MarDini: Masked Autoregressive Diffusion for Video Generation at Scale
Haozhe Liu, Shikun Liu, Zijian Zhou, Mengmeng Xu, Yanping Xie, Xiao Han, Juan C. Pérez, Ding Liu, Kumara Kahatapitiya, Menglin Jia, Jui-Chieh Wu, Sen He, Tao Xiang, Jürgen Schmidhuber, Juan-Manuel Pérez-Rúa
2024-10-29
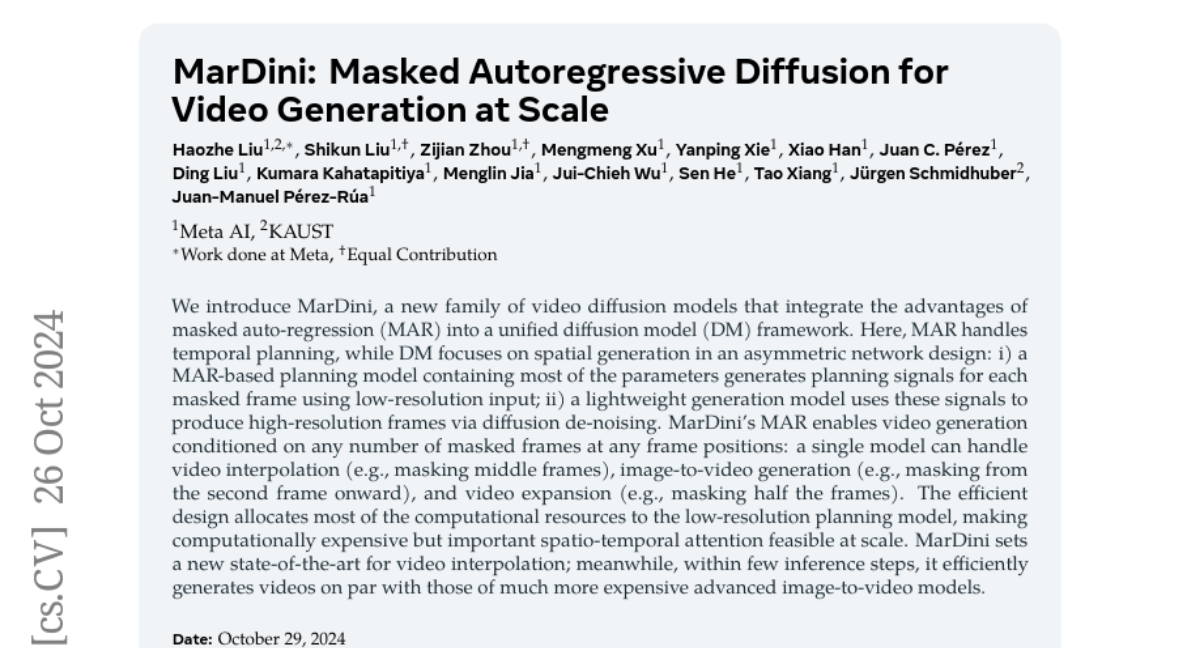
Summary
This paper introduces MarDini, a new type of video generation model that combines different techniques to create high-quality videos efficiently.
What's the problem?
Creating videos using AI can be challenging because it requires understanding both the timing of actions (temporal planning) and the visual details (spatial generation). Existing models often struggle to balance these two aspects, leading to slower video generation and lower quality outputs.
What's the solution?
MarDini uses a unique approach that integrates masked autoregressive (MAR) techniques for planning the timing of video frames with a diffusion model (DM) for generating detailed visuals. The model is designed in two parts: one part focuses on planning using low-resolution inputs to generate signals for each frame, while the other part creates high-resolution frames based on those signals. This allows MarDini to handle various tasks like filling in missing frames or turning images into videos efficiently. The design prioritizes computational resources for planning, making it faster and more effective than previous models.
Why it matters?
This research is significant because it sets a new standard for video generation technology. By improving how AI models create videos, MarDini can be used in various applications, from film production to video game development, enhancing creativity and productivity in these fields.
Abstract
We introduce MarDini, a new family of video diffusion models that integrate the advantages of masked auto-regression (MAR) into a unified diffusion model (DM) framework. Here, MAR handles temporal planning, while DM focuses on spatial generation in an asymmetric network design: i) a MAR-based planning model containing most of the parameters generates planning signals for each masked frame using low-resolution input; ii) a lightweight generation model uses these signals to produce high-resolution frames via diffusion de-noising. MarDini's MAR enables video generation conditioned on any number of masked frames at any frame positions: a single model can handle video interpolation (e.g., masking middle frames), image-to-video generation (e.g., masking from the second frame onward), and video expansion (e.g., masking half the frames). The efficient design allocates most of the computational resources to the low-resolution planning model, making computationally expensive but important spatio-temporal attention feasible at scale. MarDini sets a new state-of-the-art for video interpolation; meanwhile, within few inference steps, it efficiently generates videos on par with those of much more expensive advanced image-to-video models.