Meta Flow Matching: Integrating Vector Fields on the Wasserstein Manifold
Lazar Atanackovic, Xi Zhang, Brandon Amos, Mathieu Blanchette, Leo J. Lee, Yoshua Bengio, Alexander Tong, Kirill Neklyudov
2024-08-30
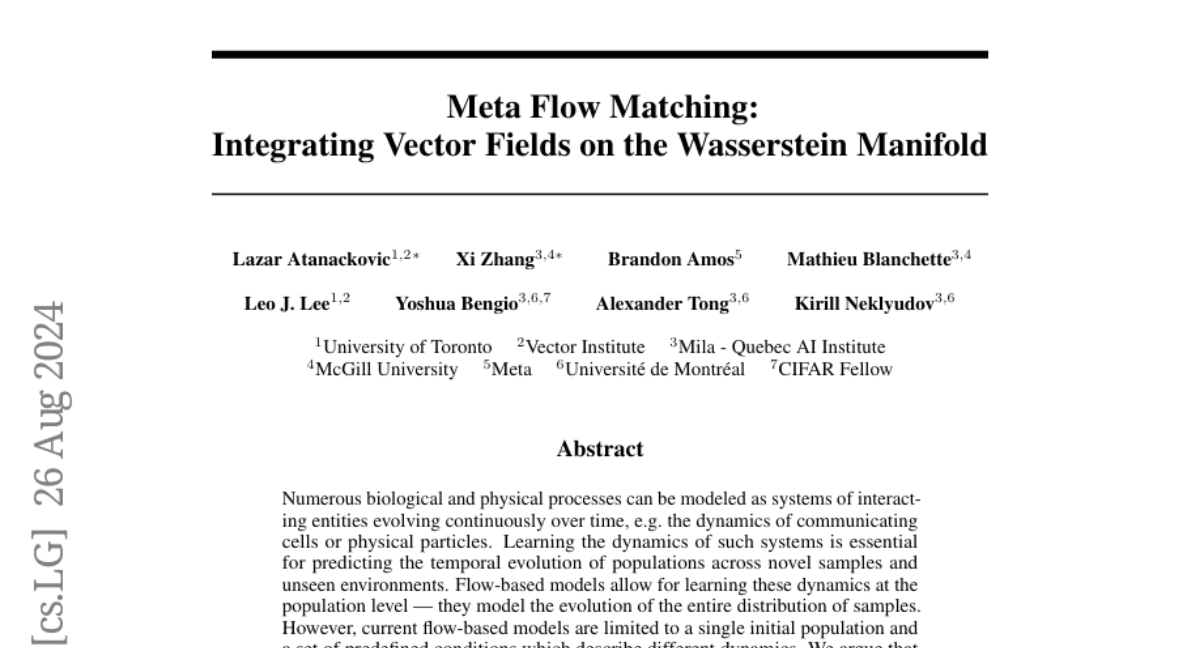
Summary
This paper introduces Meta Flow Matching (MFM), a new method for understanding how different biological and physical systems change over time using advanced mathematical techniques.
What's the problem?
Many natural processes, like how cells communicate or how particles move, need to be studied to predict their behavior in new situations. Current models often only look at one starting point and set conditions, which limits their ability to accurately represent the complex interactions that occur in real life.
What's the solution?
MFM addresses this issue by treating the changes in populations as vector fields on a special mathematical space called the Wasserstein manifold. This allows the model to consider how the population itself influences its changes over time. MFM uses a Graph Neural Network (GNN) to organize information about the population and then applies flow matching techniques to predict how these populations will evolve, even with different starting conditions.
Why it matters?
This research is significant because it can improve predictions in fields like personalized medicine, where understanding how diseases develop in individual patients is crucial. By providing a more accurate way to model complex systems, MFM can help scientists and doctors make better decisions based on predicted outcomes.
Abstract
Numerous biological and physical processes can be modeled as systems of interacting entities evolving continuously over time, e.g. the dynamics of communicating cells or physical particles. Learning the dynamics of such systems is essential for predicting the temporal evolution of populations across novel samples and unseen environments. Flow-based models allow for learning these dynamics at the population level - they model the evolution of the entire distribution of samples. However, current flow-based models are limited to a single initial population and a set of predefined conditions which describe different dynamics. We argue that multiple processes in natural sciences have to be represented as vector fields on the Wasserstein manifold of probability densities. That is, the change of the population at any moment in time depends on the population itself due to the interactions between samples. In particular, this is crucial for personalized medicine where the development of diseases and their respective treatment response depends on the microenvironment of cells specific to each patient. We propose Meta Flow Matching (MFM), a practical approach to integrating along these vector fields on the Wasserstein manifold by amortizing the flow model over the initial populations. Namely, we embed the population of samples using a Graph Neural Network (GNN) and use these embeddings to train a Flow Matching model. This gives MFM the ability to generalize over the initial distributions unlike previously proposed methods. We demonstrate the ability of MFM to improve prediction of individual treatment responses on a large scale multi-patient single-cell drug screen dataset.