Medical SAM 2: Segment medical images as video via Segment Anything Model 2
Jiayuan Zhu, Yunli Qi, Junde Wu
2024-08-05
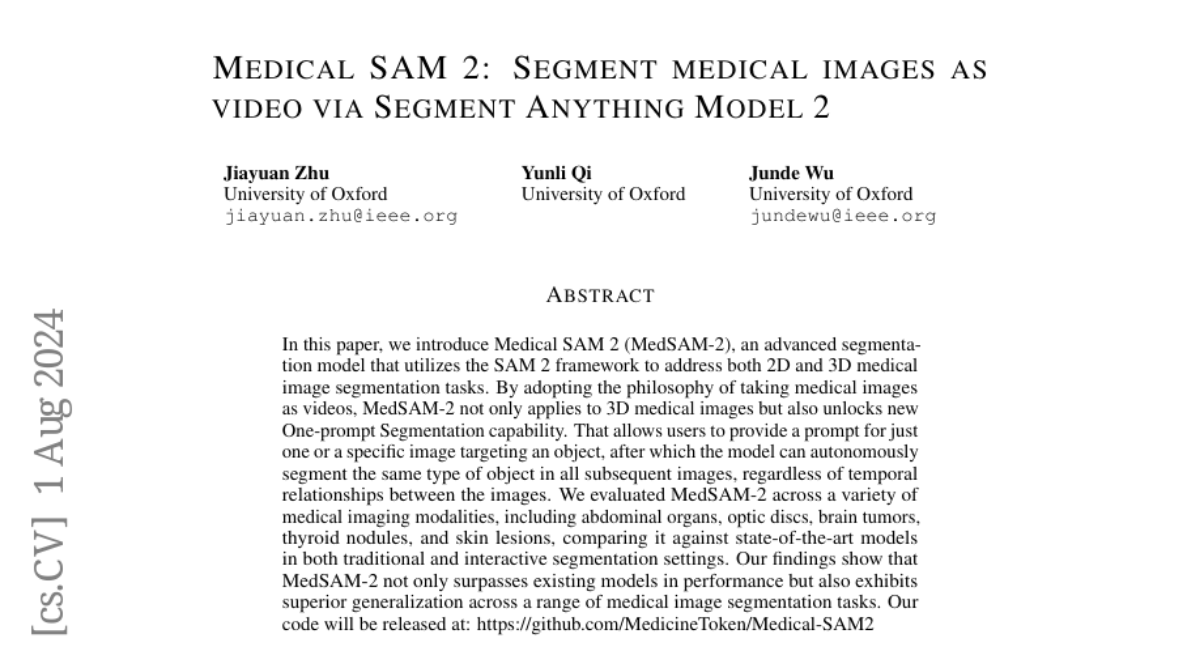
Summary
This paper introduces Medical SAM 2 (MedSAM-2), a new advanced model that helps segment medical images, treating them like videos to improve the accuracy and efficiency of identifying different parts of the images.
What's the problem?
Medical image segmentation is important for diagnosing and treating health conditions, but it can be challenging to accurately identify and separate different structures in both 2D and 3D images. Traditional methods may not effectively handle the complexities of medical images, leading to errors in diagnosis and treatment planning.
What's the solution?
MedSAM-2 uses the Segment Anything Model 2 framework to analyze medical images as if they were parts of a video. This allows users to provide a prompt for one image, and then the model can automatically segment similar objects in all following images without needing additional input. The authors tested MedSAM-2 on various types of medical images, including those of organs and tumors, and found that it outperformed existing models in both traditional and interactive settings.
Why it matters?
This research is significant because it enhances the ability of medical professionals to accurately analyze images quickly, which can lead to better patient outcomes. By improving how machines understand medical images, MedSAM-2 can help in areas like surgery planning, disease diagnosis, and monitoring treatment progress, ultimately making healthcare more effective.
Abstract
In this paper, we introduce Medical SAM 2 (MedSAM-2), an advanced segmentation model that utilizes the SAM 2 framework to address both 2D and 3D medical image segmentation tasks. By adopting the philosophy of taking medical images as videos, MedSAM-2 not only applies to 3D medical images but also unlocks new One-prompt Segmentation capability. That allows users to provide a prompt for just one or a specific image targeting an object, after which the model can autonomously segment the same type of object in all subsequent images, regardless of temporal relationships between the images. We evaluated MedSAM-2 across a variety of medical imaging modalities, including abdominal organs, optic discs, brain tumors, thyroid nodules, and skin lesions, comparing it against state-of-the-art models in both traditional and interactive segmentation settings. Our findings show that MedSAM-2 not only surpasses existing models in performance but also exhibits superior generalization across a range of medical image segmentation tasks. Our code will be released at: https://github.com/MedicineToken/Medical-SAM2