LoRA-Contextualizing Adaptation of Large Multimodal Models for Long Document Understanding
Jian Chen, Ruiyi Zhang, Yufan Zhou, Tong Yu, Franck Dernoncourt, Jiuxiang Gu, Ryan A. Rossi, Changyou Chen, Tong Sun
2024-11-05
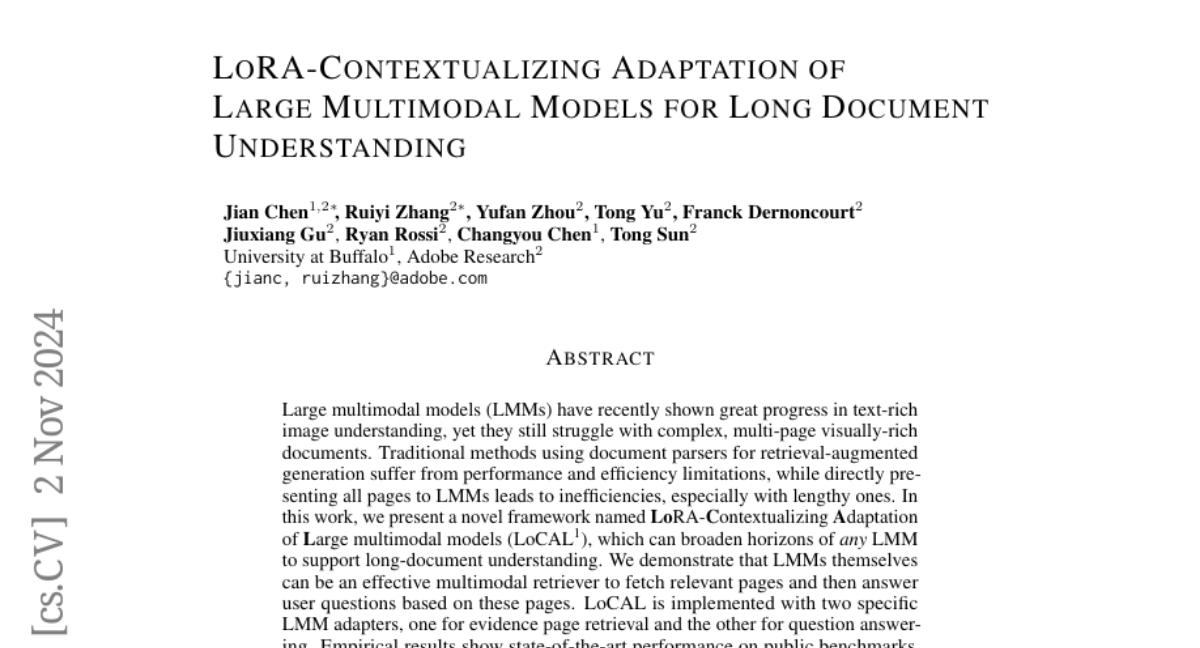
Summary
This paper introduces LoRA-Contextualizing Adaptation of Large Multimodal Models (LoCAL), a new framework that helps large multimodal models (LMMs) better understand and work with long, complex documents that contain both text and images.
What's the problem?
Large multimodal models have made progress in understanding images and text, but they still struggle with lengthy, multi-page documents that are visually rich. Traditional methods for processing these documents often rely on document parsers, which can be inefficient and perform poorly. Presenting all pages of a long document to an LMM at once can also lead to inefficiencies, making it difficult for the model to provide accurate answers to user questions.
What's the solution?
LoCAL addresses these challenges by allowing LMMs to act as multimodal retrievers, meaning they can fetch relevant pages from long documents based on user queries. The framework includes two specific adapters: one for retrieving evidence pages and another for answering questions. This setup helps the model focus on the most relevant information without getting overwhelmed by unnecessary details. The authors tested LoCAL and found that it performed exceptionally well on public benchmarks, demonstrating its effectiveness in understanding long documents.
Why it matters?
This research is significant because it enhances the capabilities of AI systems in processing complex documents, making them more useful for tasks like research, education, and data analysis. By improving how LMMs handle long texts with images, LoCAL can help users retrieve important information more efficiently and accurately.
Abstract
Large multimodal models (LMMs) have recently shown great progress in text-rich image understanding, yet they still struggle with complex, multi-page, visually-rich documents. Traditional methods using document parsers for retrieval-augmented generation suffer from performance and efficiency limitations, while directly presenting all pages to LMMs leads to inefficiencies, especially with lengthy documents. In this work, we present a novel framework named LoRA-Contextualizing Adaptation of Large multimodal models (LoCAL), which broadens the capabilities of any LMM to support long-document understanding. We demonstrate that LMMs can effectively serve as multimodal retrievers, fetching relevant pages to answer user questions based on these pages. LoCAL is implemented with two specific LMM adapters: one for evidence page retrieval and another for question answering. Empirical results show state-of-the-art performance on public benchmarks, demonstrating the effectiveness of LoCAL.