MaskedMimic: Unified Physics-Based Character Control Through Masked Motion Inpainting
Chen Tessler, Yunrong Guo, Ofir Nabati, Gal Chechik, Xue Bin Peng
2024-09-24
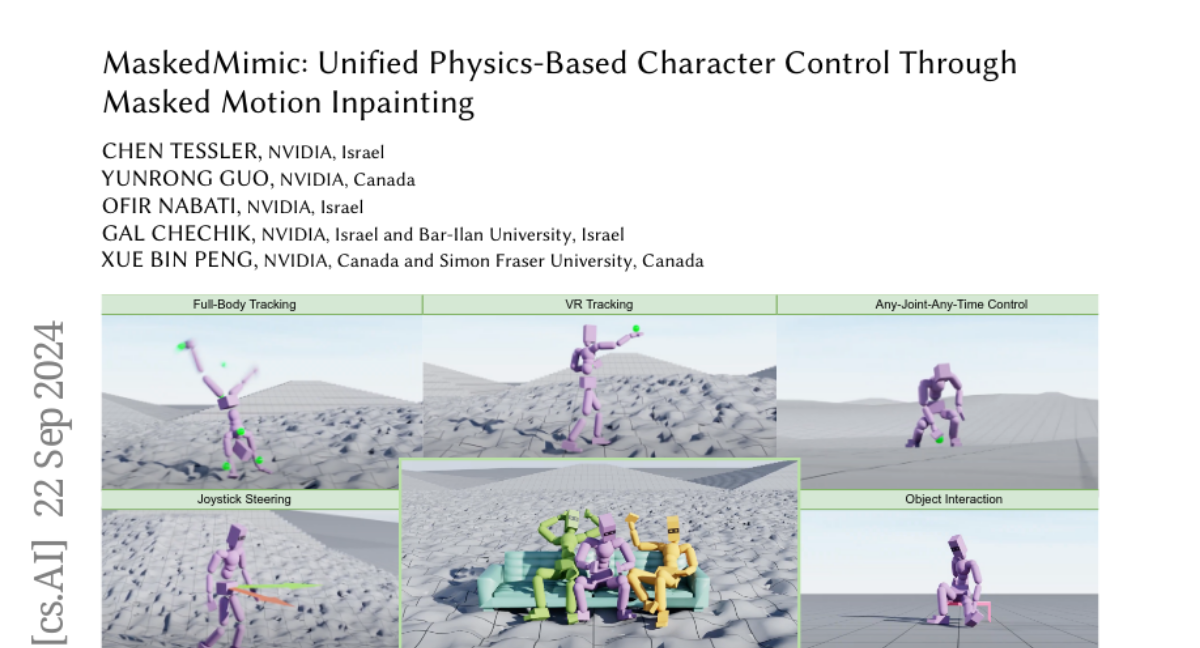
Summary
This paper introduces MaskedMimic, a new system that allows for advanced control of animated characters in a realistic way. It uses a technique called motion inpainting to create smooth and believable movements based on partial information about what the character should do.
What's the problem?
Creating realistic animations for characters that can respond to different commands and environments is challenging. Most existing systems are limited because they only work well for specific tasks and require a lot of detailed input. This makes it hard to create versatile characters that can adapt to various situations without needing extensive programming or adjustments.
What's the solution?
MaskedMimic solves this problem by treating character control as a motion inpainting task. This means it can generate complete motions from incomplete information, like partial keyframes or simple text instructions. The system uses a single model trained on a wide range of motion data, allowing it to produce realistic animations that fit different scenarios. For example, it can generate full-body movements based on just the positions of a few joints or respond to user commands in real-time.
Why it matters?
This research is significant because it makes character animation more flexible and intuitive. By allowing characters to be controlled through simple inputs, MaskedMimic can enhance video games, virtual reality experiences, and other interactive applications. This opens up new possibilities for creating engaging and lifelike characters that can easily adapt to users' needs.
Abstract
Crafting a single, versatile physics-based controller that can breathe life into interactive characters across a wide spectrum of scenarios represents an exciting frontier in character animation. An ideal controller should support diverse control modalities, such as sparse target keyframes, text instructions, and scene information. While previous works have proposed physically simulated, scene-aware control models, these systems have predominantly focused on developing controllers that each specializes in a narrow set of tasks and control modalities. This work presents MaskedMimic, a novel approach that formulates physics-based character control as a general motion inpainting problem. Our key insight is to train a single unified model to synthesize motions from partial (masked) motion descriptions, such as masked keyframes, objects, text descriptions, or any combination thereof. This is achieved by leveraging motion tracking data and designing a scalable training method that can effectively utilize diverse motion descriptions to produce coherent animations. Through this process, our approach learns a physics-based controller that provides an intuitive control interface without requiring tedious reward engineering for all behaviors of interest. The resulting controller supports a wide range of control modalities and enables seamless transitions between disparate tasks. By unifying character control through motion inpainting, MaskedMimic creates versatile virtual characters. These characters can dynamically adapt to complex scenes and compose diverse motions on demand, enabling more interactive and immersive experiences.