MaskBit: Embedding-free Image Generation via Bit Tokens
Mark Weber, Lijun Yu, Qihang Yu, Xueqing Deng, Xiaohui Shen, Daniel Cremers, Liang-Chieh Chen
2024-09-25
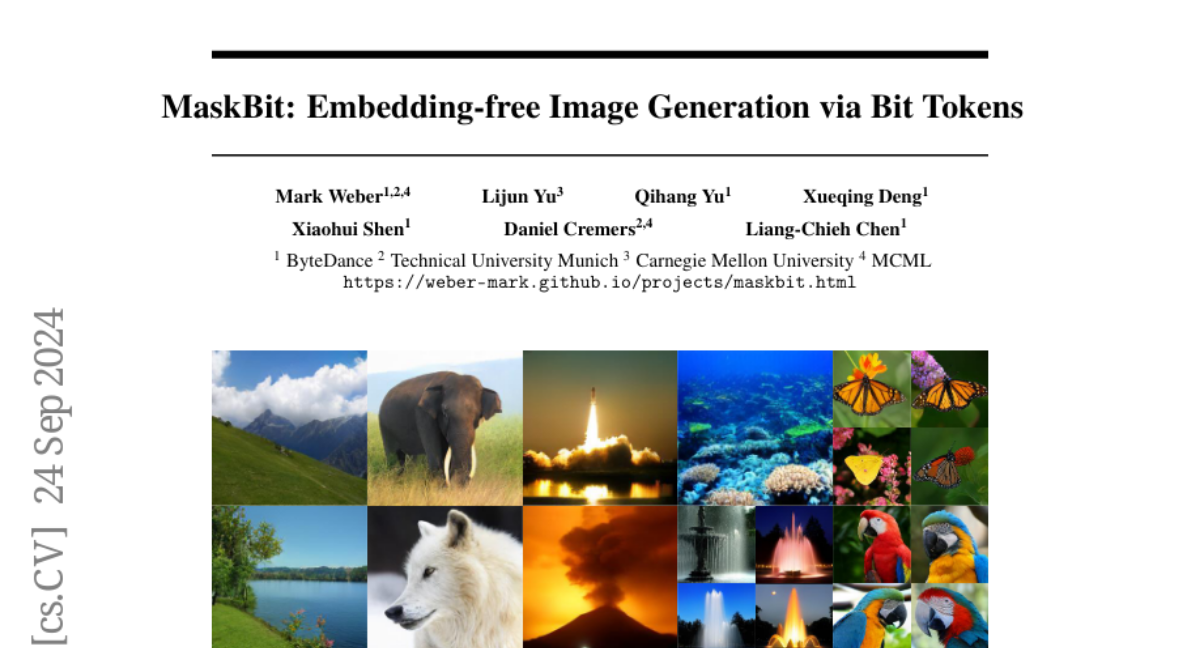
Summary
This paper introduces MaskBit, a new method for generating images that doesn't rely on traditional embedding techniques. Instead, it uses bit tokens, which are a compact binary representation of image features, to create high-quality images efficiently.
What's the problem?
Most current image generation methods use complex models that require a lot of resources and time to learn how to represent images. These methods often involve two main stages: first converting images into a simpler form (latent space) and then generating the final image from that form. This process can be slow and may not always produce the best results.
What's the solution?
To improve this process, the researchers developed MaskBit, which simplifies image generation by using bit tokens instead of traditional embeddings. Bit tokens are binary representations that capture important features of images in a compact way. The researchers also modernized the VQGAN model, which is commonly used in these tasks, making it more efficient and effective. Their experiments showed that MaskBit achieved a new state-of-the-art performance on the ImageNet benchmark, producing high-quality images with a smaller model size of only 305 million parameters.
Why it matters?
This research is significant because it offers a more efficient way to generate images without sacrificing quality. By using bit tokens, MaskBit can produce images faster and with less computational power than traditional methods. This advancement could lead to improvements in various applications such as video games, movies, and virtual reality, where high-quality images are essential.
Abstract
Masked transformer models for class-conditional image generation have become a compelling alternative to diffusion models. Typically comprising two stages - an initial VQGAN model for transitioning between latent space and image space, and a subsequent Transformer model for image generation within latent space - these frameworks offer promising avenues for image synthesis. In this study, we present two primary contributions: Firstly, an empirical and systematic examination of VQGANs, leading to a modernized VQGAN. Secondly, a novel embedding-free generation network operating directly on bit tokens - a binary quantized representation of tokens with rich semantics. The first contribution furnishes a transparent, reproducible, and high-performing VQGAN model, enhancing accessibility and matching the performance of current state-of-the-art methods while revealing previously undisclosed details. The second contribution demonstrates that embedding-free image generation using bit tokens achieves a new state-of-the-art FID of 1.52 on the ImageNet 256x256 benchmark, with a compact generator model of mere 305M parameters.