MaskLLM: Learnable Semi-Structured Sparsity for Large Language Models
Gongfan Fang, Hongxu Yin, Saurav Muralidharan, Greg Heinrich, Jeff Pool, Jan Kautz, Pavlo Molchanov, Xinchao Wang
2024-09-27
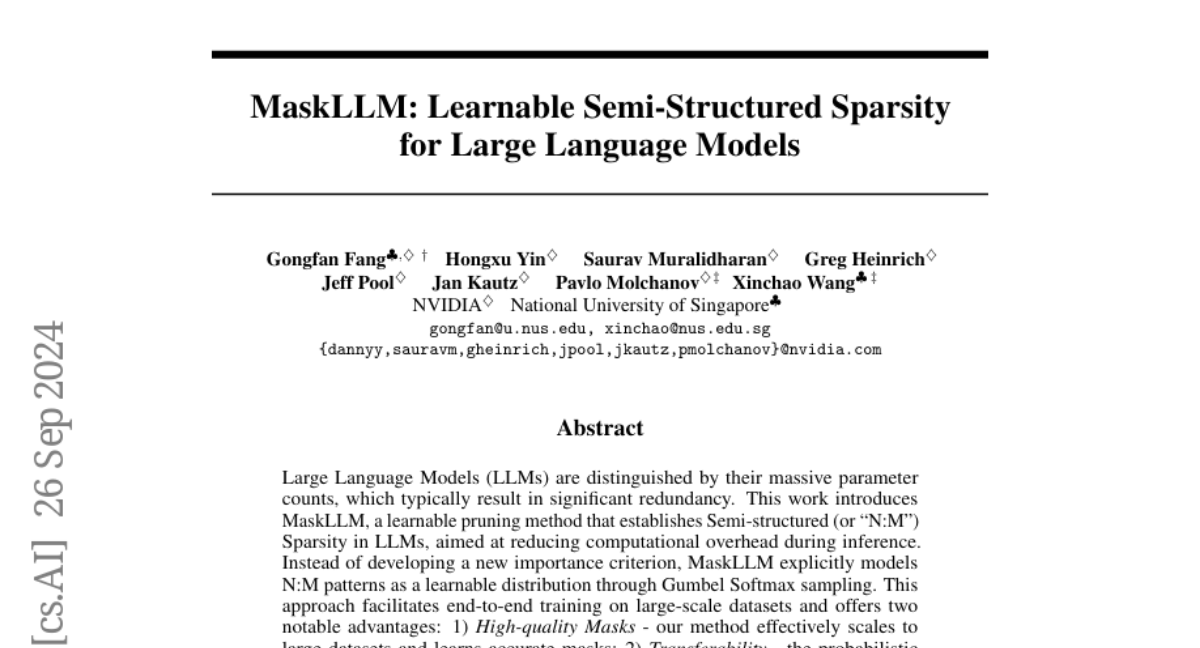
Summary
This paper talks about MaskLLM, a new method designed to make large language models (LLMs) more efficient by using a technique called learnable semi-structured sparsity. This approach helps reduce the amount of computational power needed while maintaining the model's performance.
What's the problem?
Large language models are powerful but often have a lot of extra parameters that can slow down their performance and require more resources to run. Traditional methods for improving efficiency usually involve removing unnecessary parts of the model, but they can be complex and not very effective. This leads to challenges in making LLMs faster and less resource-intensive without losing their accuracy.
What's the solution?
MaskLLM introduces a method that prunes (removes) parts of the model in a smart way. Instead of just cutting out random parameters, it uses a learnable process that identifies which parts of the model can be removed while keeping the important ones. The method employs something called Gumbel Softmax sampling to create flexible patterns for pruning, allowing the model to learn which parameters are less important during training. This way, MaskLLM can efficiently reduce the size of the model while still performing well on tasks.
Why it matters?
This research is significant because it provides a way to make large language models more efficient, which is crucial as these models become more widely used in various applications like chatbots, translation services, and content generation. By reducing the computational load, MaskLLM can help lower costs and make it easier to deploy these powerful models in real-world scenarios.
Abstract
Large Language Models (LLMs) are distinguished by their massive parameter counts, which typically result in significant redundancy. This work introduces MaskLLM, a learnable pruning method that establishes Semi-structured (or ``N:M'') Sparsity in LLMs, aimed at reducing computational overhead during inference. Instead of developing a new importance criterion, MaskLLM explicitly models N:M patterns as a learnable distribution through Gumbel Softmax sampling. This approach facilitates end-to-end training on large-scale datasets and offers two notable advantages: 1) High-quality Masks - our method effectively scales to large datasets and learns accurate masks; 2) Transferability - the probabilistic modeling of mask distribution enables the transfer learning of sparsity across domains or tasks. We assessed MaskLLM using 2:4 sparsity on various LLMs, including LLaMA-2, Nemotron-4, and GPT-3, with sizes ranging from 843M to 15B parameters, and our empirical results show substantial improvements over state-of-the-art methods. For instance, leading approaches achieve a perplexity (PPL) of 10 or greater on Wikitext compared to the dense model's 5.12 PPL, but MaskLLM achieves a significantly lower 6.72 PPL solely by learning the masks with frozen weights. Furthermore, MaskLLM's learnable nature allows customized masks for lossless application of 2:4 sparsity to downstream tasks or domains. Code is available at https://github.com/NVlabs/MaskLLM.