MedXpertQA: Benchmarking Expert-Level Medical Reasoning and Understanding
Yuxin Zuo, Shang Qu, Yifei Li, Zhangren Chen, Xuekai Zhu, Ermo Hua, Kaiyan Zhang, Ning Ding, Bowen Zhou
2025-01-31
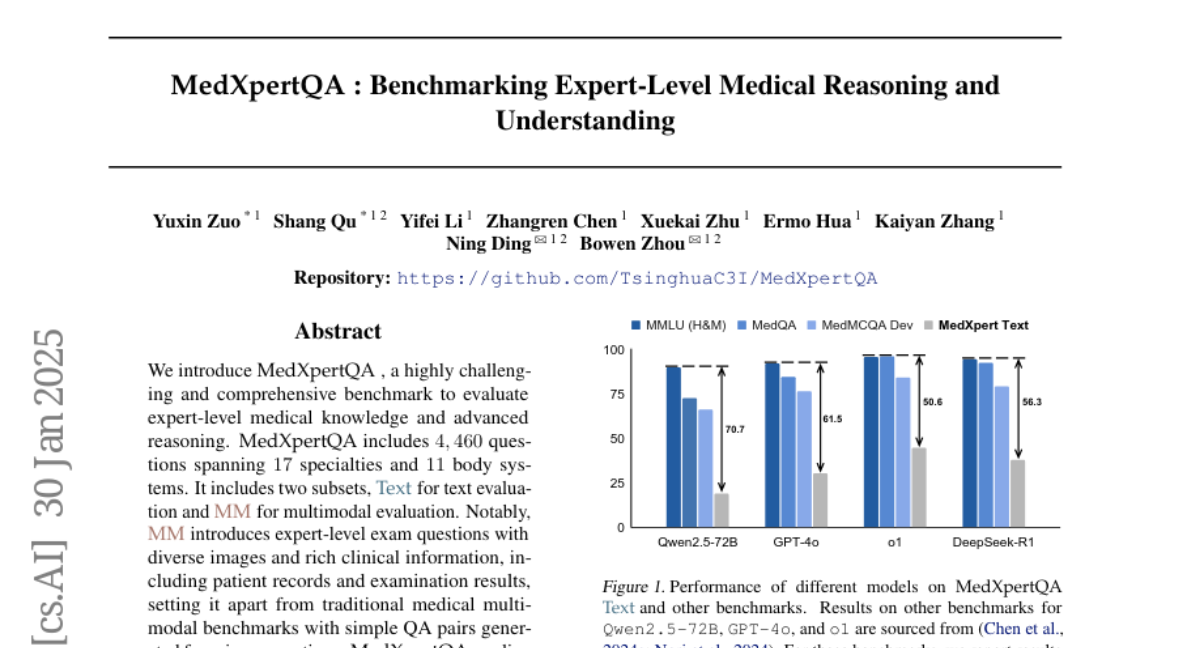
Summary
This paper talks about MedXpertQA, a new and challenging test designed to measure how well AI models understand and reason about complex medical knowledge. It's like creating a super hard medical exam that even the smartest AI has to work hard to pass.
What's the problem?
Current tests for AI in medicine aren't tough enough and don't cover all the important areas. They're often too simple, like asking basic questions about medical images, which doesn't really show if an AI can think like a real doctor. This makes it hard to know if AI is truly ready to help with real medical decisions.
What's the solution?
The researchers created MedXpertQA, which includes 4,460 really difficult questions covering 17 different medical specialties. They made two types of tests: one with just text and another that includes images and detailed patient information. They carefully chose and reviewed each question to make sure it's accurate and truly tests expert-level knowledge. They also made a special part of the test to see how well AI can reason through complex medical situations, not just memorize facts.
Why it matters?
This matters because as AI becomes more involved in healthcare, we need to be sure it's up to the task. MedXpertQA helps us understand if AI can really think like a doctor, which is crucial for patient safety and effective treatment. It also pushes AI developers to create smarter systems that can handle the complexity of real medical situations. By setting a high standard, this test could lead to better AI that doctors can trust to help them make important decisions about patient care.
Abstract
We introduce MedXpertQA, a highly challenging and comprehensive benchmark to evaluate expert-level medical knowledge and advanced reasoning. MedXpertQA includes 4,460 questions spanning 17 specialties and 11 body systems. It includes two subsets, Text for text evaluation and MM for multimodal evaluation. Notably, MM introduces expert-level exam questions with diverse images and rich clinical information, including patient records and examination results, setting it apart from traditional medical multimodal benchmarks with simple QA pairs generated from image captions. MedXpertQA applies rigorous filtering and augmentation to address the insufficient difficulty of existing benchmarks like MedQA, and incorporates specialty board questions to improve clinical relevance and comprehensiveness. We perform data synthesis to mitigate data leakage risk and conduct multiple rounds of expert reviews to ensure accuracy and reliability. We evaluate 16 leading models on MedXpertQA. Moreover, medicine is deeply connected to real-world decision-making, providing a rich and representative setting for assessing reasoning abilities beyond mathematics and code. To this end, we develop a reasoning-oriented subset to facilitate the assessment of o1-like models.