Lumina-mGPT: Illuminate Flexible Photorealistic Text-to-Image Generation with Multimodal Generative Pretraining
Dongyang Liu, Shitian Zhao, Le Zhuo, Weifeng Lin, Yu Qiao, Hongsheng Li, Peng Gao
2024-08-06
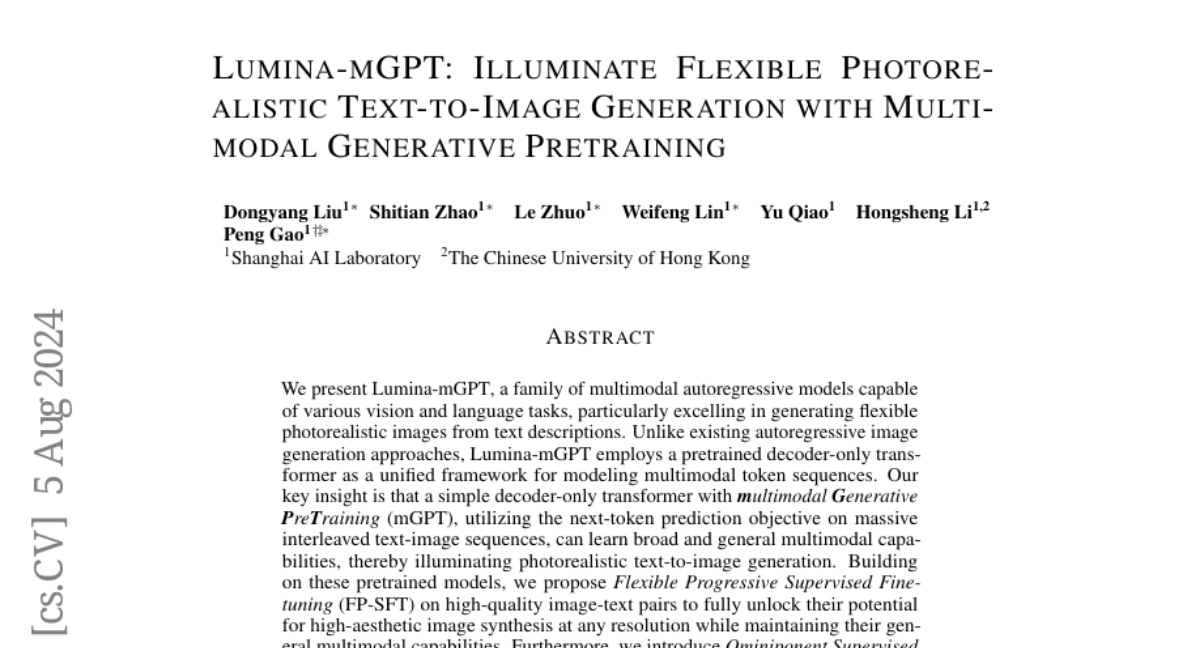
Summary
This paper introduces Lumina-mGPT, a new model that can generate high-quality, photorealistic images from text descriptions. It combines advanced techniques in language and image processing to perform various tasks effectively.
What's the problem?
Many existing models for generating images from text struggle with flexibility and quality, especially when trying to create detailed and realistic images. Additionally, they often require complex setups and are not efficient for different tasks, making them less useful for practical applications.
What's the solution?
Lumina-mGPT uses a pretrained decoder-only transformer that learns from both text and image data. This allows it to generate images based on text prompts efficiently. The model incorporates two new training methods: Flexible Progressive Supervised Finetuning (FP-SFT), which improves image quality at different resolutions, and Ominiponent Supervised Finetuning (Omni-SFT), which enables the model to handle a variety of tasks like image generation, recognition, and question answering all in one system. This unified approach enhances its performance across multiple applications.
Why it matters?
This research is significant because it makes it easier to create high-quality images from text, which can be applied in many fields such as art, design, and education. By improving the capabilities of multimodal models like Lumina-mGPT, we can unlock new possibilities for AI in creative industries and enhance how we interact with technology.
Abstract
We present Lumina-mGPT, a family of multimodal autoregressive models capable of various vision and language tasks, particularly excelling in generating flexible photorealistic images from text descriptions. Unlike existing autoregressive image generation approaches, Lumina-mGPT employs a pretrained decoder-only transformer as a unified framework for modeling multimodal token sequences. Our key insight is that a simple decoder-only transformer with multimodal Generative PreTraining (mGPT), utilizing the next-token prediction objective on massive interleaved text-image sequences, can learn broad and general multimodal capabilities, thereby illuminating photorealistic text-to-image generation. Building on these pretrained models, we propose Flexible Progressive Supervised Finetuning (FP-SFT) on high-quality image-text pairs to fully unlock their potential for high-aesthetic image synthesis at any resolution while maintaining their general multimodal capabilities. Furthermore, we introduce Ominiponent Supervised Finetuning (Omni-SFT), transforming Lumina-mGPT into a foundation model that seamlessly achieves omnipotent task unification. The resulting model demonstrates versatile multimodal capabilities, including visual generation tasks like flexible text-to-image generation and controllable generation, visual recognition tasks like segmentation and depth estimation, and vision-language tasks like multiturn visual question answering. Additionally, we analyze the differences and similarities between diffusion-based and autoregressive methods in a direct comparison.