Marco-o1: Towards Open Reasoning Models for Open-Ended Solutions
Yu Zhao, Huifeng Yin, Bo Zeng, Hao Wang, Tianqi Shi, Chenyang Lyu, Longyue Wang, Weihua Luo, Kaifu Zhang
2024-11-22
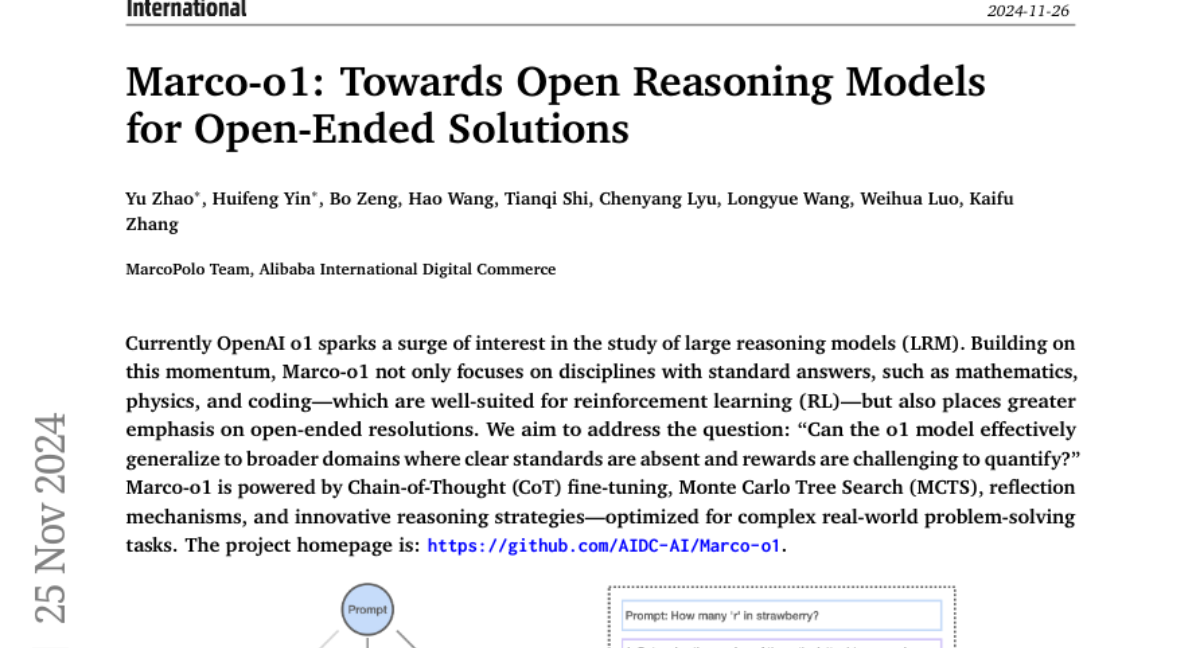
Summary
This paper introduces Marco-o1, a new type of reasoning model that aims to improve how AI systems handle complex, open-ended problems, going beyond traditional subjects like math and coding.
What's the problem?
Current reasoning models often struggle with tasks that don't have clear or standard answers. They excel in structured areas like mathematics but find it challenging to generalize their skills to more ambiguous situations where solutions are not straightforward. This limits their effectiveness in real-world applications where problems can be complex and open-ended.
What's the solution?
Marco-o1 enhances reasoning capabilities by using advanced techniques like Chain-of-Thought (CoT) fine-tuning and Monte Carlo Tree Search (MCTS). CoT helps the model break down problems into smaller steps, making it easier to think through complex issues. MCTS allows the model to explore different possible actions and outcomes, simulating various paths to find the best solution. This combination enables Marco-o1 to tackle a wider range of problems effectively, even those without clear answers.
Why it matters?
This research is important because it pushes the boundaries of what AI can do in terms of reasoning and problem-solving. By developing models that can handle open-ended questions and ambiguous situations, Marco-o1 has the potential to improve AI applications in areas like natural language processing, decision-making, and strategic planning, making AI systems more versatile and useful in everyday scenarios.
Abstract
Currently OpenAI o1 has sparked a surge of interest in the study of large reasoning models (LRM). Building on this momentum, Marco-o1 not only focuses on disciplines with standard answers, such as mathematics, physics, and coding -- which are well-suited for reinforcement learning (RL) -- but also places greater emphasis on open-ended resolutions. We aim to address the question: "Can the o1 model effectively generalize to broader domains where clear standards are absent and rewards are challenging to quantify?" Marco-o1 is powered by Chain-of-Thought (CoT) fine-tuning, Monte Carlo Tree Search (MCTS), reflection mechanisms, and innovative reasoning strategies -- optimized for complex real-world problem-solving tasks.