LongReward: Improving Long-context Large Language Models with AI Feedback
Jiajie Zhang, Zhongni Hou, Xin Lv, Shulin Cao, Zhenyu Hou, Yilin Niu, Lei Hou, Yuxiao Dong, Ling Feng, Juanzi Li
2024-10-29
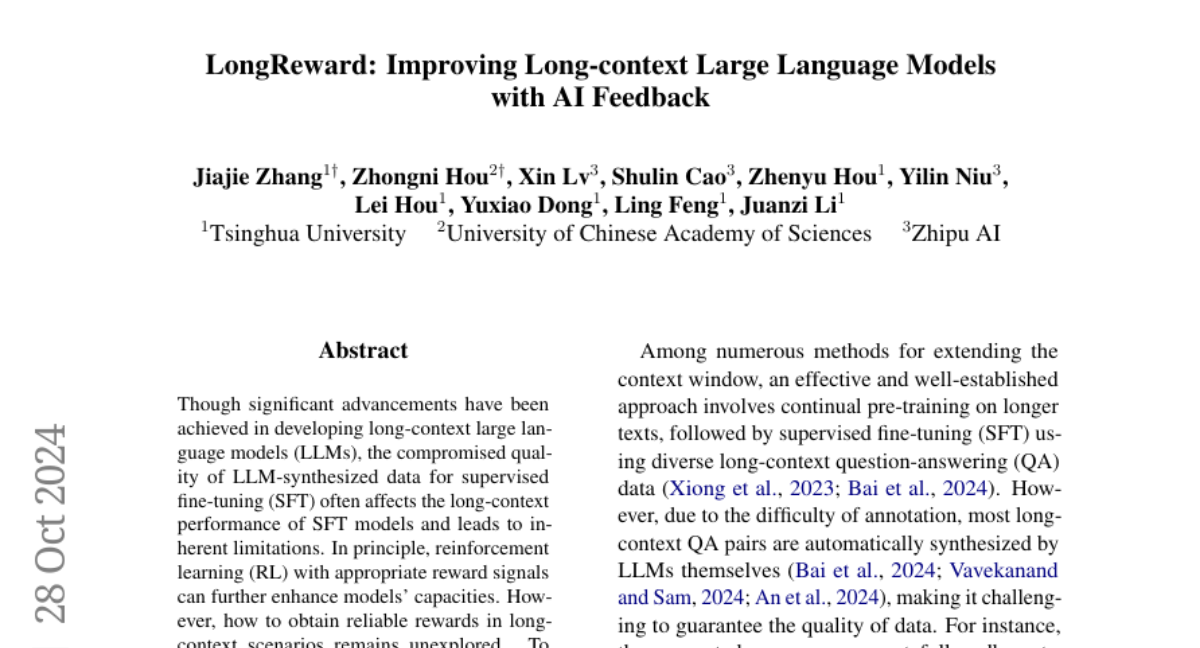
Summary
This paper presents LongReward, a new method that improves the performance of large language models (LLMs) when handling long pieces of text by using AI-generated feedback.
What's the problem?
While LLMs have made great progress, they often struggle with understanding and generating responses for long texts. This is partly because the data used to train them can be of lower quality, which affects how well they learn to handle long contexts. Additionally, finding reliable ways to give feedback during training for these long contexts has not been well explored.
What's the solution?
To address these challenges, the authors developed LongReward, which uses another LLM to provide feedback on how well the model's responses meet four important criteria: helpfulness, logicality, faithfulness, and completeness. This feedback acts as a reward signal during training. By combining LongReward with an offline reinforcement learning algorithm called DPO, they were able to significantly enhance the performance of LLMs on tasks that require understanding long texts. Their experiments showed that this method not only improved long-context performance but also helped the models follow short instructions better.
Why it matters?
This research is important because it demonstrates a new way to enhance the capabilities of language models, making them more effective at processing and generating long texts. This can lead to better applications in various fields such as education, content creation, and customer service, where understanding complex information is crucial.
Abstract
Though significant advancements have been achieved in developing long-context large language models (LLMs), the compromised quality of LLM-synthesized data for supervised fine-tuning (SFT) often affects the long-context performance of SFT models and leads to inherent limitations. In principle, reinforcement learning (RL) with appropriate reward signals can further enhance models' capacities. However, how to obtain reliable rewards in long-context scenarios remains unexplored. To this end, we propose LongReward, a novel method that utilizes an off-the-shelf LLM to provide rewards for long-context model responses from four human-valued dimensions: helpfulness, logicality, faithfulness, and completeness, each with a carefully designed assessment pipeline. By combining LongReward and offline RL algorithm DPO, we are able to effectively improve long-context SFT models. Our experiments indicate that LongReward not only significantly improves models' long-context performance but also enhances their ability to follow short instructions. We also find that long-context DPO with LongReward and conventional short-context DPO can be used together without hurting either one's performance.