Masked Generative Video-to-Audio Transformers with Enhanced Synchronicity
Santiago Pascual, Chunghsin Yeh, Ioannis Tsiamas, Joan Serrà
2024-07-16
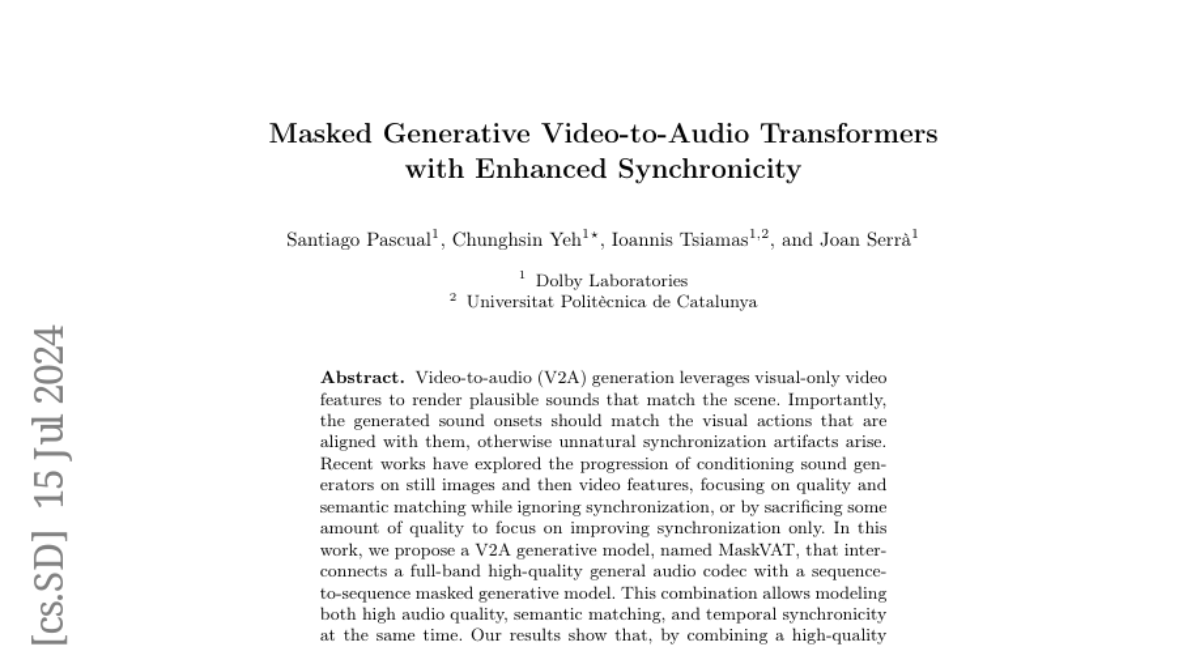
Summary
This paper introduces MaskVAT, a new model designed to generate audio from video while ensuring that the sounds match the actions happening in the video closely.
What's the problem?
When creating sounds to match videos, it’s crucial that the audio is synchronized with the visual actions. If the sounds don’t align well with what’s happening on screen, it can feel unnatural and distracting. Previous methods either focused on making high-quality sounds or on improving synchronization but often compromised on one aspect or the other.
What's the solution?
MaskVAT combines a high-quality audio codec with a masked generative model to generate audio that is both high-quality and well-synchronized with the video. It uses a technique where it learns to predict missing parts of audio based on the video frames. This allows it to generate sounds that accurately reflect what is happening in the video, maintaining both quality and synchronicity. The model has been trained to handle various tasks and shows strong performance in generating synchronized audio for different types of videos.
Why it matters?
This research is important because it enhances how we can create sound for videos, which is essential for applications like film production, animation, and video games. By improving synchronization between audio and visual elements, MaskVAT can help create more immersive and engaging experiences for viewers.
Abstract
Video-to-audio (V2A) generation leverages visual-only video features to render plausible sounds that match the scene. Importantly, the generated sound onsets should match the visual actions that are aligned with them, otherwise unnatural synchronization artifacts arise. Recent works have explored the progression of conditioning sound generators on still images and then video features, focusing on quality and semantic matching while ignoring synchronization, or by sacrificing some amount of quality to focus on improving synchronization only. In this work, we propose a V2A generative model, named MaskVAT, that interconnects a full-band high-quality general audio codec with a sequence-to-sequence masked generative model. This combination allows modeling both high audio quality, semantic matching, and temporal synchronicity at the same time. Our results show that, by combining a high-quality codec with the proper pre-trained audio-visual features and a sequence-to-sequence parallel structure, we are able to yield highly synchronized results on one hand, whilst being competitive with the state of the art of non-codec generative audio models. Sample videos and generated audios are available at https://maskvat.github.io .