Magpie: Alignment Data Synthesis from Scratch by Prompting Aligned LLMs with Nothing
Zhangchen Xu, Fengqing Jiang, Luyao Niu, Yuntian Deng, Radha Poovendran, Yejin Choi, Bill Yuchen Lin
2024-06-13
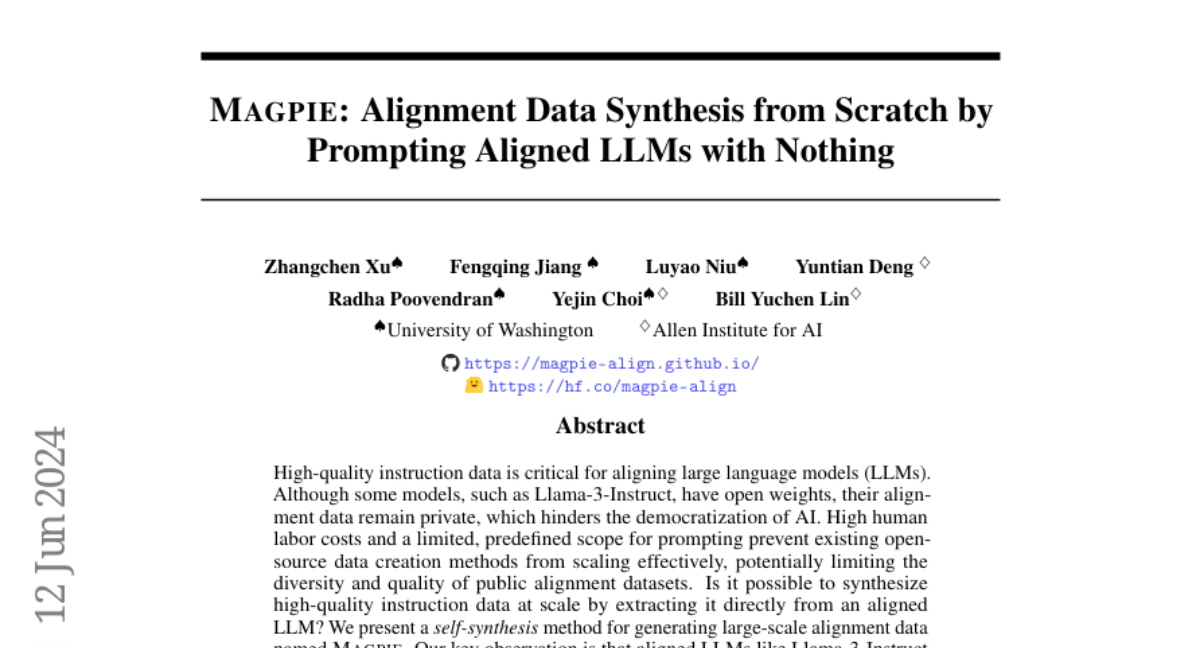
Summary
This paper introduces Magpie, a new method for creating high-quality instruction data using large language models (LLMs) like Llama-3. It focuses on generating this data efficiently and at scale without needing extensive human input.
What's the problem?
High-quality instruction data is essential for training AI models to understand and follow instructions. However, many existing methods for creating this data are limited because they require a lot of human effort and often don't produce diverse datasets. Additionally, while some models have open weights, their training data is still private, making it hard for researchers to access and use.
What's the solution?
The authors developed Magpie, a self-synthesis method that allows LLMs to generate instruction data by using only simple templates. They prompted the Llama-3 model to create 4 million instructions and their responses. After analyzing the generated data, they selected 300,000 high-quality examples. They then tested how well models trained on Magpie's data performed compared to those trained on other datasets, finding that Magpie's results were competitive.
Why it matters?
Magpie is significant because it provides a scalable way to generate diverse and high-quality instruction data without heavy reliance on human labor. This can help democratize AI by making it easier for researchers to develop and improve AI systems, ultimately leading to better AI assistants that can understand and execute complex tasks.
Abstract
High-quality instruction data is critical for aligning large language models (LLMs). Although some models, such as Llama-3-Instruct, have open weights, their alignment data remain private, which hinders the democratization of AI. High human labor costs and a limited, predefined scope for prompting prevent existing open-source data creation methods from scaling effectively, potentially limiting the diversity and quality of public alignment datasets. Is it possible to synthesize high-quality instruction data at scale by extracting it directly from an aligned LLM? We present a self-synthesis method for generating large-scale alignment data named Magpie. Our key observation is that aligned LLMs like Llama-3-Instruct can generate a user query when we input only the left-side templates up to the position reserved for user messages, thanks to their auto-regressive nature. We use this method to prompt Llama-3-Instruct and generate 4 million instructions along with their corresponding responses. We perform a comprehensive analysis of the extracted data and select 300K high-quality instances. To compare Magpie data with other public instruction datasets, we fine-tune Llama-3-8B-Base with each dataset and evaluate the performance of the fine-tuned models. Our results indicate that in some tasks, models fine-tuned with Magpie perform comparably to the official Llama-3-8B-Instruct, despite the latter being enhanced with 10 million data points through supervised fine-tuning (SFT) and subsequent feedback learning. We also show that using Magpie solely for SFT can surpass the performance of previous public datasets utilized for both SFT and preference optimization, such as direct preference optimization with UltraFeedback. This advantage is evident on alignment benchmarks such as AlpacaEval, ArenaHard, and WildBench.