MedINST: Meta Dataset of Biomedical Instructions
Wenhan Han, Meng Fang, Zihan Zhang, Yu Yin, Zirui Song, Ling Chen, Mykola Pechenizkiy, Qingyu Chen
2024-10-24
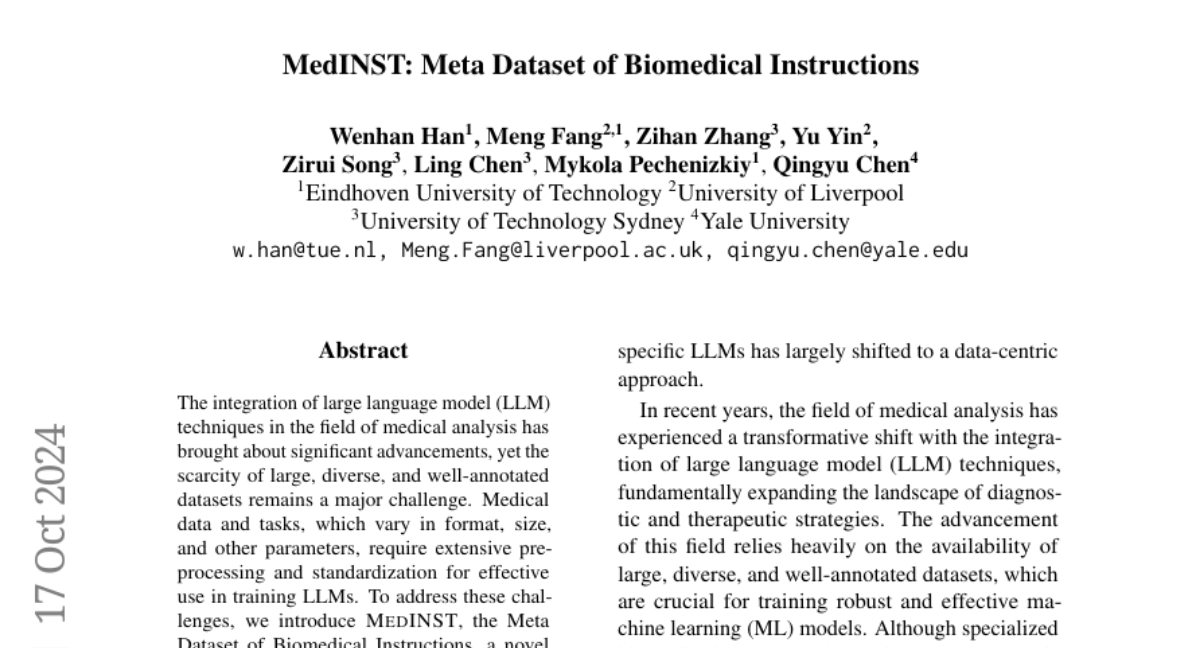
Summary
This paper introduces MedINST, a new dataset designed to improve the training of large language models (LLMs) for biomedical tasks by providing a wide variety of well-structured instructions.
What's the problem?
In the medical field, there is a lack of large and diverse datasets that can effectively train LLMs. Many existing datasets are not well-annotated or are limited in scope, making it difficult for these models to learn from the complex and varied nature of medical data.
What's the solution?
MedINST addresses this issue by creating a comprehensive meta-dataset that includes 133 different biomedical natural language processing (NLP) tasks and over 7 million training samples. This dataset allows for better training of LLMs across various medical tasks. Additionally, the authors developed a benchmark called MedINST32 to evaluate how well these models can generalize their knowledge across different tasks.
Why it matters?
This research is important because it provides a valuable resource for improving AI models in the medical field. By enhancing the training data available for LLMs, MedINST can lead to better performance in medical analysis, potentially improving patient care and outcomes through more accurate and efficient AI tools.
Abstract
The integration of large language model (LLM) techniques in the field of medical analysis has brought about significant advancements, yet the scarcity of large, diverse, and well-annotated datasets remains a major challenge. Medical data and tasks, which vary in format, size, and other parameters, require extensive preprocessing and standardization for effective use in training LLMs. To address these challenges, we introduce MedINST, the Meta Dataset of Biomedical Instructions, a novel multi-domain, multi-task instructional meta-dataset. MedINST comprises 133 biomedical NLP tasks and over 7 million training samples, making it the most comprehensive biomedical instruction dataset to date. Using MedINST as the meta dataset, we curate MedINST32, a challenging benchmark with different task difficulties aiming to evaluate LLMs' generalization ability. We fine-tune several LLMs on MedINST and evaluate on MedINST32, showcasing enhanced cross-task generalization.