Measuring Human and AI Values based on Generative Psychometrics with Large Language Models
Haoran Ye, Yuhang Xie, Yuanyi Ren, Hanjun Fang, Xin Zhang, Guojie Song
2024-09-19
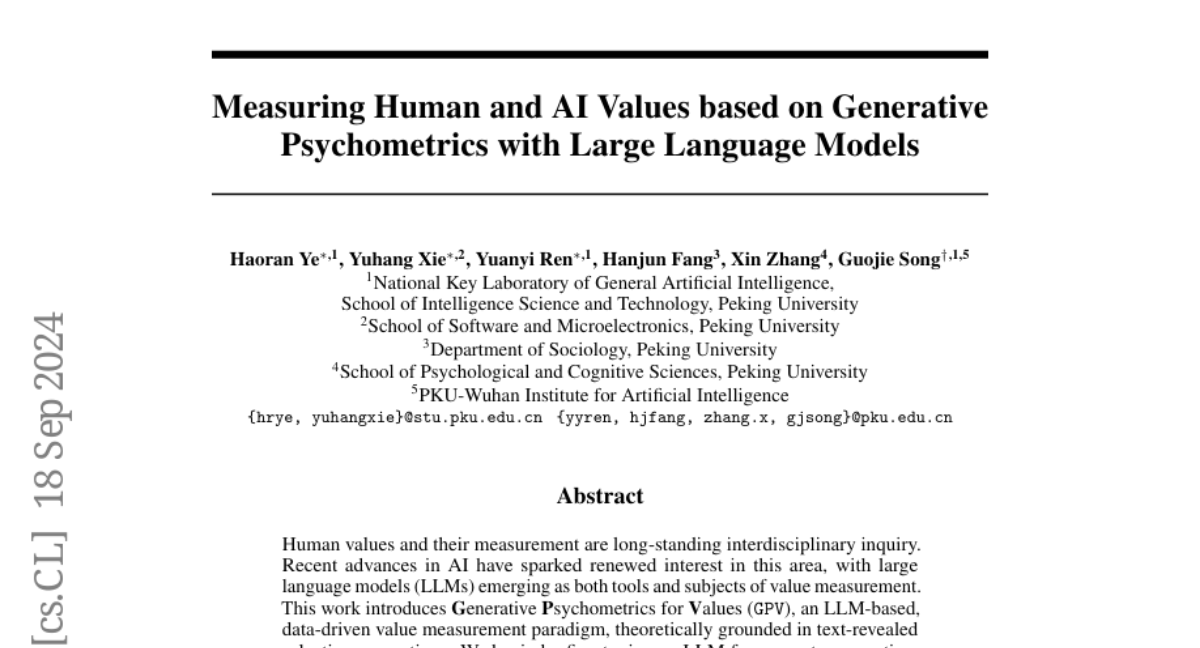
Summary
This paper introduces a new method called Generative Psychometrics for Values (GPV) that uses large language models (LLMs) to measure human and AI values based on the text they produce.
What's the problem?
Understanding and measuring human values has always been important, but traditional methods often rely on self-reports or surveys, which can be biased or not fully capture a person's true values. Additionally, as AI becomes more advanced, we need ways to measure the values of AI systems to ensure they align with human values.
What's the solution?
The authors developed GPV, which fine-tunes a large language model to accurately measure values by analyzing text. The model can break down text into specific perceptions (like beliefs or opinions) and then categorize these into broader value systems. They tested GPV on blogs written by people to show that it is stable and more effective than older psychological tools. They also extended this method to evaluate AI values based on the outputs generated by language models, highlighting how different value systems can affect AI safety.
Why it matters?
This research is significant because it provides a more reliable way to assess both human and AI values, which is crucial for developing AI systems that are safe and aligned with human ethics. By improving how we measure values, we can better understand how to create AI that supports positive outcomes in society.
Abstract
Human values and their measurement are long-standing interdisciplinary inquiry. Recent advances in AI have sparked renewed interest in this area, with large language models (LLMs) emerging as both tools and subjects of value measurement. This work introduces Generative Psychometrics for Values (GPV), an LLM-based, data-driven value measurement paradigm, theoretically grounded in text-revealed selective perceptions. We begin by fine-tuning an LLM for accurate perception-level value measurement and verifying the capability of LLMs to parse texts into perceptions, forming the core of the GPV pipeline. Applying GPV to human-authored blogs, we demonstrate its stability, validity, and superiority over prior psychological tools. Then, extending GPV to LLM value measurement, we advance the current art with 1) a psychometric methodology that measures LLM values based on their scalable and free-form outputs, enabling context-specific measurement; 2) a comparative analysis of measurement paradigms, indicating response biases of prior methods; and 3) an attempt to bridge LLM values and their safety, revealing the predictive power of different value systems and the impacts of various values on LLM safety. Through interdisciplinary efforts, we aim to leverage AI for next-generation psychometrics and psychometrics for value-aligned AI.