LongVILA: Scaling Long-Context Visual Language Models for Long Videos
Fuzhao Xue, Yukang Chen, Dacheng Li, Qinghao Hu, Ligeng Zhu, Xiuyu Li, Yunhao Fang, Haotian Tang, Shang Yang, Zhijian Liu, Ethan He, Hongxu Yin, Pavlo Molchanov, Jan Kautz, Linxi Fan, Yuke Zhu, Yao Lu, Song Han
2024-08-20
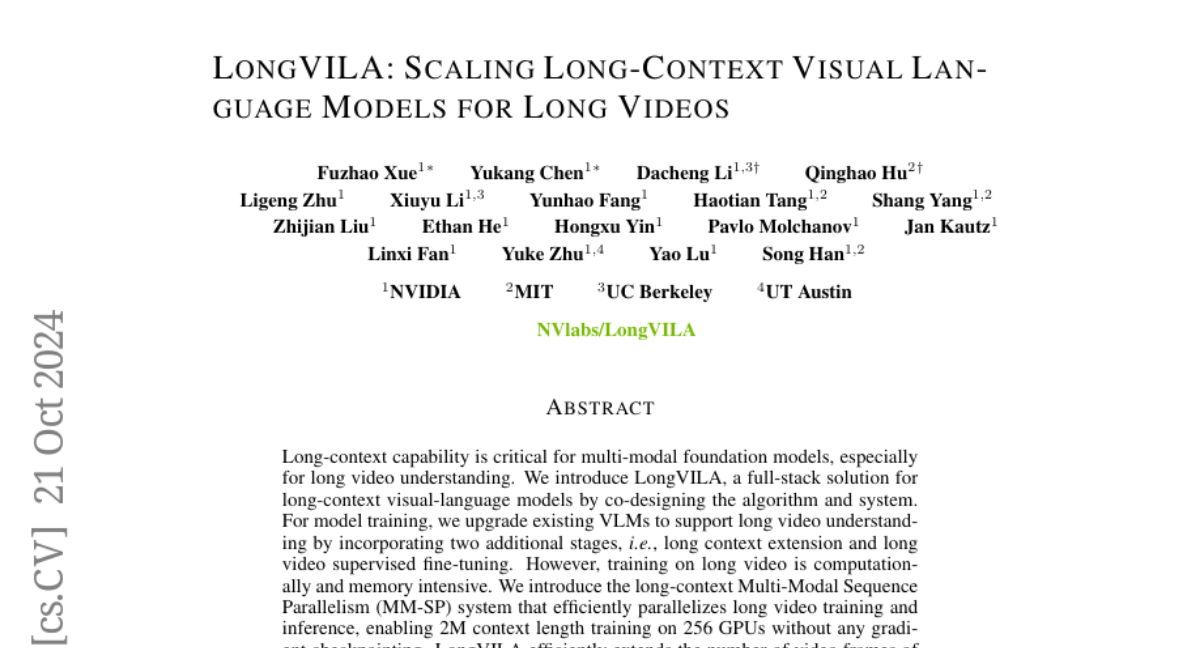
Summary
This paper presents LongVILA, a new system designed to improve how visual language models can handle long videos by using advanced training techniques and datasets.
What's the problem?
Many existing models struggle to process long videos effectively due to limitations in their ability to remember and analyze extended sequences of frames. This makes it difficult for them to understand and generate accurate descriptions for longer video content.
What's the solution?
LongVILA introduces a comprehensive approach that includes a new system for training called Multi-Modal Sequence Parallelism (MM-SP), which allows the model to handle longer contexts more efficiently. It also uses a detailed training pipeline that involves multiple stages to improve the model's understanding of video content. Additionally, LongVILA is supported by large-scale datasets specifically designed for training on long videos, enabling it to analyze up to 1024 frames at once.
Why it matters?
This research is important because it enhances the capabilities of visual language models, making them better suited for real-world applications that involve long videos, such as video summarization, content creation, and educational tools. By improving how these models process and understand lengthy video content, we can create more effective AI systems that can assist in various fields.
Abstract
Long-context capability is critical for multi-modal foundation models. We introduce LongVILA, a full-stack solution for long-context vision-language models, including system, model training, and dataset development. On the system side, we introduce the first Multi-Modal Sequence Parallelism (MM-SP) system that enables long-context training and inference, enabling 2M context length training on 256 GPUs. MM-SP is also efficient, being 2.1x - 5.7x faster than Ring-Style Sequence Parallelism and 1.1x - 1.4x faster than Megatron-LM in text-only settings. Moreover, it seamlessly integrates with Hugging Face Transformers. For model training, we propose a five-stage pipeline comprising alignment, pre-training, context extension, and long-short joint supervised fine-tuning. Regarding datasets, we meticulously construct large-scale visual language pre-training datasets and long video instruction-following datasets to support our multi-stage training process. The full-stack solution extends the feasible frame number of VILA by a factor of 128 (from 8 to 1024 frames) and improves long video captioning score from 2.00 to 3.26 (1.6x), achieving 99.5% accuracy in 1400-frames video (274k context length) needle in a haystack. LongVILA-8B also demonstrates a consistent improvement in performance on long videos within the VideoMME benchmark as the video frames increase.