Meta-Rewarding Language Models: Self-Improving Alignment with LLM-as-a-Meta-Judge
Tianhao Wu, Weizhe Yuan, Olga Golovneva, Jing Xu, Yuandong Tian, Jiantao Jiao, Jason Weston, Sainbayar Sukhbaatar
2024-07-30
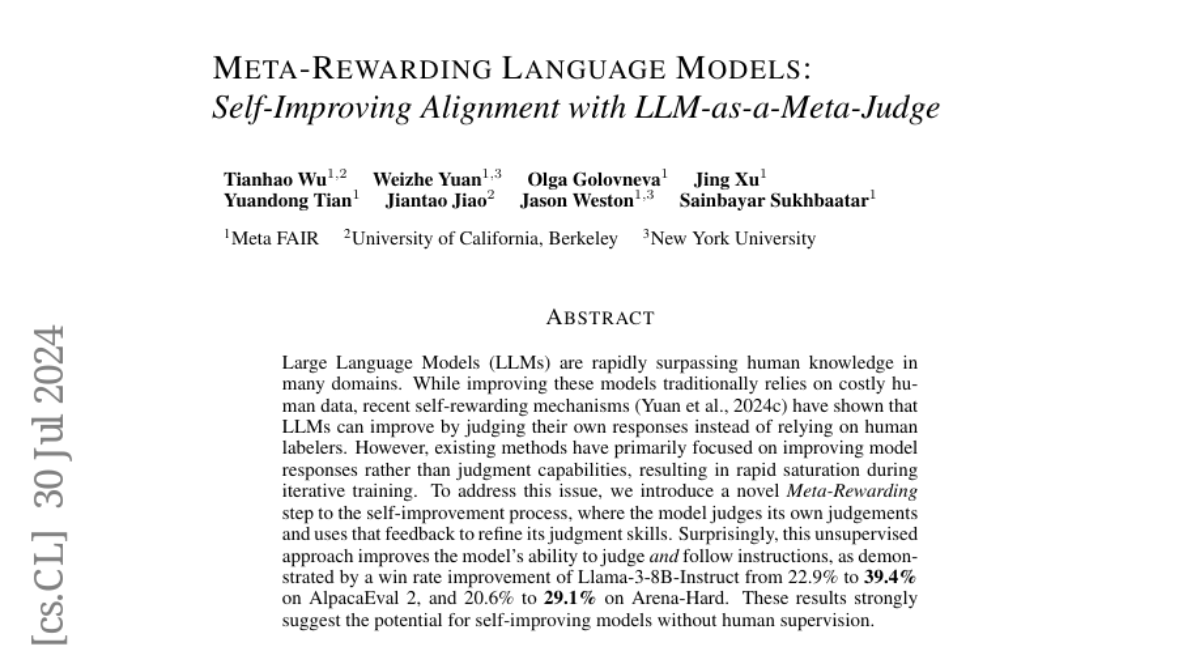
Summary
This paper introduces a new approach called Meta-Rewarding, which helps large language models (LLMs) improve their ability to judge their own responses. By using a model as a 'meta-judge,' it can refine its judgment skills and enhance its overall performance without needing human feedback.
What's the problem?
Traditionally, improving LLMs requires a lot of expensive human-generated data. While some recent methods allow models to learn by judging their own answers, they mainly focus on improving the responses rather than the judgment process itself. This can lead to quick saturation, where the model stops getting better over time because it doesn't improve its ability to evaluate its own outputs.
What's the solution?
To tackle this issue, the authors propose the Meta-Rewarding method, where the model not only generates responses but also judges those responses and then judges its own judgments. This self-evaluating process helps the model learn to improve both its responses and its judging capabilities. The authors tested this approach on a specific model called Llama-3-8B-Instruct and found significant improvements in its performance on various benchmarks, showing that this method can lead to better instruction-following abilities.
Why it matters?
This research is important because it suggests a way for AI models to become more capable and aligned with human values without relying heavily on human input. By enabling models to self-improve through their own evaluations, it could make them more effective in various applications, leading to smarter and more reliable AI systems.
Abstract
Large Language Models (LLMs) are rapidly surpassing human knowledge in many domains. While improving these models traditionally relies on costly human data, recent self-rewarding mechanisms (Yuan et al., 2024) have shown that LLMs can improve by judging their own responses instead of relying on human labelers. However, existing methods have primarily focused on improving model responses rather than judgment capabilities, resulting in rapid saturation during iterative training. To address this issue, we introduce a novel Meta-Rewarding step to the self-improvement process, where the model judges its own judgements and uses that feedback to refine its judgment skills. Surprisingly, this unsupervised approach improves the model's ability to judge {\em and} follow instructions, as demonstrated by a win rate improvement of Llama-3-8B-Instruct from 22.9% to 39.4% on AlpacaEval 2, and 20.6% to 29.1% on Arena-Hard. These results strongly suggest the potential for self-improving models without human supervision.