LVSM: A Large View Synthesis Model with Minimal 3D Inductive Bias
Haian Jin, Hanwen Jiang, Hao Tan, Kai Zhang, Sai Bi, Tianyuan Zhang, Fujun Luan, Noah Snavely, Zexiang Xu
2024-10-24
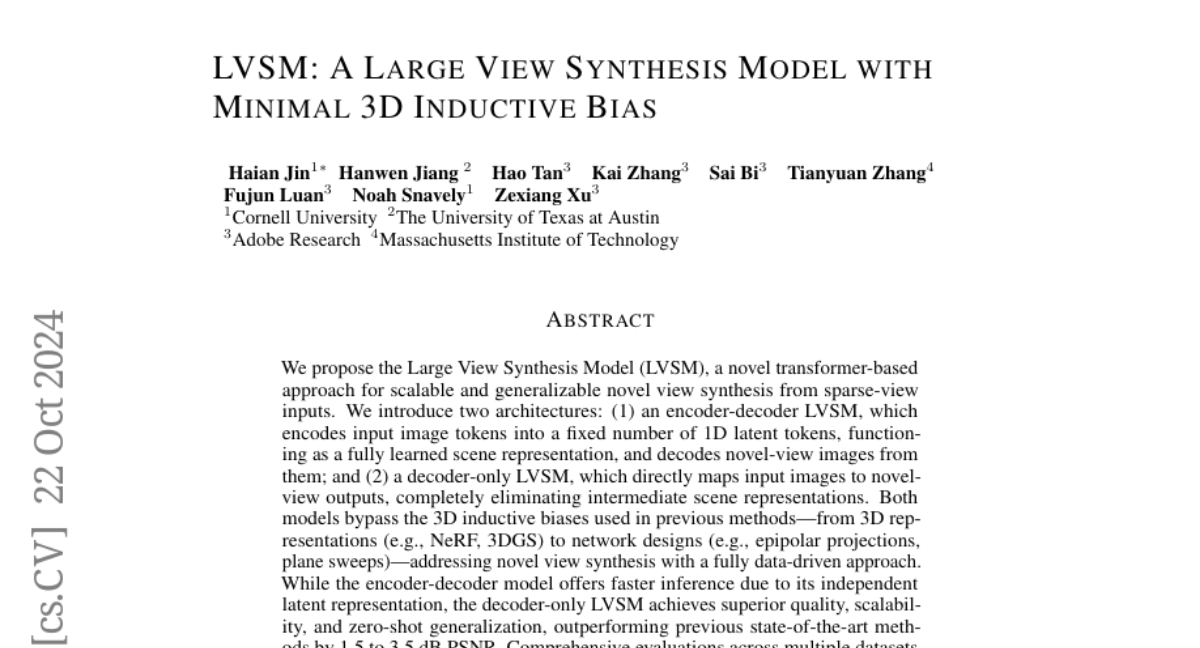
Summary
This paper presents LVSM, a new model that generates high-quality images from sparse inputs by using a transformer-based approach without relying on traditional 3D methods.
What's the problem?
Creating new views of a scene from limited images can be challenging because existing methods often depend on complex 3D representations. These methods can be slow and may not work well with fewer input images, leading to lower quality results.
What's the solution?
The authors introduce two versions of the Large View Synthesis Model (LVSM). The first is an encoder-decoder model that processes input images into a simplified format and then generates new views. The second is a decoder-only model that skips the intermediate steps and directly produces new images from the input. Both models avoid traditional 3D biases, allowing them to learn directly from data and perform better in generating novel views.
Why it matters?
This research is significant because it improves how we can create realistic images from limited data, which is useful in various applications like virtual reality, gaming, and film production. By enhancing the efficiency and quality of image generation, LVSM opens up new possibilities for visual content creation.
Abstract
We propose the Large View Synthesis Model (LVSM), a novel transformer-based approach for scalable and generalizable novel view synthesis from sparse-view inputs. We introduce two architectures: (1) an encoder-decoder LVSM, which encodes input image tokens into a fixed number of 1D latent tokens, functioning as a fully learned scene representation, and decodes novel-view images from them; and (2) a decoder-only LVSM, which directly maps input images to novel-view outputs, completely eliminating intermediate scene representations. Both models bypass the 3D inductive biases used in previous methods -- from 3D representations (e.g., NeRF, 3DGS) to network designs (e.g., epipolar projections, plane sweeps) -- addressing novel view synthesis with a fully data-driven approach. While the encoder-decoder model offers faster inference due to its independent latent representation, the decoder-only LVSM achieves superior quality, scalability, and zero-shot generalization, outperforming previous state-of-the-art methods by 1.5 to 3.5 dB PSNR. Comprehensive evaluations across multiple datasets demonstrate that both LVSM variants achieve state-of-the-art novel view synthesis quality. Notably, our models surpass all previous methods even with reduced computational resources (1-2 GPUs). Please see our website for more details: https://haian-jin.github.io/projects/LVSM/ .