Meissonic: Revitalizing Masked Generative Transformers for Efficient High-Resolution Text-to-Image Synthesis
Jinbin Bai, Tian Ye, Wei Chow, Enxin Song, Qing-Guo Chen, Xiangtai Li, Zhen Dong, Lei Zhu, Shuicheng Yan
2024-10-14
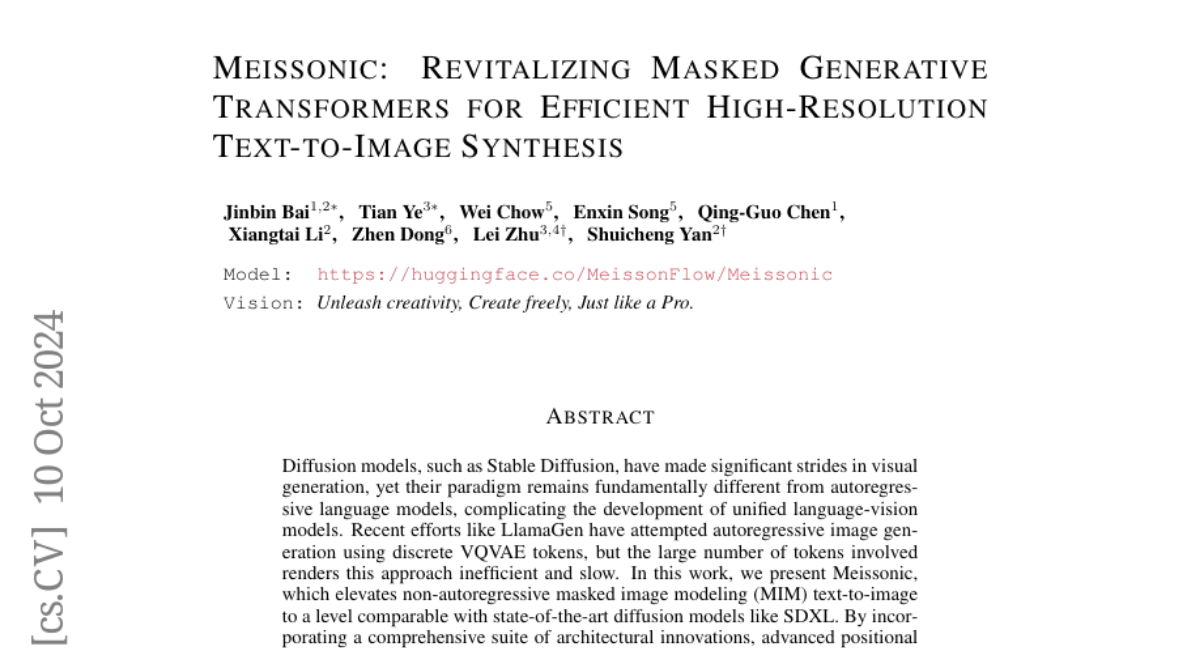
Summary
This paper introduces Meissonic, a new model designed for generating high-resolution images from text prompts using a non-autoregressive approach, which improves efficiency and quality compared to existing methods.
What's the problem?
Current models for generating images from text, like Stable Diffusion, are effective but can be slow and complicated due to their reliance on autoregressive methods. These methods require processing a lot of tokens, which can make the image generation process inefficient and time-consuming.
What's the solution?
Meissonic uses a technique called masked image modeling (MIM) that allows it to generate images more quickly and efficiently without needing to process each token in sequence. The researchers implemented several improvements, including better architectural designs, advanced encoding strategies, and optimized sampling conditions. They also used high-quality training data and incorporated human preferences to enhance the model's ability to produce clear and detailed images.
Why it matters?
This research is important because it sets a new standard for text-to-image synthesis by providing an open-source model that can generate high-quality images quickly. By improving the efficiency and performance of image generation, Meissonic has the potential to benefit various applications in art, design, and media where high-resolution images are needed.
Abstract
Diffusion models, such as Stable Diffusion, have made significant strides in visual generation, yet their paradigm remains fundamentally different from autoregressive language models, complicating the development of unified language-vision models. Recent efforts like LlamaGen have attempted autoregressive image generation using discrete VQVAE tokens, but the large number of tokens involved renders this approach inefficient and slow. In this work, we present Meissonic, which elevates non-autoregressive masked image modeling (MIM) text-to-image to a level comparable with state-of-the-art diffusion models like SDXL. By incorporating a comprehensive suite of architectural innovations, advanced positional encoding strategies, and optimized sampling conditions, Meissonic substantially improves MIM's performance and efficiency. Additionally, we leverage high-quality training data, integrate micro-conditions informed by human preference scores, and employ feature compression layers to further enhance image fidelity and resolution. Our model not only matches but often exceeds the performance of existing models like SDXL in generating high-quality, high-resolution images. Extensive experiments validate Meissonic's capabilities, demonstrating its potential as a new standard in text-to-image synthesis. We release a model checkpoint capable of producing 1024 times 1024 resolution images.