MegaFusion: Extend Diffusion Models towards Higher-resolution Image Generation without Further Tuning
Haoning Wu, Shaocheng Shen, Qiang Hu, Xiaoyun Zhang, Ya Zhang, Yanfeng Wang
2024-08-21
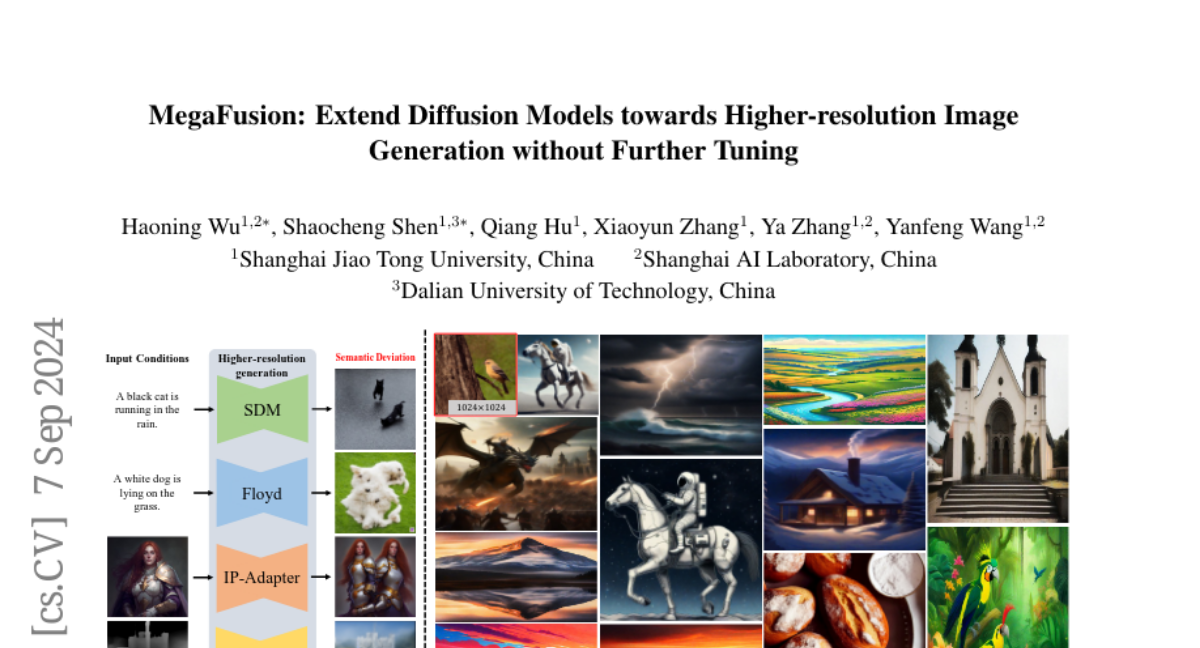
Summary
This paper introduces MegaFusion, a new method for generating high-resolution images using diffusion models without needing extra training.
What's the problem?
Diffusion models are great for generating images from text, but they usually have a fixed resolution during training. This can lead to problems when trying to create high-resolution images, such as inaccuracies in details and repeating objects.
What's the solution?
MegaFusion addresses these issues by using a technique called 'truncate and relay' to connect the image generation process across different resolutions. It allows the model to generate high-resolution images step by step, starting from a lower resolution and refining it. Additionally, it incorporates special techniques like dilated convolutions and noise re-scheduling to better handle higher resolutions. This method works well with various types of diffusion models and requires only about 40% of the original computational resources.
Why it matters?
This research is significant because it enables the creation of clearer and more detailed images from text prompts without the need for extensive additional training. This advancement can benefit many applications, including graphic design, video game development, and any field that relies on high-quality visual content.
Abstract
Diffusion models have emerged as frontrunners in text-to-image generation for their impressive capabilities. Nonetheless, their fixed image resolution during training often leads to challenges in high-resolution image generation, such as semantic inaccuracies and object replication. This paper introduces MegaFusion, a novel approach that extends existing diffusion-based text-to-image generation models towards efficient higher-resolution generation without additional fine-tuning or extra adaptation. Specifically, we employ an innovative truncate and relay strategy to bridge the denoising processes across different resolutions, allowing for high-resolution image generation in a coarse-to-fine manner. Moreover, by integrating dilated convolutions and noise re-scheduling, we further adapt the model's priors for higher resolution. The versatility and efficacy of MegaFusion make it universally applicable to both latent-space and pixel-space diffusion models, along with other derivative models. Extensive experiments confirm that MegaFusion significantly boosts the capability of existing models to produce images of megapixels and various aspect ratios, while only requiring about 40% of the original computational cost.