Marigold-DC: Zero-Shot Monocular Depth Completion with Guided Diffusion
Massimiliano Viola, Kevin Qu, Nando Metzger, Bingxin Ke, Alexander Becker, Konrad Schindler, Anton Obukhov
2024-12-18
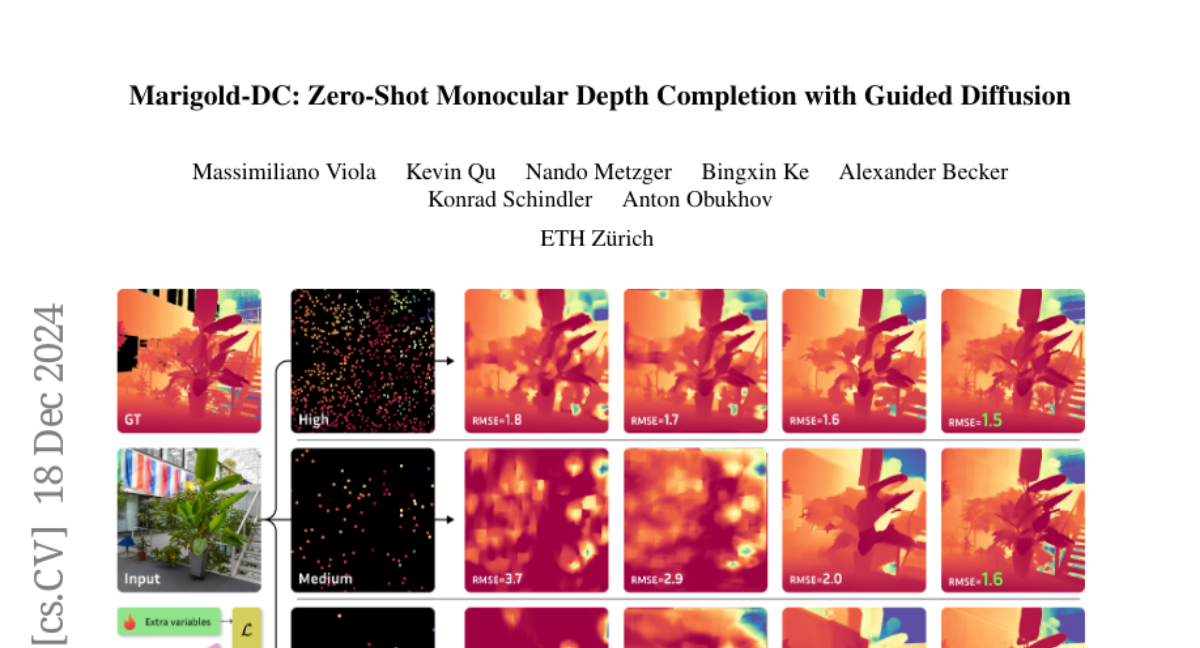
Summary
This paper talks about Marigold-DC, a new method for improving depth completion from sparse depth measurements using guided diffusion, allowing for better depth maps from single images.
What's the problem?
Depth completion is the process of turning incomplete or sparse depth data (like how far away objects are) into detailed depth maps. Traditional methods struggle when the depth measurements are irregular or when they come from images that the model hasn't seen before. This makes it difficult to accurately understand the 3D structure of scenes in real-world applications.
What's the solution?
Marigold-DC addresses these issues by using a technique called guided diffusion, which helps generate dense depth maps based on sparse measurements. It combines information from a conventional image with these sparse depth points to create a more complete picture. The method is designed to work well even without prior training on specific types of data, allowing it to generalize across different environments effectively.
Why it matters?
This research is important because it enhances the ability of AI systems to interpret and understand 3D spaces from limited data. By improving depth completion techniques, Marigold-DC can be applied in various fields such as robotics, virtual reality, and autonomous vehicles, where accurate depth perception is crucial for navigation and interaction with the environment.
Abstract
Depth completion upgrades sparse depth measurements into dense depth maps guided by a conventional image. Existing methods for this highly ill-posed task operate in tightly constrained settings and tend to struggle when applied to images outside the training domain or when the available depth measurements are sparse, irregularly distributed, or of varying density. Inspired by recent advances in monocular depth estimation, we reframe depth completion as an image-conditional depth map generation guided by sparse measurements. Our method, Marigold-DC, builds on a pretrained latent diffusion model for monocular depth estimation and injects the depth observations as test-time guidance via an optimization scheme that runs in tandem with the iterative inference of denoising diffusion. The method exhibits excellent zero-shot generalization across a diverse range of environments and handles even extremely sparse guidance effectively. Our results suggest that contemporary monocular depth priors greatly robustify depth completion: it may be better to view the task as recovering dense depth from (dense) image pixels, guided by sparse depth; rather than as inpainting (sparse) depth, guided by an image. Project website: https://MarigoldDepthCompletion.github.io/