Long Video Diffusion Generation with Segmented Cross-Attention and Content-Rich Video Data Curation
Xin Yan, Yuxuan Cai, Qiuyue Wang, Yuan Zhou, Wenhao Huang, Huan Yang
2024-12-03
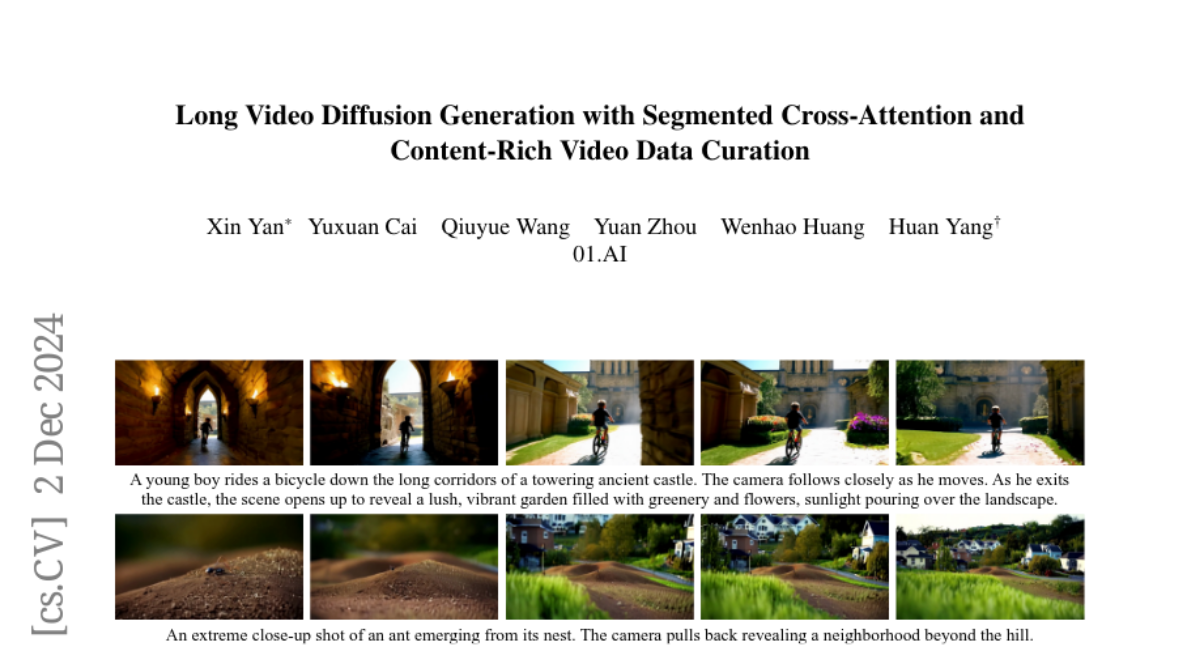
Summary
This paper introduces Presto, a new video generation model that creates 15-second videos with coherent storytelling and rich details using a method called Segmented Cross-Attention.
What's the problem?
Generating long videos that maintain a consistent story and high-quality content is challenging. Traditional methods often struggle to keep the video interesting and coherent over longer durations, which can lead to repetitive or disjointed scenes.
What's the solution?
Presto tackles this problem by using a technique called Segmented Cross-Attention (SCA). This method divides the video into segments and allows each segment to focus on specific parts of the accompanying text (sub-captions) that describe what is happening in the video. By doing this, Presto can generate videos that are not only coherent but also rich in content. Additionally, the researchers created a new dataset called LongTake-HD, which contains 261,000 videos with detailed captions to help train the model effectively.
Why it matters?
This research is important because it enhances the ability to create high-quality videos that tell a story over time, which can be useful in many fields such as filmmaking, education, and entertainment. By improving how videos are generated, Presto can help content creators produce more engaging and dynamic videos that capture viewers' attention.
Abstract
We introduce Presto, a novel video diffusion model designed to generate 15-second videos with long-range coherence and rich content. Extending video generation methods to maintain scenario diversity over long durations presents significant challenges. To address this, we propose a Segmented Cross-Attention (SCA) strategy, which splits hidden states into segments along the temporal dimension, allowing each segment to cross-attend to a corresponding sub-caption. SCA requires no additional parameters, enabling seamless incorporation into current DiT-based architectures. To facilitate high-quality long video generation, we build the LongTake-HD dataset, consisting of 261k content-rich videos with scenario coherence, annotated with an overall video caption and five progressive sub-captions. Experiments show that our Presto achieves 78.5% on the VBench Semantic Score and 100% on the Dynamic Degree, outperforming existing state-of-the-art video generation methods. This demonstrates that our proposed Presto significantly enhances content richness, maintains long-range coherence, and captures intricate textual details. More details are displayed on our project page: https://presto-video.github.io/.