M-Longdoc: A Benchmark For Multimodal Super-Long Document Understanding And A Retrieval-Aware Tuning Framework
Yew Ken Chia, Liying Cheng, Hou Pong Chan, Chaoqun Liu, Maojia Song, Sharifah Mahani Aljunied, Soujanya Poria, Lidong Bing
2024-11-12
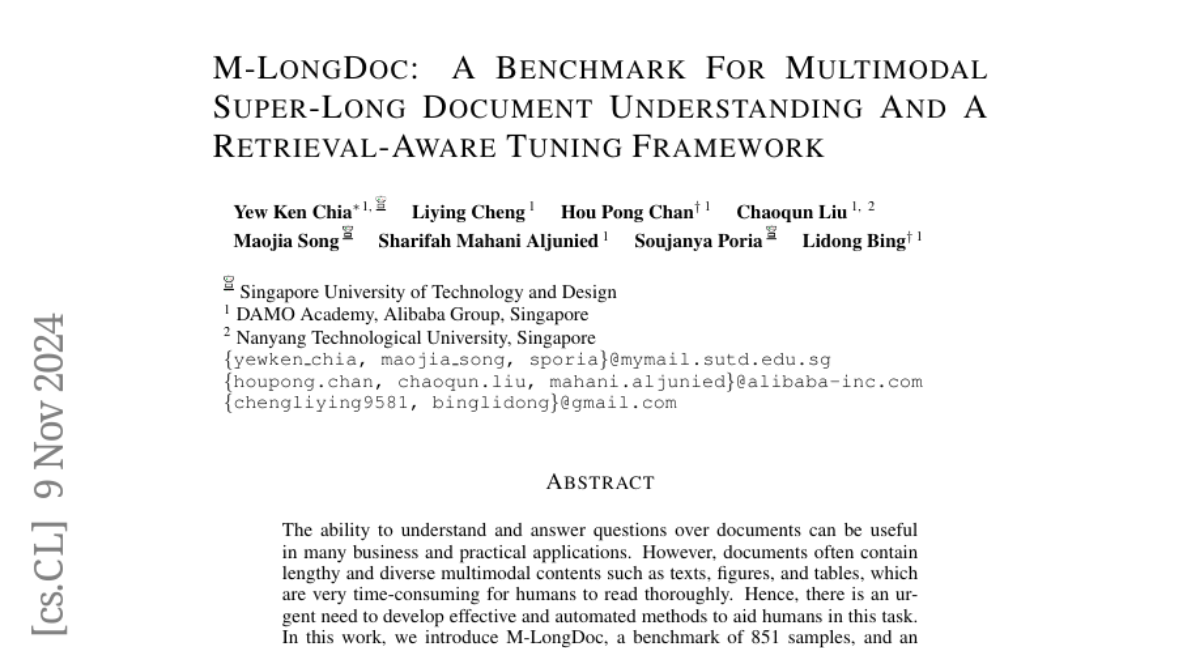
Summary
This paper introduces M-LongDoc, a new benchmark and framework designed to help large models understand and answer questions about very long documents that include text, figures, and tables.
What's the problem?
Understanding lengthy documents can be difficult and time-consuming for humans, especially when these documents contain a mix of different types of information. Current methods for processing such documents are not efficient enough, and there is a need for automated systems that can quickly and accurately extract relevant information from these complex materials.
What's the solution?
M-LongDoc consists of a benchmark with 851 samples of recent and lengthy documents, along with a new automated framework that helps evaluate how well multimodal models perform on these tasks. The researchers also developed a retrieval-aware tuning approach that improves how models read and understand long documents. This framework allows for open-ended questions, meaning the models can provide detailed answers rather than just pulling out specific pieces of information. The authors created a training dataset automatically to help models learn to answer questions based on the structure of the long documents.
Why it matters?
This research is significant because it provides tools to improve how AI systems interact with complex documents. By enhancing the ability of models to process and understand long texts, this work can lead to better applications in fields like business, law, and education, where quick access to information is crucial.
Abstract
The ability to understand and answer questions over documents can be useful in many business and practical applications. However, documents often contain lengthy and diverse multimodal contents such as texts, figures, and tables, which are very time-consuming for humans to read thoroughly. Hence, there is an urgent need to develop effective and automated methods to aid humans in this task. In this work, we introduce M-LongDoc, a benchmark of 851 samples, and an automated framework to evaluate the performance of large multimodal models. We further propose a retrieval-aware tuning approach for efficient and effective multimodal document reading. Compared to existing works, our benchmark consists of more recent and lengthy documents with hundreds of pages, while also requiring open-ended solutions and not just extractive answers. To our knowledge, our training framework is the first to directly address the retrieval setting for multimodal long documents. To enable tuning open-source models, we construct a training corpus in a fully automatic manner for the question-answering task over such documents. Experiments show that our tuning approach achieves a relative improvement of 4.6% for the correctness of model responses, compared to the baseline open-source models. Our data, code, and models are available at https://multimodal-documents.github.io.