MambaEVT: Event Stream based Visual Object Tracking using State Space Model
Xiao Wang, Chao wang, Shiao Wang, Xixi Wang, Zhicheng Zhao, Lin Zhu, Bo Jiang
2024-08-21
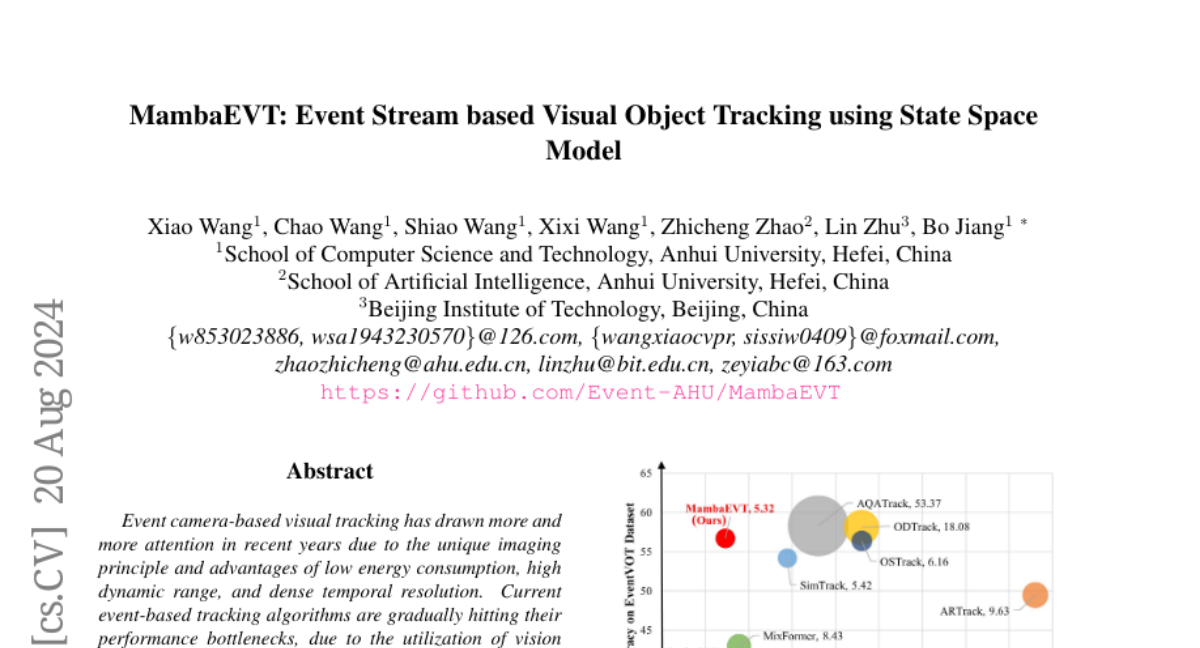
Summary
This paper introduces MambaEVT, a new method for tracking objects in videos using event cameras, which are special cameras that capture changes in a scene very quickly.
What's the problem?
Current methods for tracking objects in videos often struggle when using event cameras because they rely on static templates and can become slow and less effective. This limits their ability to accurately follow fast-moving objects or handle complex scenes.
What's the solution?
MambaEVT uses a state space model to improve the tracking process. It efficiently extracts features from both the search areas and the target objects simultaneously. Additionally, it incorporates a dynamic template update strategy, which allows the system to adjust its tracking template based on the current scene. This combination helps maintain accuracy while reducing computational costs.
Why it matters?
This research is important because it enhances the ability to track objects in real-time using advanced camera technology. By improving how we track objects, MambaEVT can be applied in various fields such as robotics, autonomous vehicles, and surveillance, where accurate and fast tracking is essential.
Abstract
Event camera-based visual tracking has drawn more and more attention in recent years due to the unique imaging principle and advantages of low energy consumption, high dynamic range, and dense temporal resolution. Current event-based tracking algorithms are gradually hitting their performance bottlenecks, due to the utilization of vision Transformer and the static template for target object localization. In this paper, we propose a novel Mamba-based visual tracking framework that adopts the state space model with linear complexity as a backbone network. The search regions and target template are fed into the vision Mamba network for simultaneous feature extraction and interaction. The output tokens of search regions will be fed into the tracking head for target localization. More importantly, we consider introducing a dynamic template update strategy into the tracking framework using the Memory Mamba network. By considering the diversity of samples in the target template library and making appropriate adjustments to the template memory module, a more effective dynamic template can be integrated. The effective combination of dynamic and static templates allows our Mamba-based tracking algorithm to achieve a good balance between accuracy and computational cost on multiple large-scale datasets, including EventVOT, VisEvent, and FE240hz. The source code will be released on https://github.com/Event-AHU/MambaEVT