Lookback Lens: Detecting and Mitigating Contextual Hallucinations in Large Language Models Using Only Attention Maps
Yung-Sung Chuang, Linlu Qiu, Cheng-Yu Hsieh, Ranjay Krishna, Yoon Kim, James Glass
2024-07-10
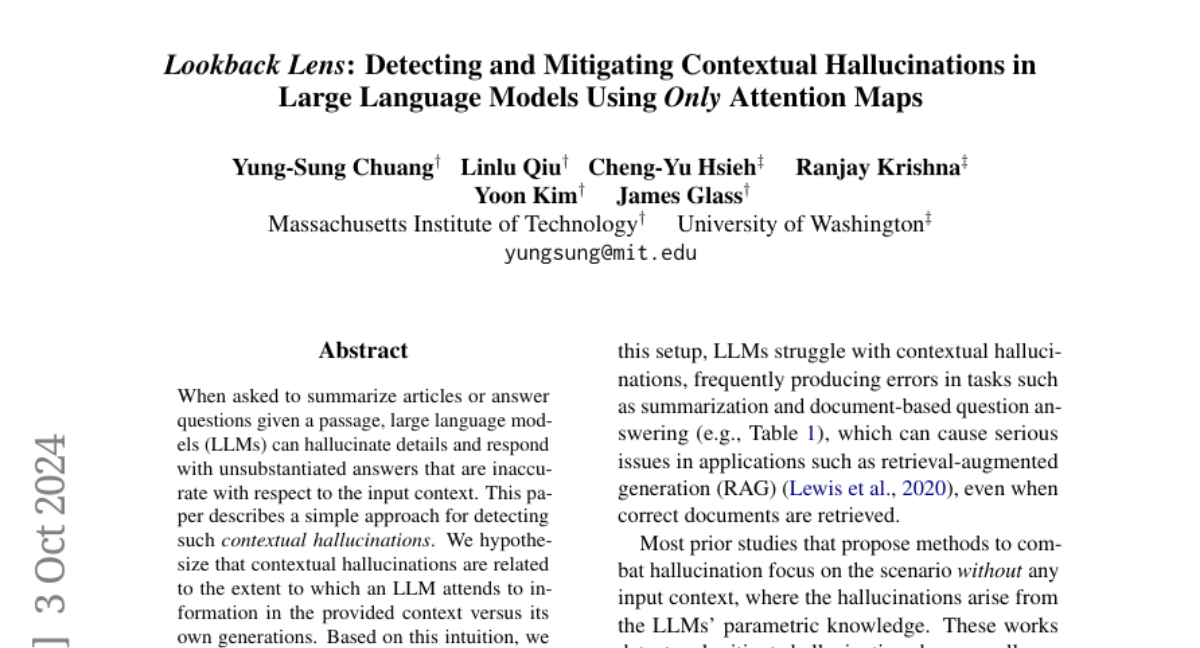
Summary
This paper talks about Lookback Lens, a new method designed to detect and reduce inaccuracies in large language models (LLMs) when they generate responses. It focuses on identifying when these models create false information that doesn't match the input context.
What's the problem?
The main problem is that LLMs often produce answers that include made-up details or inaccuracies, known as hallucinations. This happens when the model relies too much on its own generated content rather than the actual information provided in the context, leading to misleading or incorrect responses.
What's the solution?
To tackle this issue, the authors developed Lookback Lens, which detects hallucinations by analyzing attention maps from the model. They hypothesize that if a model pays more attention to its own generated tokens than to the context it was given, it is likely to hallucinate. Lookback Lens uses a simple linear classifier that compares the attention given to the original context versus the generated content. This method has been shown to work effectively across different tasks and even different model sizes without needing retraining. Additionally, they implemented a classifier-guided decoding approach that helps reduce hallucinations in generated outputs, achieving a notable decrease in inaccuracies during summarization tasks.
Why it matters?
This research is important because it provides a straightforward way to improve the reliability of LLMs by reducing hallucinations. By enhancing how these models process and respond to information, Lookback Lens can lead to more accurate and trustworthy AI applications in areas like education, content creation, and customer service, where accurate information is crucial.
Abstract
When asked to summarize articles or answer questions given a passage, large language models (LLMs) can hallucinate details and respond with unsubstantiated answers that are inaccurate with respect to the input context. This paper describes a simple approach for detecting such contextual hallucinations. We hypothesize that contextual hallucinations are related to the extent to which an LLM attends to information in the provided context versus its own generations. Based on this intuition, we propose a simple hallucination detection model whose input features are given by the ratio of attention weights on the context versus newly generated tokens (for each attention head). We find that a linear classifier based on these lookback ratio features is as effective as a richer detector that utilizes the entire hidden states of an LLM or a text-based entailment model. The lookback ratio-based detector -- Lookback Lens -- is found to transfer across tasks and even models, allowing a detector that is trained on a 7B model to be applied (without retraining) to a larger 13B model. We further apply this detector to mitigate contextual hallucinations, and find that a simple classifier-guided decoding approach is able to reduce the amount of hallucination, for example by 9.6% in the XSum summarization task.