MatchTime: Towards Automatic Soccer Game Commentary Generation
Jiayuan Rao, Haoning Wu, Chang Liu, Yanfeng Wang, Weidi Xie
2024-06-27
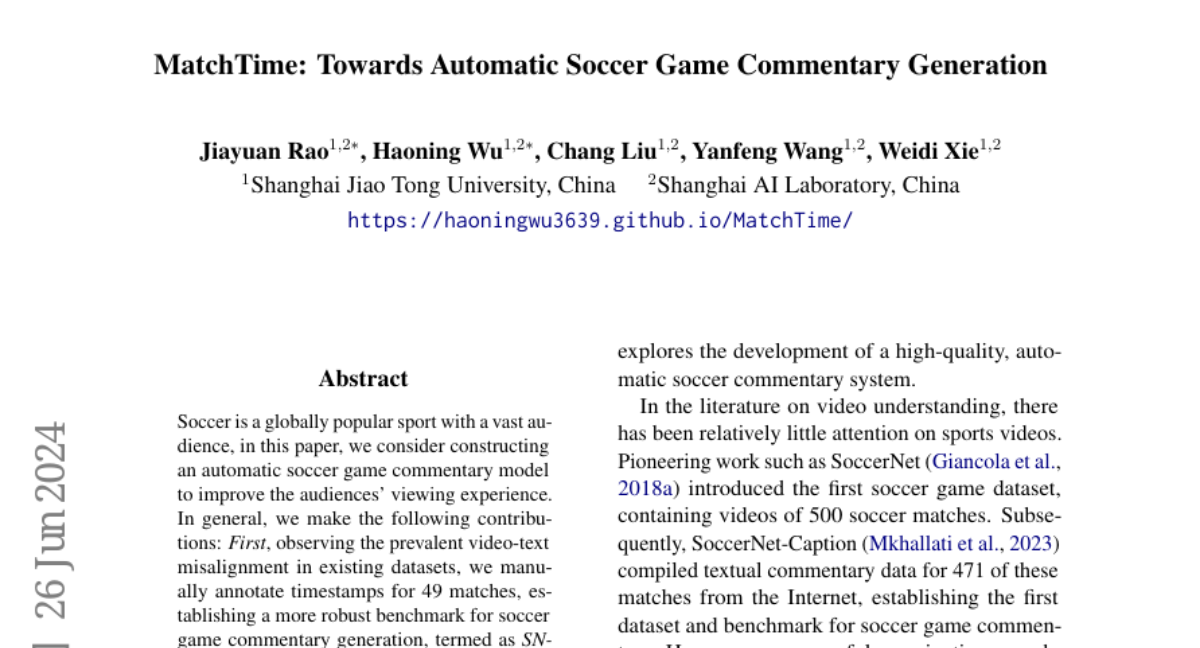
Summary
This paper introduces MatchTime, a system designed to automatically generate commentary for soccer games. It aims to enhance the viewing experience for fans by providing accurate and engaging commentary during matches.
What's the problem?
Many existing datasets used to train AI models for generating soccer commentary have issues with video and text not matching up properly. This misalignment can lead to poor-quality commentary that doesn't accurately reflect what is happening in the game. Without a reliable way to evaluate and improve these models, the generated commentary can be confusing or uninformative.
What's the solution?
To solve this problem, the authors first manually corrected the timestamps for 49 soccer matches, creating a new benchmark called SN-Caption-test-align. They then developed a multi-modal temporal alignment pipeline that automatically improves and filters existing datasets, resulting in a higher-quality training dataset named MatchTime. Finally, they trained an automatic commentary generation model called MatchVoice using this curated dataset. Their experiments showed that better alignment leads to significant improvements in how well the model generates commentary.
Why it matters?
This research is important because it addresses the challenges of creating high-quality automated sports commentary, which can enhance the experience for viewers. By improving how AI understands and describes soccer games, this work can lead to better broadcasting tools, making sports more enjoyable and accessible for fans around the world.
Abstract
Soccer is a globally popular sport with a vast audience, in this paper, we consider constructing an automatic soccer game commentary model to improve the audiences' viewing experience. In general, we make the following contributions: First, observing the prevalent video-text misalignment in existing datasets, we manually annotate timestamps for 49 matches, establishing a more robust benchmark for soccer game commentary generation, termed as SN-Caption-test-align; Second, we propose a multi-modal temporal alignment pipeline to automatically correct and filter the existing dataset at scale, creating a higher-quality soccer game commentary dataset for training, denoted as MatchTime; Third, based on our curated dataset, we train an automatic commentary generation model, named MatchVoice. Extensive experiments and ablation studies have demonstrated the effectiveness of our alignment pipeline, and training model on the curated datasets achieves state-of-the-art performance for commentary generation, showcasing that better alignment can lead to significant performance improvements in downstream tasks.