LVCD: Reference-based Lineart Video Colorization with Diffusion Models
Zhitong Huang, Mohan Zhang, Jing Liao
2024-09-20
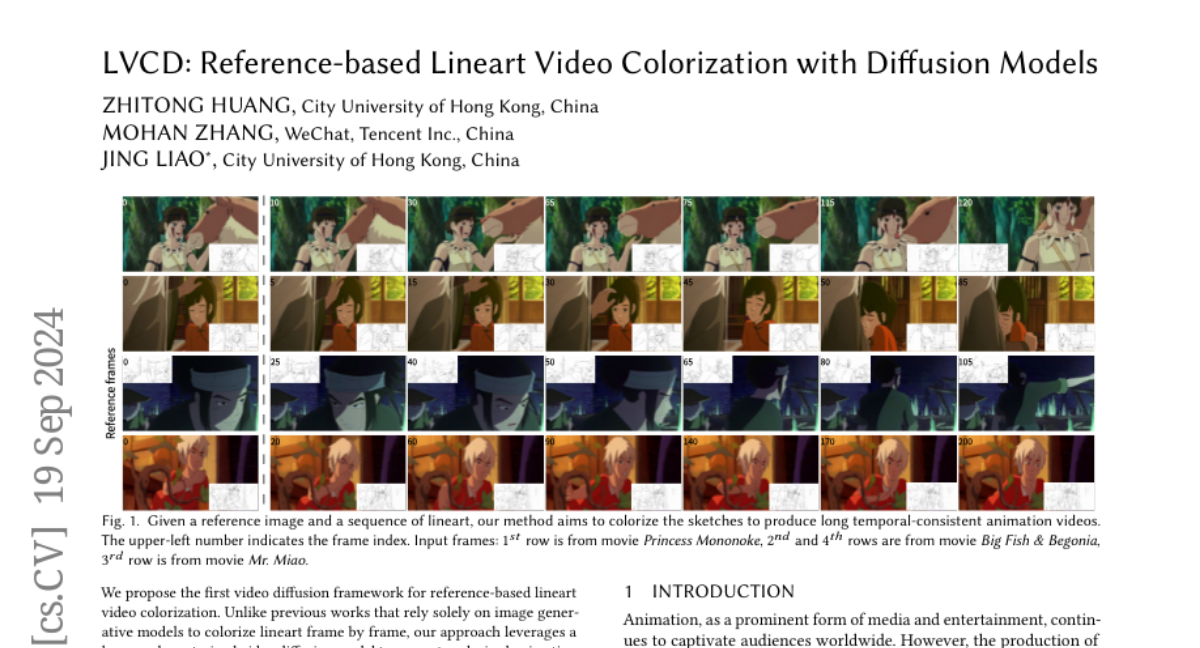
Summary
This paper introduces LVCD, a new framework for colorizing lineart videos using a reference frame, which improves the quality and consistency of animated videos by utilizing advanced diffusion models.
What's the problem?
Previous methods for colorizing lineart animations often worked on each frame individually, leading to inconsistent colors and poor handling of large movements in the animation. This makes the final product look choppy or unrealistic, especially in scenes with a lot of action.
What's the solution?
The authors developed LVCD, which uses a video diffusion model to colorize animations more effectively. They introduced several key innovations: Sketch-guided ControlNet allows for better control over how the model generates video based on lineart sketches; Reference Attention helps transfer colors from a reference frame to other frames, even during fast movements; and a new sequential sampling method enables the model to create longer animations without losing quality. Together, these features help maintain color consistency and improve the overall quality of the animations.
Why it matters?
This research is significant because it enhances the ability to create high-quality animated videos from simple lineart drawings. By improving how colors are applied in animations, LVCD can be useful in various fields like animation production, video games, and educational content, allowing creators to produce visually appealing results more efficiently.
Abstract
We propose the first video diffusion framework for reference-based lineart video colorization. Unlike previous works that rely solely on image generative models to colorize lineart frame by frame, our approach leverages a large-scale pretrained video diffusion model to generate colorized animation videos. This approach leads to more temporally consistent results and is better equipped to handle large motions. Firstly, we introduce Sketch-guided ControlNet which provides additional control to finetune an image-to-video diffusion model for controllable video synthesis, enabling the generation of animation videos conditioned on lineart. We then propose Reference Attention to facilitate the transfer of colors from the reference frame to other frames containing fast and expansive motions. Finally, we present a novel scheme for sequential sampling, incorporating the Overlapped Blending Module and Prev-Reference Attention, to extend the video diffusion model beyond its original fixed-length limitation for long video colorization. Both qualitative and quantitative results demonstrate that our method significantly outperforms state-of-the-art techniques in terms of frame and video quality, as well as temporal consistency. Moreover, our method is capable of generating high-quality, long temporal-consistent animation videos with large motions, which is not achievable in previous works. Our code and model are available at https://luckyhzt.github.io/lvcd.