for efficiently fine-tuning large language models (LLMs) by reducing the number of parameters that need to be trained while still maintaining good performance.
What's the problem?
As LLMs grow larger, they require a lot of computational power and resources for fine-tuning, which can be expensive and time-consuming. Existing methods, like Low-Rank Adaptation (LoRA), try to make this process easier but often use random setups that can lead to less accurate results compared to full fine-tuning.
What's the solution?
To overcome these challenges, the authors propose LoLDU (Low-Rank Adaptation via Lower-Diag-Upper Decomposition). This method significantly cuts down the number of parameters that need to be trained—by up to 2600 times compared to traditional methods—while still achieving similar performance. LoLDU uses a specific technique called Lower-Diag-Upper (LDU) decomposition to set up the training process more effectively. The authors conducted extensive tests across various datasets and found that their approach not only improved efficiency but also maintained high accuracy.
Why it matters?
This research is important because it makes it easier and cheaper to fine-tune powerful AI models. By reducing the resources needed for training while keeping performance high, LoLDU can help more researchers and developers use advanced language models in real-world applications, such as chatbots, translation services, and other AI-driven tools.
Abstract
The rapid growth of model scale has necessitated substantial computational resources for fine-tuning. Existing approach such as Low-Rank Adaptation (LoRA) has sought to address the problem of handling the large updated parameters in full fine-tuning. However, LoRA utilize random initialization and optimization of low-rank matrices to approximate updated weights, which can result in suboptimal convergence and an accuracy gap compared to full fine-tuning. To address these issues, we propose LoLDU, a Parameter-Efficient Fine-Tuning (PEFT) approach that significantly reduces trainable parameters by 2600 times compared to regular PEFT methods while maintaining comparable performance. LoLDU leverages Lower-Diag-Upper Decomposition (LDU) to initialize low-rank matrices for faster convergence and orthogonality. We focus on optimizing the diagonal matrix for scaling transformations. To the best of our knowledge, LoLDU has the fewest parameters among all PEFT approaches. We conducted extensive experiments across 4 instruction-following datasets, 6 natural language understanding (NLU) datasets, 8 image classification datasets, and image generation datasets with multiple model types (LLaMA2, RoBERTa, ViT, and Stable Diffusion), providing a comprehensive and detailed analysis. Our open-source code can be accessed at https://github.com/SKDDJ/LoLDU{https://github.com/SKDDJ/LoLDU}.