MegaPairs: Massive Data Synthesis For Universal Multimodal Retrieval
Junjie Zhou, Zheng Liu, Ze Liu, Shitao Xiao, Yueze Wang, Bo Zhao, Chen Jason Zhang, Defu Lian, Yongping Xiong
2024-12-20
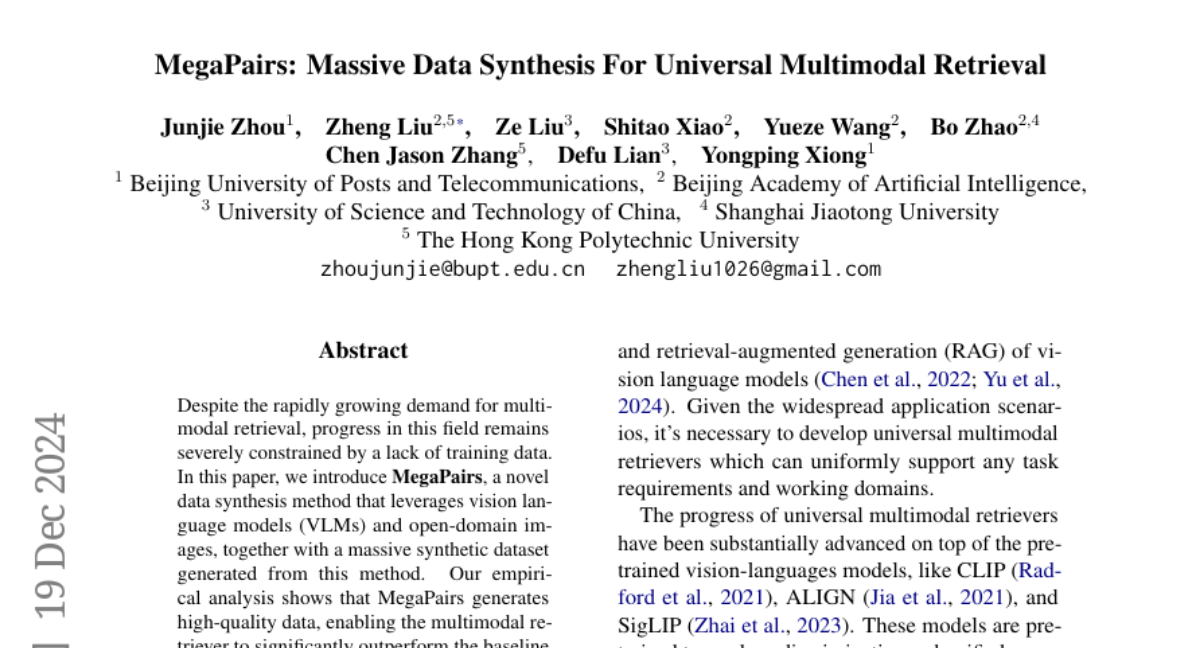
Summary
This paper introduces MegaPairs, a new method for creating large amounts of training data to improve multimodal retrieval systems, which are designed to understand and find information from both images and text.
What's the problem?
The field of multimodal retrieval is growing, but there isn't enough high-quality training data available. This lack of data makes it difficult for models to learn how to effectively connect and retrieve information from different types of content, like images and text.
What's the solution?
MegaPairs addresses this issue by synthesizing new training data using vision language models (VLMs) and open-domain images. The method generates over 26 million training examples that help models learn better. It uses a combination of different similarity models to create pairs of images and corresponding text descriptions, allowing the models to understand the relationships between them. This approach enables the models to perform well even with less initial data.
Why it matters?
This research is important because it significantly enhances the ability of multimodal retrieval systems to learn from diverse data sources. By providing a scalable way to generate high-quality training data, MegaPairs can help improve how AI understands and retrieves information from various media, which is essential for applications in search engines, digital assistants, and other AI-driven technologies.
Abstract
Despite the rapidly growing demand for multimodal retrieval, progress in this field remains severely constrained by a lack of training data. In this paper, we introduce MegaPairs, a novel data synthesis method that leverages vision language models (VLMs) and open-domain images, together with a massive synthetic dataset generated from this method. Our empirical analysis shows that MegaPairs generates high-quality data, enabling the multimodal retriever to significantly outperform the baseline model trained on 70times more data from existing datasets. Moreover, since MegaPairs solely relies on general image corpora and open-source VLMs, it can be easily scaled up, enabling continuous improvements in retrieval performance. In this stage, we produced more than 26 million training instances and trained several models of varying sizes using this data. These new models achieve state-of-the-art zero-shot performance across 4 popular composed image retrieval (CIR) benchmarks and the highest overall performance on the 36 datasets provided by MMEB. They also demonstrate notable performance improvements with additional downstream fine-tuning. Our produced dataset, well-trained models, and data synthesis pipeline will be made publicly available to facilitate the future development of this field.