Making Text Embedders Few-Shot Learners
Chaofan Li, MingHao Qin, Shitao Xiao, Jianlyu Chen, Kun Luo, Yingxia Shao, Defu Lian, Zheng Liu
2024-09-25
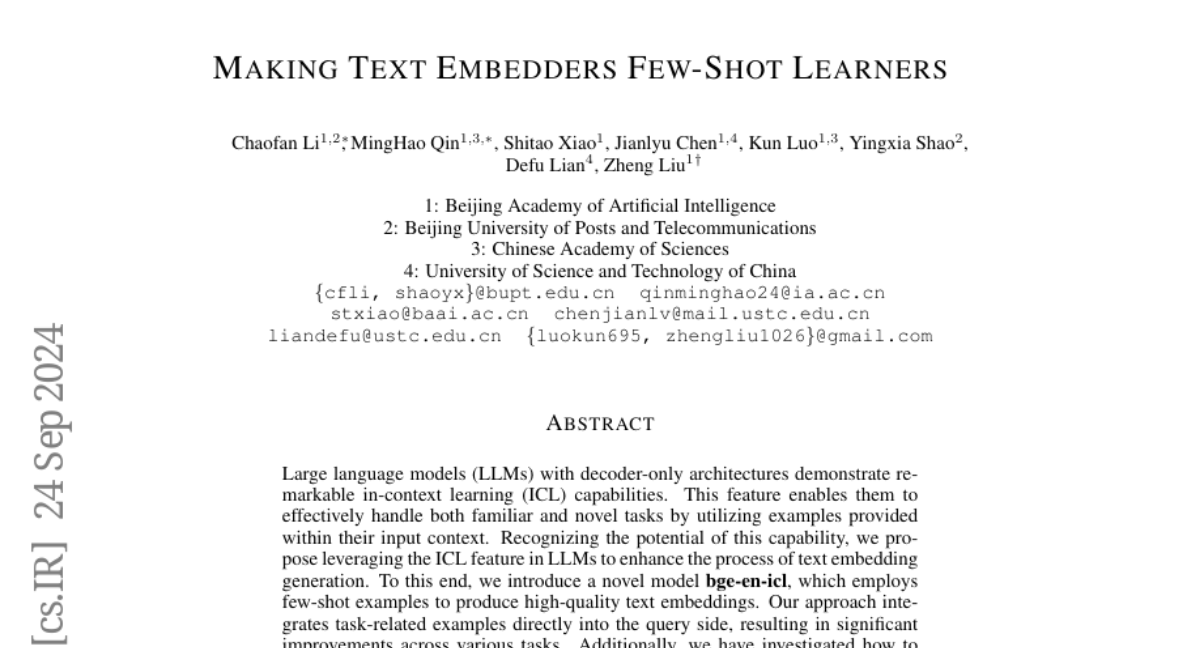
Summary
This paper discusses a new model called bge-en-icl, which improves how large language models (LLMs) create text embeddings. It uses few-shot learning, meaning it learns from a small number of examples to generate high-quality text representations.
What's the problem?
While large language models are great at understanding and generating text, they often struggle when it comes to creating text embeddings—compact representations of text that capture its meaning. Existing methods don't effectively leverage the in-context learning (ICL) capabilities of these models, making it hard for them to adapt to new tasks or instructions without extensive training.
What's the solution?
To tackle this issue, the researchers introduced bge-en-icl, a model that incorporates few-shot examples directly into the input queries. This allows the model to learn from just a few examples while generating embeddings. They also explored different techniques for using LLMs as embedding models, including attention mechanisms and pooling methods. Their experiments showed that keeping the approach simple and using ICL effectively led to better performance on various benchmarks, setting new standards in the field.
Why it matters?
This research is significant because it enhances the ability of language models to produce useful text embeddings with minimal training data. This improvement can benefit many applications, such as search engines, recommendation systems, and any technology that relies on understanding and processing language effectively. By making these models more adaptable and efficient, it can lead to better user experiences in various AI-driven tools.
Abstract
Large language models (LLMs) with decoder-only architectures demonstrate remarkable in-context learning (ICL) capabilities. This feature enables them to effectively handle both familiar and novel tasks by utilizing examples provided within their input context. Recognizing the potential of this capability, we propose leveraging the ICL feature in LLMs to enhance the process of text embedding generation. To this end, we introduce a novel model bge-en-icl, which employs few-shot examples to produce high-quality text embeddings. Our approach integrates task-related examples directly into the query side, resulting in significant improvements across various tasks. Additionally, we have investigated how to effectively utilize LLMs as embedding models, including various attention mechanisms, pooling methods, etc. Our findings suggest that retaining the original framework often yields the best results, underscoring that simplicity is best. Experimental results on the MTEB and AIR-Bench benchmarks demonstrate that our approach sets new state-of-the-art (SOTA) performance. Our model, code and dataset are freely available at https://github.com/FlagOpen/FlagEmbedding .